This article and associated images are based on a poster originally authored by Thomas Pesnot, Sandeep Pal, Zandile Nare, Vincenzo A. Rao, Ian Morrison, Andrew Scott, Matilda J. Bingham, Muhammad Jan, Brian O. Smith and Edward A. Fitzgerald and presented at ELRIG Drug Discovery 2024 in affiliation with Concept Life Sciences Ltd, University of Glasgow and Malvern Panalytical.
This poster is being hosted on this website in its raw form, without modifications. It has not undergone peer review but has been reviewed to meet AZoNetwork's editorial quality standards. The information contained is for informational purposes only and should not be considered validated by independent peer assessment.
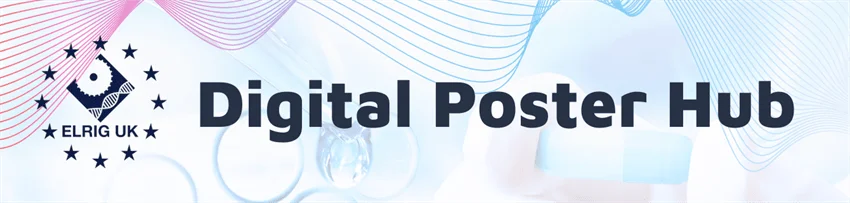
Shifting the Hit Identification Paradigm
In this study, a team of researchers developed BioPALS, an innovative, lean, and versatile hit identification platform combining AI-powdered virtual screening with cutting-edge biophysical technologies.
BioPALS enables the rapid identification and comprehensive characterization of hits. The implementation of this technology is exemplified in this study using the BRPF1b bromodomain for which BioPALS identified a range of potent, selective, and lead-like hits, thus offering new opportunities for the treatment of AML and many other cancers.
BRPF1b—An Actionable Target
Bromodomains are epigenetic proteins that recognize acetylated histone tails. Their activity can lead to chromatin remodeling and alter the expression of genes, such as those driving cancer, inflammation, and neurological disorders.1
Bromodomain and PHD finger-containing protein 1b (BRPF1b) modulate gene transcription via multiple chromatin reader domains, including a double PHD and zinc finger assembly, a bromodomain, and a C-terminal PWWP domain. BRPF1b has been hailed as an attractive therapeutic target to treat HCC and< AML, an aggressive cancer with a 5-year survival rate below 30 % for adults.2,3,4
A range of BRPF1b bromodomain inhibitors have been reported in recent years, including GSK6853 and NI-57 (Figure 1). In silico methodologies, such as high-throughput fragment docking and ligand-based screening, have been employed to identify additional chemotypes, and their optimization has been aided by X-ray crystallography.5,6,7,8
Despite these efforts, a selective BRPF1b inhibitor has yet to enter clinical investigation, and new chemotypes inhibiting this target are still highly sought after.
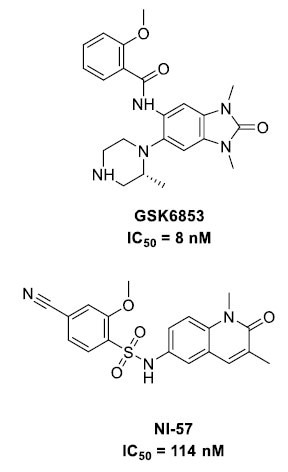
Figure 1. BRPF1b inhibitors. Image Credit: Concept Life Sciences
The BioPALS Workflow
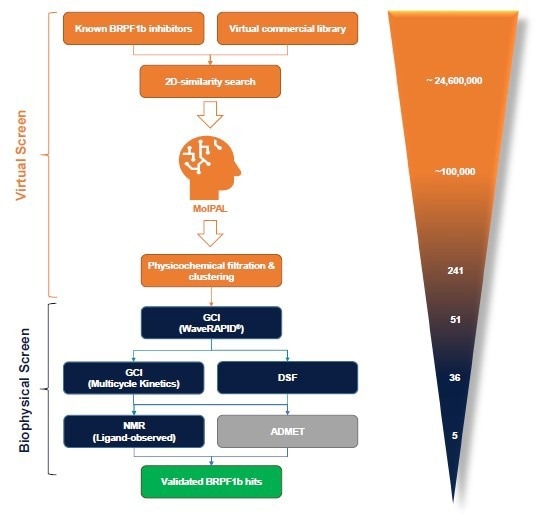
Figure 2. The BioPALS hit identification process is centered on the MolPAL algorithm and a GCI-driven biophysical hit confirmation workflow. In silico activities are displayed in orange, biophysical screening activities are displayed in blue, and ADMET profiling is displayed in grey. The number of compounds processed through each individual stage for the BRPF1b bromodomain is shown on the right. Image Credit: Concept Life Sciences
1. AI-Driven Virtual Screening—MolPAL
The MolPAL structure-based virtual screening algorithm predicts docking scores using simple molecular fingerprints. Despite docking only a small fraction (typically around 1 %) of the entire compound collection, MolPAL efficiently identifies the highest-scoring compounds.
