From the original rolled ink method to the new use of 3D contactless imaging and deep learning, fingerprinting technology has progressed rapidly over the years. Nonetheless, many challenges remain that require to be addressed for the technology to evolve further.
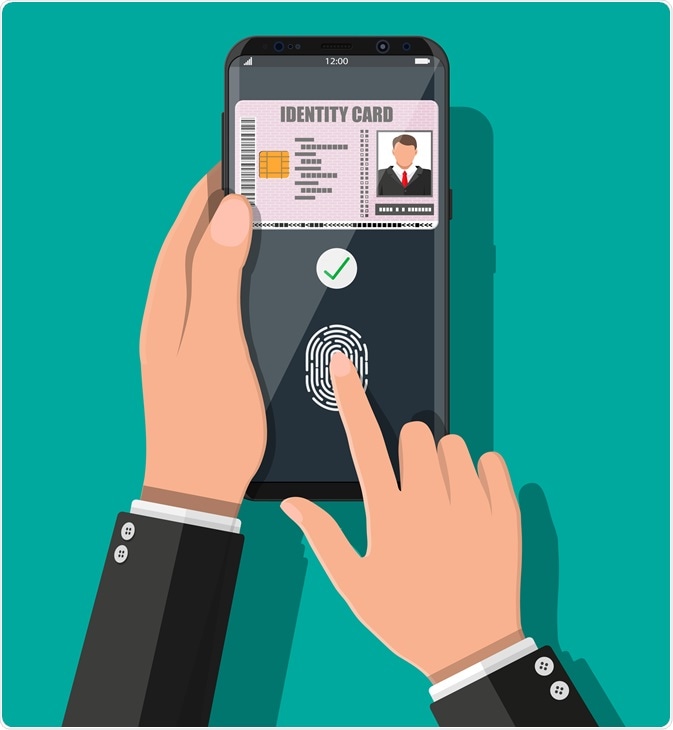
Image Credit: Abscent/Shutterstock.com
Improving the most common method for biometric identification
Considered as one of the most accurate methods for biometric identification, fingerprints can reliably distinguish the uniqueness of prints leading to its widespread usage for individual authentication.
The fingerprint scanner, which requires individuals to physically touch a computer scanner, remains the most widespread method for fingerprint authentication and biometric identification altogether. Typically, methods for fingerprint identification that require touching are known to distort and flatten the fingerprint, which reduces the accuracy of the stored image. Nonetheless, an estimated 98% of current fingerprinting procedures and equipment employed require the use of distortion-inducing contact methods.
In response, researchers have focused on the development of contactless fingerprinting technology to overcome the limitations of touch-based technology and advance fingerprint identification. The usage of contactless technology is now prevalent across modern devices from mobile phones to credit cards. Now, contactless fingerprinting has also revolutionized methods for fingerprint identification making touch-based systems antiquated in comparison.
Contactless fingerprinting avoids the limitations of touch-based methods as it does not distort or flatten the print, and technology has now taken contactless fingerprinting even further by implementing neural network analysis as well as 3D models to provide even more accurate identification. Such developments are particularly useful for many fields including law enforcement as well as the commercial sector that always looks towards a better and faster way of identifying individuals.
Nevertheless, the progress of contactless technology remains limited by issues that prevent further development.
Challenges to current contactless fingerprinting methods
In a study by Sam Mil'shtein and colleagues from The Advanced Electronic Technology Centre of the University of Massachusetts, Lowell, USA, researchers reviewed the progress of fingerprint analysis over the past 100 years and the challenges facing it in the future.
The work was published in 2011 in the journal Biometric Technology Today and demonstrates the increasingly frequent usage of fingerprinting technology from commercial applications to immigration control. Law enforcement is particularly reliant on fingerprint identification and the accuracy of the methodology.
Researchers focused on the range of applications of contactless fingerprinting relating to law enforcement, demonstrating that law enforcement faces key challenges.
This includes the necessity to confirm that a fingerprint is registered from an actual live finger, and not a decoy, which requires a subsystem capable of performing a liveliness test. This data exists for the USA and is stored is in the FBI's current fingerprint library, where fingerprints printed in wet ink are present by the hundreds of millions, but finding a faster and more reliable method is of urgent need.
Indeed, finding a way of accurately comparing and cross-referencing digitized fingerprints acquired via fingerprint scanners from older samples produced by wet ink technology, as well as new samples from contactless methods, is key to advancing contactless usage in law enforcement.
