The world population is estimated to reach nine billion by 2050, and agricultural productivity needs to increase to meet rising food demands. However, reduced soil quality and lack of arable land severely undermine agricultural expansion. Nevertheless, emerging technologies can provide much-needed support to improving agricultural productivity.
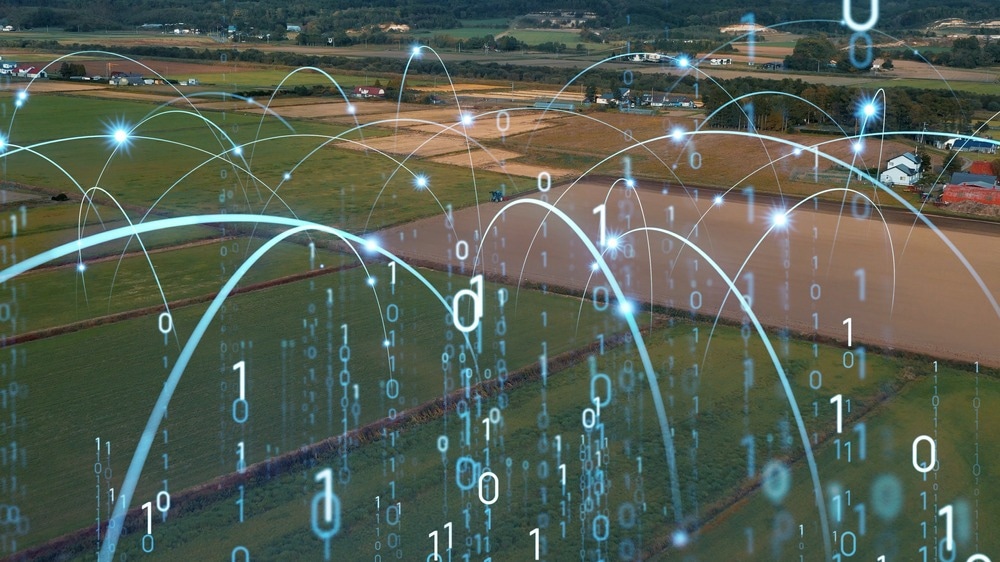
Image Credit: metamorworks/Shutterstock.com
The implications of the digital revolution and big data in agriculture
Over recent decades, the digital revolution driven by the advances in Information and Communication Technologies (ICT) has led to extensive innovations across industrial, financial, and agricultural sectors. Big data has transformed how information is collected, stored, and used within the ICT domain. For agriculture, big data enables greater productivity and profitability.
Big data refers to data of different types and sizes, arriving in increasing volumes and with more velocity. It is primarily characterized by these so-called “3-Vs” (i.e., variety, volume, velocity) and, therefore, cannot be handled by traditional data systems. This is particularly significant as information processing in the modern world can be collected across spatial and temporal scales and across many platforms and media.
As a result, big data is particularly relevant for agriculture, which can benefit from the information being collected, processed, and analyzed across scales and media. From individual agricultural plots to satellite-derived environmental data, processing large quantities of data is crucial to inform effective management decisions. This is particularly true in a rapidly changing world, as environmental conditions and socioeconomic factors increasingly impact food production.
The potential progress derived from big data for agriculture was reviewed by Himesh et al., 2018, who discusses the promising applications of big data. The authors discuss the rapid transformations within the agricultural sector, highlighting the use of big data throughout the food production process from farm to food. The review also presents how the transformation induced by the digital revolution appears irreversible, as the changes being made could alter how food production is designed, managed, and executed for the foreseeable future.
The same findings were echoed in a separate survey paper by Lytos et al. (2020), who used agriculture intelligence to refer to the recent advances in data collection and analysis using big data and intelligent technologies. Researchers present various implementations of technologies across agricultural systems through the intensive use of data, demonstrating the unprecedented capabilities of big data. The study concludes by discussing the future development of big data in agricultural sectors and attempts to bridge the knowledge gap between big data systems and agricultural applications.
Agricultural applications of big data
Reviews of literature discuss how processing big data can help to inform stakeholders on food productivity rapidly, accurately, and at a low cost. The primary aim of agriculture is to maximize land utilization and increase financial profits, which can both be improved by collecting and using data in different ways.
For instance, the widespread use of Geographic information systems (GIS) is a technology allowing researchers to create dynamic maps based on specific information (e.g., environmental, social, etc.). In recent years, big GIS analytics (BGA) in agriculture is becoming increasingly popular. This trend was reviewed by Sharma et al. in a 2018 study in which authors designed a BGA framework to improve the quality of GIS applications in agriculture.
Similarly, modern agriculture benefits from big data due to the emerging trends of precision agriculture. The origins of precision agriculture date to the late 1990s, as farmers began integrating GPS sensors into machinery to sense the surrounding environment during cultivation. The internet of things further accelerated the movement towards precision-guided farming, as wireless networks using online resources further improved management.
Precision agriculture is, therefore, the main force underlying the use of big data analytics in agriculture, as stakeholders are integrating ‘smarter’ technologies requiring extensive amounts and data types. Modern innovations are therefore focusing primarily on automated technologies, which rely on the processing and analysis of vast amounts of data. As a result, modern agriculture is becoming increasingly associated with big data.