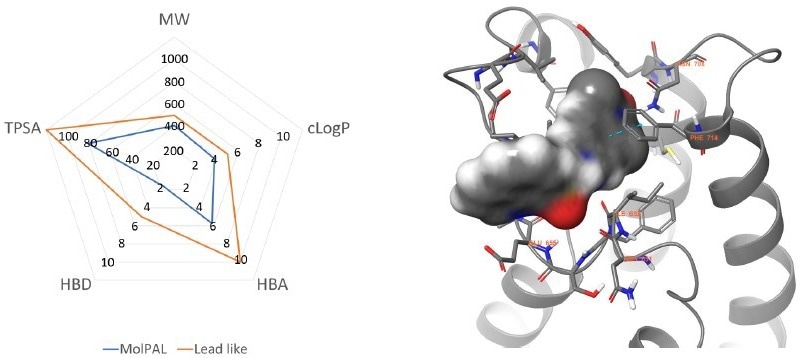
Figure 3. Left: Physicochemical profile of the MolPAL output (blue) compared to lead-like boundaries (orange). Right: The lowest energy docking pose was predicted by MolPAL for compound 5 (grey surface) within the BRPF1b active site (grey cartoon, PDB = 6EQK). Key residues are represented in grey sticks and amino acid sequence numbers with orange labels. Nitrogen, Oxygen, and Sulfur atoms are colored blue, red, and yellow, respectively. π- π interactions are represented with dotted cyan lines. Image Credit: Concept Life Sciences
After an initial 2D similarity search across 24.6 million commercial compounds, approximately 100,000 compounds were analyzed with MolPAL over four cycles using our cloud computing platform. A subsequent chemoinformatic triage of the top virtual hits yielded 51 diverse, lead-like candidates with predicted binding topologies from MolPAL (Figure 3).
2. Primary in vitro Confirmation—GCI
Grating-coupled interferometry (GCI) is a label-free biosensing technique used to provide excellent resolution of extremely rapid binding kinetics. WaveRAPID® (Repeated Analyte Pulses of Increasing Duration) injects a single concentration, pulsing the sample over the sensing surface at increasing durations.10
A waveRAPID® GCI screening of the 51 virtual hits (100 μM) highlighted 36 primary hit binders (Figure 4). Multicycle kinetics confirmed 20 compounds bind BRPF1b with KD < 250 μM (39 % hit rate). Binding kinetics were determined for all hits, GSK6853 and NI-57 (Table 1).
A BRPF1a counter-screen did not identify any binder, demonstrating the identified hits are selective for BRPF1b over BRPF1a.
