Smart criminal investigation requires accurate forensic examination. To determine a single fingerprint, many suspected fingerprint data must be analyzed. This could be an extremely time-consuming and tedious task if performed manually. Automation has helped forensic science experts to tackle the exponential growth in cases, to solve crimes, and prevent them.
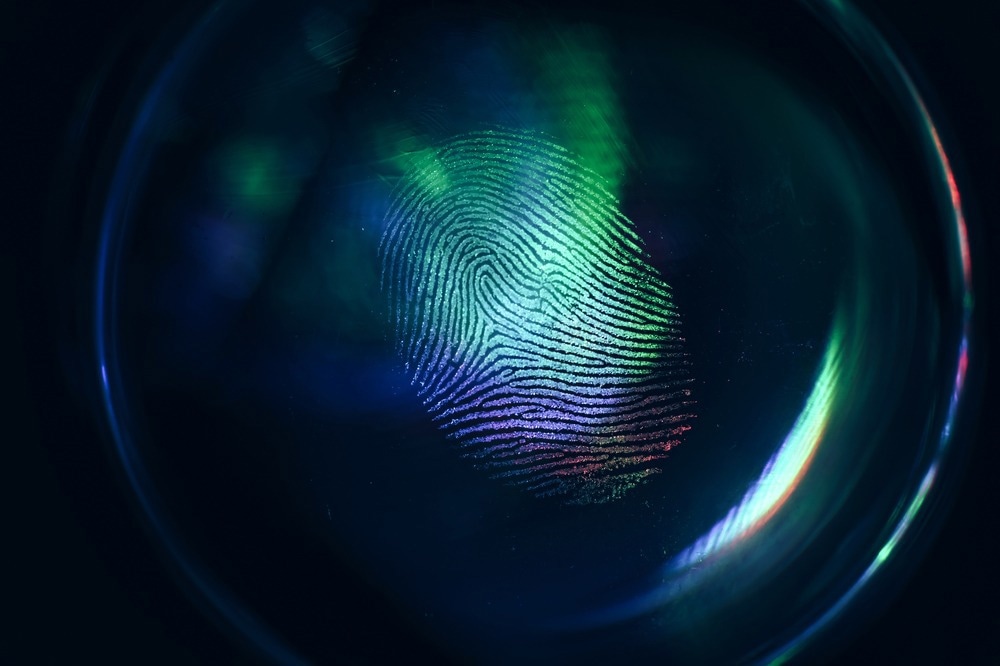
Image Credit: Bushko Oleksandr/Shutterstock.com
Technologies to Advance Automation in Forensic Science
Artificial Intelligence (AI), Machine Learning (ML), and Deep Learning (DL) methods have been used to advance automation in forensic science to solve crimes. AI is a branch of computer science associated with developing intelligent machines or computers that work like humans. ML and DL function similarly and can be grouped under AI at large.
ML is a part of AI that allows a computer program or machine to learn from previous data or patterns. ML-based computer programs or machines utilize these data to predict new output values via specific algorithms. DL is a subset of ML whose algorithms, known as neural networks, can sometimes mimic the human brain. Put simply; ML is the ability of computers to learn without specific programming, while DL enables computers or machines to perform unsupervised learning.
Artificial neural networks are based on computational, mathematical, or technological models that mimic human logic and the functions of the brain’s neurons. AI-based machines are able to engage in human-like processes, such as speech recognition, adaptation, learning, self-correction, reasoning, visual perception, cognitive thinking, decision-making, and rational acting.
AI-based machines can learn from experience and solve complex problems faster and more accurately than humans. AI technology is improving rapidly; for instance, AI-based programs that are known as intelligent agents are used to interact with the environment through sensors and procure important data.
Automation in Forensic Science Using AI
AI has proved to be highly beneficial for varied aspects of forensic science, such as image recognition, image and video analysis, firearm identification, gunshot detection, 3D crime scene reconstruction, handwriting identification, and large-scale data analysis. Furthermore, this technology has enabled the determination of time since death, age, and personal identifications through dental examination data, sex determination of skeletal remains, 3D facial reconstruction from an unidentified skull, and cybercrimes and digital evidence detection.