Additionally, the authors of the study discussed how statistical information of the resolution and accuracy from comparisons between digitized fingerprints and older printed fingerprints is missing. This also requires the development of another system that would be able to integrate old and new fingerprint data but remains to be developed.
The study also discusses the necessity to review fingerprint recognition algorithms, which have yet to be standardized, as they demonstrate varying precision. The size of files and processing speed of algorithms are also of key importance, as they affect the speed and accuracy of processing, and require updating that has yet to be undertaken.
Although these issues may seem limited to the application of contactless fingerprinting for law enforcement, the same challenges are present for commercial applications as well. For instance, new businesses looking to develop secure ways of payment based on contactless fingerprints require efficient and standardized algorithms. Nonetheless, new methods are being developed to address these challenges.
Addressing the challenges facing contactless fingerprinting by designing new methods
The emergence of new techniques of contactless fingerprinting has led to many alternative strategies to overcome the issues of accuracy, standardization, and speed, related to the current contactless methodology.
For instance, in recent years, the use of deep learning techniques using computational models has offered effective processes to classify, recognize, and represent objects and images, which can be applied to fingerprint technology. In a 2018 study published by Chenhao Lin and Ajay Kumar from the Department of Computing at The Hong Kong Polytechnic University, Hong Kong SAR, authors were able to develop a novel end-to-end contactless 3D fingerprint representation learning model based on a convolutional neural network (CNN).
The study offered a new framework to recover multi-view deep feature representation from contactless 3D fingerprints and is the first paper to address the challenging contactless partial 3D fingerprint identification problem. In the study, the authors demonstrated that experimental results on two publicly available databases indicate significantly improved performance over currently used state-of-the-art methods.
Researchers aimed to overcome the degradation of 3D fingerprint images that limit matching accuracy due to partial prints and proposes an alternative model that includes a fully convolutional network for fingerprint segmentation and three Siamese networks to learn multi-view 3D fingerprint feature representation. The neural network developed by the researchers is able to address the limitations of fingerprint identification during contactless 3D fingerprint acquisition by using extensive training datasets.
Another improvement upon current fingerprinting technology was developed by Steven Grosz and colleagues from Michigan State University and published in 2021. The authors were able to develop an end-to-end automated system called C2CL, which incorporates a mobile finger photo capture app as well as pre-processing and matching algorithms.
The system is able to avoid the challenges of previous fingerprint matching methods, namely issues with the low ridge-valley contrast of contactless fingerprints, the varying roll, pitch, yaw, and distance of the finger to the camera, non-linear distortion of contact-based fingerprints, and the different image qualities of smartphone cameras. These issues are addressed by segmenting, enhancing, scaling, and unwarping data using a unique pre-processing algorithm.
From 3 publicly available datasets, the researchers then demonstrated that the new algorithm performed with incomparable accuracy across existing contact to contact fingerprint matching systems.
By improving current methods as well as developing new systems that include more efficient, faster, and more accurate algorithms, the technology of contactless fingerprinting is improving rapidly. Nonetheless, for new technology, it is important to address existing and emerging issues that arise with the natural progression of biometrics as well as consider how easy it is to incorporate new technology within the technological infrastructure already in use.
Sources:
- C2CL: Contact to Contactless Fingerprint Matching (arXiv:2104.02811). (2021, April). Cornell University. https://arxiv.org/abs/2104.02811
- Lin, C., & Kumar, A. (2018a). Contactless and partial 3D fingerprint recognition using multi-view deep representation. Pattern Recognition, 83, 314–327. doi:10.1016/j.patcog.2018.05.004
- Lin, C., & Kumar, A. (2018b). Matching Contactless and Contact-Based Conventional Fingerprint Images for Biometrics Identification. IEEE Transactions on Image Processing, 27(4), 2008–2021. doi:10.1109/tip.2017.2788866
- Mil’shtein, S., Paradise, M., Bustos, P., Baier, M., Foret, S., Kunnil, V. O., & Northrup, J. (2011). Contactless challenges. Biometric Technology Today, 2011(3), 10–11. doi:10.1016/s0969-4765(11)70057-1
- Xia, X., & O’Gorman, L. (2003). Innovations in fingerprint capture devices. Pattern Recognition, 36(2), 361–369. doi:10.1016/s0031-3203(02)00036-5
Further Reading