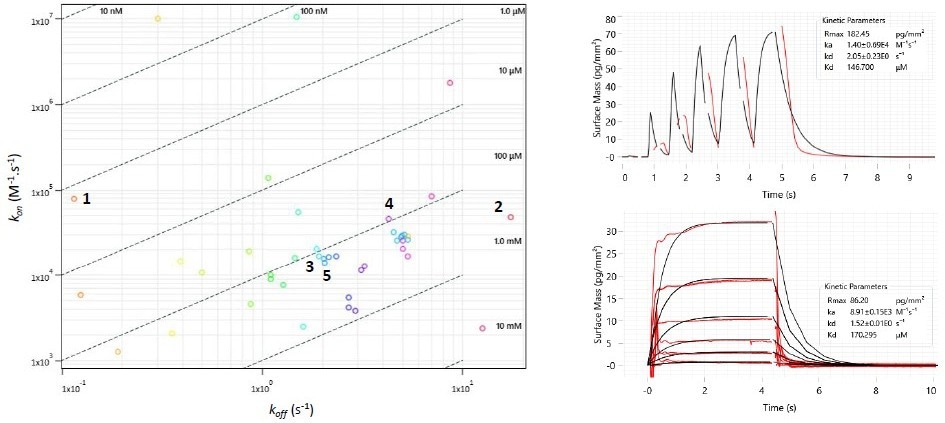
Figure 4. Left: Two-dimensional isoaffinity kinetic plot of association (kon) and dissociation (koff) rate constants from waveRAPID® screen of 51 virtual hits. The 36 primary hit binders are shown. Diagonal lines indicate equilibrium binding constants (KD). Each circle represents a binder and is colored according to the dissociation rate. Top right: WaveRAPID® GCI sensorgram of compound 5 (100 μM) binding to BRPF1b. Bottom right: Multicycle kinetics GCI sensorgram of 5 binding to BRPF1b. Image Credit: Concept Life Sciences
3. Orthogonal Hit Confirmation—DSF
The 36 waveRAPID® hits were validated orthogonally through differential scanning fluorimetry (DSF) at 100 μM. Thirteen compounds produced a BRPF1b thermal shift (ΔTm) of more than ±4 °C, indicating a substantial interaction with the protein (Figure 5). Four of these hits induced a thermal shift equal to or greater than that of GSK6853 and NI-57.
Eight hits displaying a KD < 250 μM and a ΔTm > ±4 °C were identified, including compounds 1, 2, 3, 4, and 5 (Table 1). This provided a prioritized list of BRPF1b hits, which were confirmed in vitro using a combination of orthogonal techniques (GCI and DSF).
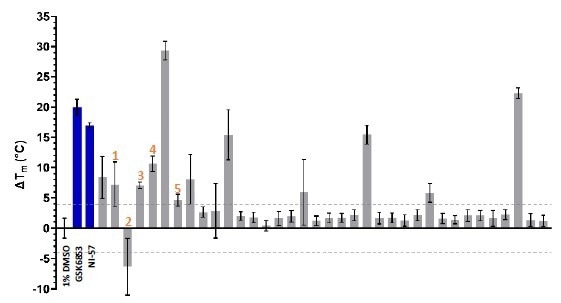
Figure 5. DSF characterization of the 36 waveRAPID® primary hits (grey). GSK6853 and NI-57 are colored in blue, and the > +4 °C and < -4 °C thresholds are indicated with grey dashed lines. Image Credit: Concept Life Sciences
4. Hit Binding Topology—Ligand-Observed NMR
Binding was further confirmed for 4 out of the 5 prioritized hits using a range of ligand-observed NMR acquisition methods, including 1D,11 STD,12 waterLOGSY13, and CPMG14 in the presence and absence of BRPF1b protein. Hit binding topologies could be inferred for some of the ligands based on specific shift changes upon the addition of protein (Figure 6), supporting the docking poses predicted by MolPAL.
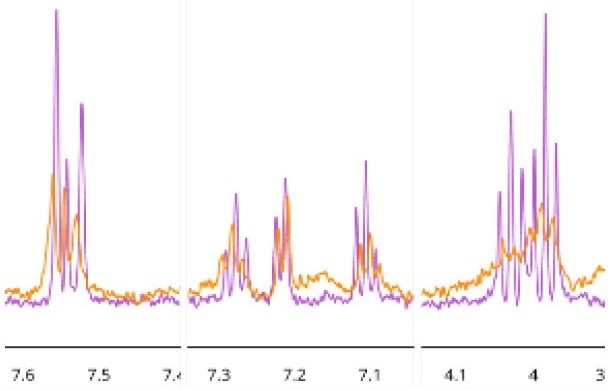
Figure 6. Sections of the 1D 1H NMR spectra illustrating peaks from compound 5 (400 μM) in the absence (pink) and presence (orange) of the BRPF1b Virtual commercial library bromodomain (26 μM). Image Credit: Concept Life Sciences
5. Selecting the Best Hits—Biophysics and ADMET

Image Credit: ELRIG (UK) Ltd.
