|
|
|
|
|
|
The latest agritech news from AZoLifeSciences |
|
|
|
|
Innovations in Fertilizer Management: Plasma Treatments & Soil Gas Flux Insights
Can innovations in fertilizer management help combat climate change?
Hear from a leading soil scientist about how understanding N₂O and CH₄ fluxes is driving new practices to reduce greenhouse gas emissions in agriculture. Join the webinar and discover the groundbreaking technologies driving the research into reducing agricultural greenhouse gas emissions.
| |
|
|
| | 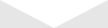 | | The effects of climate change on marine biology are profound, impacting species distribution, ecosystem health, and the services oceans provide to humanity. | | | | Insect pests can destroy crops if they are not controlled. Crops that produce bacterial proteins that kill important pests without endangering humans or wildlife have been genetically modified to limit damage and lessen the need for insecticide sprays. | | | | Key gene regulators that allow certain deafened animals, such as fish and lizards, to naturally regenerate their hearing have been identified by a recent USC Stem Cell study that was published in the journal Proceedings of the National Academy of Sciences (PNAS). | | | | Innovative approaches to soil erosion, including biopolymers and geotextiles, are vital for enhancing soil health and supporting sustainable agriculture. | | | | Advancements in agricultural science are transforming farming, emphasizing sustainability, precision agriculture, and innovative practices to boost crop yields. | | | | Researchers used AI-powered analysis of 52 UK lakes to identify insecticides, fungicides, and heavy metals as top drivers of freshwater biodiversity loss, revealing widespread harm to non-target species and actionable targets for conservation. | | | | Invasive plants are doing more than just taking over landscapes -; they're also changing the soil beneath them. | |
|
|
| | How would you rate today's newsletter?
| |
|
|
| |
 |
Stay updated with the latest in health and medical news! Follow News‑Medical.net on Google News for real‑time updates. Click here to follow us now. |
| |
|
|
|
|
|
|