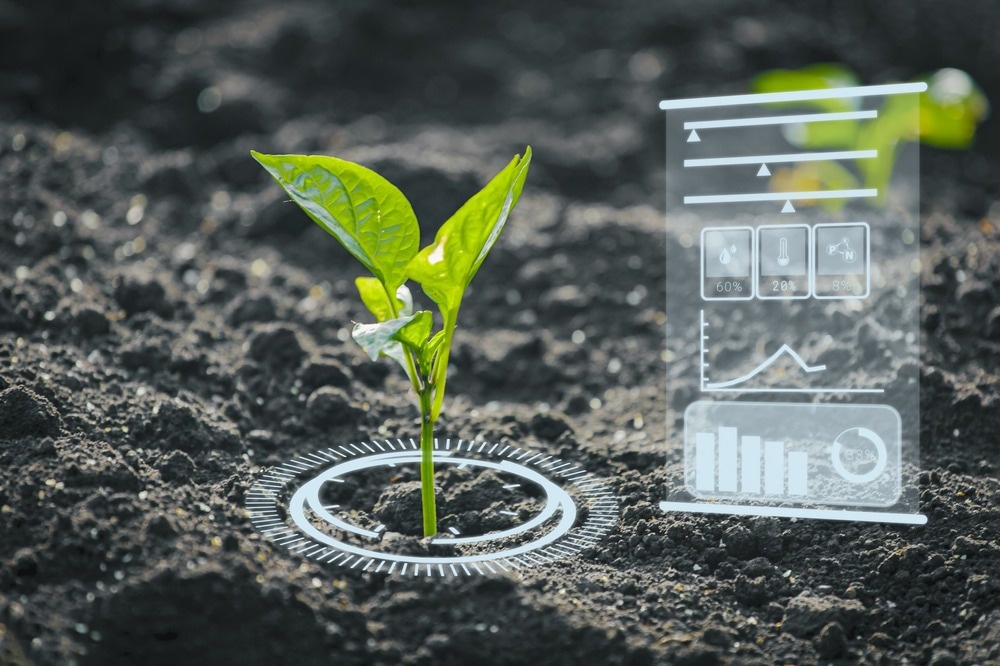
Image Credit: Leonid Sorokin/Shutterstock.com
Challenges to integrating big data analytics in agriculture
Although big data has extensive potential to improve agricultural management and food productivity, several limitations require consideration when implementing big data analytics. Emerging technologies, such as the ones used to collect and analyze big data, focus on producing more food on less land with fewer external inputs and a reduced ecological footprint. However, many barriers oppose this potential due to the lack of data interpretation.
The issues facing big data was reviewed by Weersink et al. (2018), who discuss how not all information that can be collected can be used to inform decisions for farmers. For instance, not all climatic variables may be valuable to predict crop health, and a selection process is required to eliminate unnecessary data to make big data analytics effective. Moreover, the problem is worsened as farmers may not be able to use or interpret such technological tools accurately, highlighting the need for education and awareness.
The authors review the barriers to realizing the potential for big data to revolutionize agriculture, particularly from a stakeholder’s perspective. Farmers themselves may be able to benefit from data analysis, but progress is needed to equip them with the necessary knowledge, toolkits, and financial support, to implement decisions from big data successfully. In turn, agricultural practices can use data to focus on environmental priorities by monitoring changes over time, ultimately demonstrating that big data represents a step toward healthier and more sustainable agricultural production.
Sources:
- Himesh, S., Rao, E. V. S. P., Gouda, K. C., Ramesh, K. V., Rakesh, V., Mohapatra, G. N., Rao, B. K., Sahoo, S. K., & Ajilesh, P. (2018). Digital revolution and Big Data: a new revolution in agriculture. CABI Reviews, 2018, 1–7. https://doi.org/10.1079/pavsnnr201813021
- Lytos, A., Lagkas, T., Sarigiannidis, P., Zervakis, M., & Livanos, G. (2020). Towards smart farming: Systems, frameworks and exploitation of multiple sources. Computer Networks, 172, 107147. https://doi.org/10.1016/j.comnet.2020.107147
- Misra, N. N., Dixit, Y., Al-Mallahi, A., Bhullar, M. S., Upadhyay, R., & Martynenko, A. (2022). IoT, Big Data, and Artificial Intelligence in Agriculture and Food Industry. IEEE Internet of Things Journal, 9(9), 6305–6324. https://doi.org/10.1109/jiot.2020.2998584
- Sharma, R., Kamble, S. S., & Gunasekaran, A. (2018). Big GIS analytics framework for agriculture supply chains: A literature review identifying the current trends and future perspectives. Computers and Electronics in Agriculture, 155, 103–120. https://doi.org/10.1016/j.compag.2018.10.001
- Weersink, A., Fraser, E., Pannell, D., Duncan, E., & Rotz, S. (2018). Opportunities and Challenges for Big Data in Agricultural and Environmental Analysis. Annual Review of Resource Economics, 10(1), 19–37. https://doi.org/10.1146/annurev-resource-100516-053654
Further Reading