Table 1. Summary of biophysical data for GSK6853, NI-57 and five BRPF1b hits. cLogDs were determined using QikProp. KD, kon and koff were determined by GCI multicycle kinetics (N=2). ΔTm was determined by DSF (N=2). Ligand-observed NMR binding classification results from a consensus using 1D, STD, waterLOGSY and CPMG acquisition methods. n.d. = not determined. Source: Concept Life Sciences
Entry |
MW |
cLogD |
KD |
kon |
koff |
ΔTm |
NMR binding |
unit |
g.mol-1 |
- |
μM |
M.s-1 |
s-1 |
°C |
- |
GSK6853 |
409 |
2.9 |
0.078 |
1.3 x 106 |
0.01 |
+ 19.9 |
n.d. |
NI-57 |
383 |
1.5 |
0.086 |
1.9 x 107 |
0.16 |
+ 17.0 |
n.d. |
1 |
448 |
3.9 |
44 |
4.6 x 104 |
2.0 |
+ 7.2 |
Yes |
2 |
431 |
2.8 |
46 |
4.8 x 104 |
2.2 |
- 6.3 |
Yes |
3 |
367 |
2.1 |
61 |
3.5 x 104 |
2.0 |
+ 7.1 |
Insoluble |
4 |
369 |
2.9 |
128 |
3.0 x 104 |
2.1 |
+ 10.6 |
Yes |
5 |
354 |
2.1 |
186 |
8.4 x 103 |
1.6 |
+ 4.6 |
Yes |
Table 2. In vitro ADMET profile of five BRPF1b hits. CYP450 inhibition was measured as a pooled mixture of 1A2, 2C9 2C19, 2D6 and 3A4 isoforms. MPPB = mouse plasma protein binding, MLM = mouse liver microsomal stability, RLM = rat liver microsomal stability, HLM = human liver microsomal stability, HHeps = human hepatocyte stability, Fu = fraction unbound, n.d. = not determined. Source: Concept Life Sciences
Entry |
MPPB
(Fu) |
MLM
(Clint) |
RLM
(Clint) |
HLM
(Clint) |
HHeps
(Clint) |
CYP450 inhib.
(IC50) |
unit |
- |
μL.min-1.mg-1 |
μL.min-1.mg-1 |
μL.min-1.mg-1 |
μL.min-1.106 cells |
μM |
1 |
0.18 |
17 |
41 |
< 5 |
< 3 |
All > 50 |
2 |
0.12 |
< 5 |
< 5 |
< 5 |
< 3 |
All > 10 |
3 |
0.23 |
20 |
89 |
10 |
4 |
n.d. |
4 |
0.12 |
6 |
< 5 |
< 5 |
< 3 |
n.d. |
5 |
0.05 |
157 |
216 |
94 |
67 |
n.d. |
References
- You, L., et al. (2015). Deficiency of the Chromatin Regulator Brpf1 Causes Abnormal Brain Development. Journal of Biological Chemistry, 290(11), pp.7114–7129. https://doi.org/10.1074/jbc.m114.635250.
- Cheng, C.L.-H., et al. (2021). Bromodomain-containing protein BRPF1 is a therapeutic target for liver cancer. Communications Biology, 4(1). https://doi.org/10.1038/s42003-021-02405-6.
- Shima, H., et al. (2013). Bromodomain-PHD finger protein 1 is critical for leukemogenesis associated with MOZ–TIF2 fusion. International Journal of Hematology, [online] 99(1), pp.21–31. https://doi.org/10.1007/s12185-013-1466-x.
- Cancer Research UK (2016). Acute myeloid leukaemia (AML) | Cancer Research UK. [online] Cancerresearchuk.org. Available at: https://www.cancerresearchuk.org/about-cancer/acute-myeloid-leukaemia-aml.
- Bamborough, P., et al. (2016). GSK6853, a Chemical Probe for Inhibition of the BRPF1 Bromodomain. ACS Medicinal Chemistry Letters, [online] 7(6), pp.552–557. https://doi.org/10.1021/acsmedchemlett.6b00092.
- Igoe, N., et al. (2017). Design of a Chemical Probe for the Bromodomain and Plant Homeodomain Finger-Containing (BRPF) Family of Proteins. Journal of Medicinal Chemistry, 60(16), pp.6998–7011. https://doi.org/10.1021/acs.jmedchem.7b00611.
- Zhu, J. and Caflisch, A. (2016). Twenty Crystal Structures of Bromodomain and PHD Finger Containing Protein 1 (BRPF1)/Ligand Complexes Reveal Conserved Binding Motifs and Rare Interactions. Journal of Medicinal Chemistry, 59(11), pp.5555–5561. https://doi.org/10.1021/acs.jmedchem.6b00215.