The advancements in AI technologies have helped not only police and security professionals in crime detection but also crime prevention and prediction. Highly effective AI algorithms have recently been developed to uncover criminal networks and detect crime patterns and suspicious anomalies. AI enables pattern recognition of crime site evidence, such as lip prints, bitemarks, shoe prints, and bullet marks. Pattern identification is a function of automatic machine recognition based on the type of learning procedure.
Automated AI systems can narrow down the suspects of a crime based on data analysis with high accuracy. Among various AI algorithms for automatic human face recognition, neural networks have proved to be the most efficient. Neural networks have been implemented to interpret visual images, face recognition, iris recognition, fingerprint recognition, and speech recognition.
The AI neuroprediction technique combines neuroimaging, AI tools linked to functional brain parameters, and ML methods for clinical or behavioral predictions. This technique has been extensively used in forensic psychiatry. Artificial neural networks can assess whether an individual is purposely behaving dumb or truly is.
The functions of biochemistry, AI, and aroma-sensor technology have been combined to develop devices or machines that can measure and characterize volatile aromas. These devices are known as electronic noses (e-nose). Criminal investigators use e-noses and chemical and biological weapons to detect explosive materials.
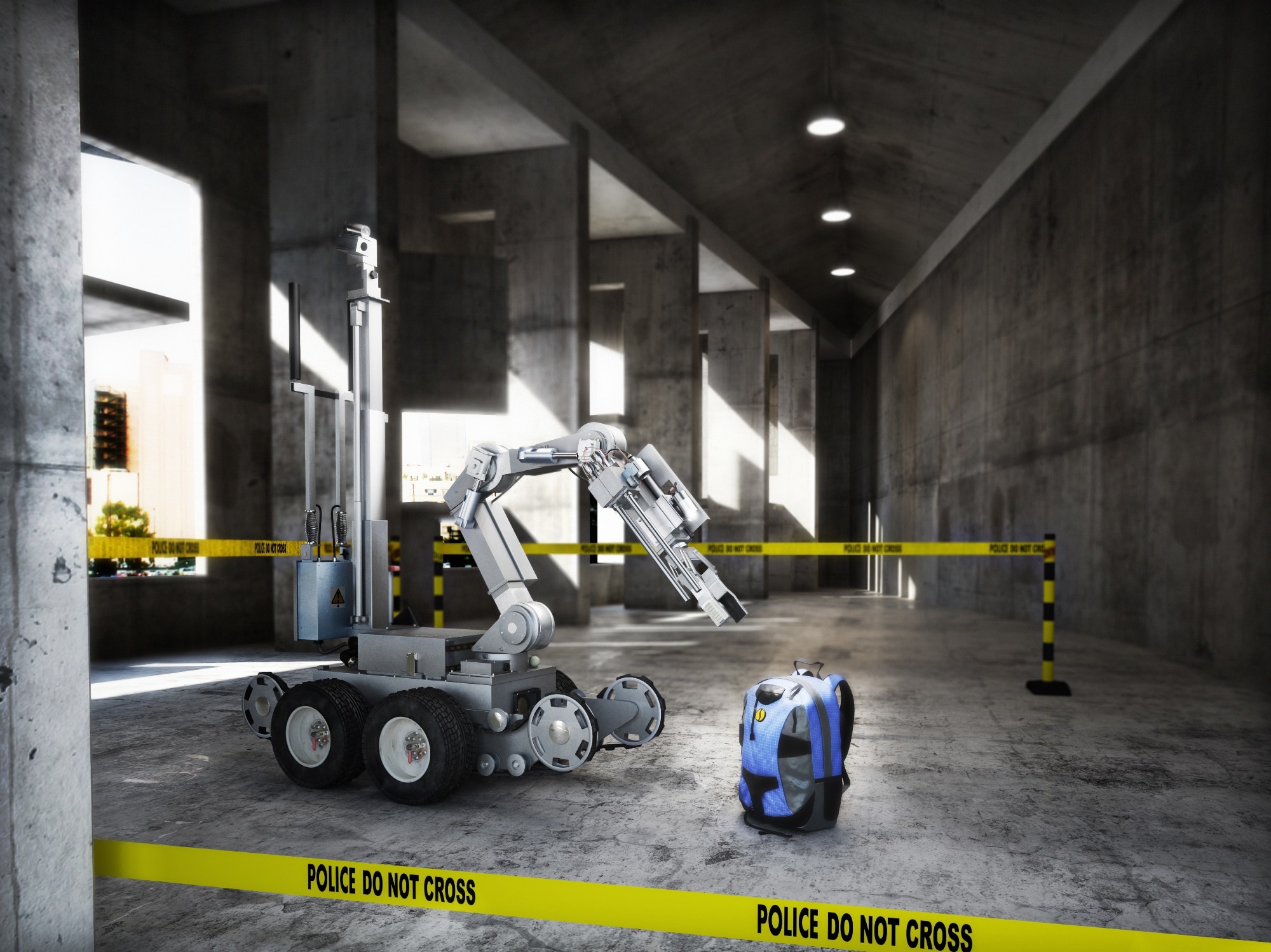
Image Credit: Digital Storm/Shutterstock.com
Importance of Automation in Forensic Science
Automation has been applied in Forensic DNA laboratories to establish DNA Databases, which has significantly reduced the processing time of crime evidence samples. Automation in digital forensics, associated with intelligent analyzing techniques for solving complex problems based on huge datasets, has proved extremely useful.