- Zhu, J., Zhou, C. and Caflisch, A. (2018). Structure-based discovery of selective BRPF1 bromodomain inhibitors. European Journal of Medicinal Chemistry, 155, pp.337–352. https://doi.org/10.1016/j.ejmech.2018.05.037.
- Graff, D.E., Shakhnovich, E.I. and Coley, C.W. (2021). Accelerating high-throughput virtual screening through molecular pool-based active learning. Chemical Science, 12(22), pp.7866–7881. https://doi.org/10.1039/d0sc06805e.
- Kartal, Ö., et al. (2021). waveRAPID—A Robust Assay for High-Throughput Kinetic Screens with the Creoptix WAVEsystem. SLAS DISCOVERY: Advancing the Science of Drug Discovery, 26(8), pp.995–1003. https://doi.org/10.1177/24725552211013827.
- Hajduk, P.J., Olejniczak, E.T. and Fesik, S.W. (1997). One-Dimensional Relaxation- and Diffusion-Edited NMR Methods for Screening Compounds That Bind to Macromolecules. Journal of the American Chemical Society, 119(50), pp.12257–12261. https://doi.org/10.1021/ja9715962.
- Mayer, M. and Meyer, B. (1999). Characterization of Ligand Binding by Saturation Transfer Difference NMR Spectroscopy. Angewandte Chemie International Edition, 38(12), pp.1784–1788. https://doi.org/10.1002/(sici)1521-3773(19990614)38:12%3C1784::aid-anie1784%3E3.0.co;2-q.
- Dalvit, C., et al. (2001). WaterLOGSY as a method for primary NMR screening: practical aspects and range of applicability. Journal of Biomolecular NMR, 21(4), 349–359. https://doi.org/10.1023/a:1013302231549.
- Larsen, F.H., Jakobsen, H.J., Ellis, P.D. and Nielsen, N.Chr. (1997). Sensitivity-Enhanced Quadrupolar-Echo NMR of Half-Integer Quadrupolar Nuclei. Magnitudes and Relative Orientation of Chemical Shielding and Quadrupolar Coupling Tensors. The Journal of Physical Chemistry A, 101(46), pp.8597–8606. https://doi.org/10.1021/jp971547b.
About Concept Life Sciences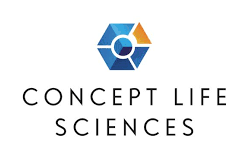
Concept Life Sciences is a knowledge-based, science-led and customer-focused contract research and manufacturing organization with world-leading expertise in the pharmaceutical, biotech and agrochemical industries.
We are based across 5 sites in the UK delivering discovery research as well as GMP, GLP and GCP-regulated work. Whether it is delivering whole programs of research or bespoke studies we deliver a collaborative client-centered approach. Our services are built on scientist-to-scientist engagement, deep knowledge, flexibility and extensive in-house resources.
About ELRIG (UK) Ltd.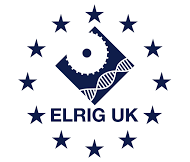
The European Laboratory Research & Innovation Group (ELRIG) is a leading European not-for-profit organization that exists to provide outstanding scientific content to the life science community. The foundation of the organization is based on the use and application of automation, robotics and instrumentation in life science laboratories, but over time, we have evolved to respond to the needs of biopharma by developing scientific programmes that focus on cutting-edge research areas that have the potential to revolutionize drug discovery.
Comprised of a global community of over 12,000 life science professionals, participating in our events, whether it be at one of our scientific conferences or one of our networking meetings, will enable any of our community to exchange information, within disciplines and across academic and biopharmaceutical organizations, on an open access basis, as all our events are free-of-charge to attend!
Our values
Our values are to always ensure the highest quality of content and that content will be made readily accessible to all, and that we will always be an inclusive organization, serving a diverse scientific network. In addition, ELRIG will always be a volunteer led organization, run by and for the life sciences community, on a not-for-profit basis.
Our purpose
ELRIG is a company whose purpose is to bring the life science and drug discovery communities together to learn, share, connect, innovate and collaborate, on an open access basis. We achieve this through the provision of world class conferences, networking events, webinars and digital content.
Sponsored Content Policy: AZoLifeSciences publishes articles and related content that may be derived from sources where we have existing commercial relationships, provided such content adds value to the core editorial ethos of AZoLifeSciences which is to educate and inform site visitors interested in life science news and information.