Forensic science automation allows the examination and comparison of large data volumes, such as fingerprints, handwriting, videos, images, and audio, accurately and in less time. An intelligent automation agent is composed of an AI-powered system to compare, analyze, interpret, and correlate data obtained during a criminal investigation.
Automation also helps efficient utilization of resources in forensic labs to alleviate backlogs. Implementation of automation uncovers artifacts that also allow criminal investigators to heed lower priority cases. Experts often need to evaluate large data volumes, such as chat or web history, during an investigation. They might repeatedly encounter similar scripts, which could be avoided using software such as Volatility.
Forensic experts can use automation for parallel processing, allowing them to analyze multiple pieces of evidence simultaneously. After automation completes the routine processes quickly, examiners can directly analyze the processed data. Automation in forensic science does not eliminate the need for experts but allows examiners to dedicate more time to analyzing data and ultimately solving the crime.
Sources:
- Jarrett, A, and Choo, K-K.R. (2021) The impact of automation and artificial intelligence on digital forensics. WIREs Forensic Science. 3(6). https://doi.org/10.1002/wfs2.1418
- Jadhav, B.K. et al. (2020) Artificial Intelligence: Advancing Automation in Forensic Science & Criminal Investigation. Journal of Seybold Report. 15(8).
- Why Automation in Digital Forensics? (2020) [Online] Available at: https://www.magnetforensics.com/blog/why-automation-in-digital-forensics/
- Iqbal, S. and Abed Alharbi, S. (2020) Advancing Automation in Digital Forensic Investigations Using Machine Learning Forensics. IntechOpen. doi: 10.5772/intechopen.90233
- Greyling, J.A. (2013) Forensic DNA laboratory automation – Principles and guidelines. Forensic Science International: Genetics Supplement Series. 4(1), pp. e135-e136. https://doi.org/10.1016/j.fsigss.2013.10.069
- Stallard, T. and Levitt, K. (2003) Automated analysis for digital forensic science: semantic integrity checking. 19th Annual Computer Security Applications Conference, 2003. Proceedings., Las Vegas, NV, USA, pp. 160-167. doi: 10.1109/CSAC.2003.1254321.
Further Reading