Infectious diseases continue to be a major cause of death in human populations and are linked to various organisms, including bacterial, viral, and fungal. This diversity in the responsible pathogen means that the method utilized to treat the infection also varies considerably. Personalizing treatment for every different infection and the responsible organisms puts a tremendous amount of strain on public health resources.
One method to combat this drain on resources is to apply methods that simplify this process, such as computational methods; software can be adapted and used for various pathogens making the process simpler. This article will focus on understanding how computational protein design combats infectious diseases.
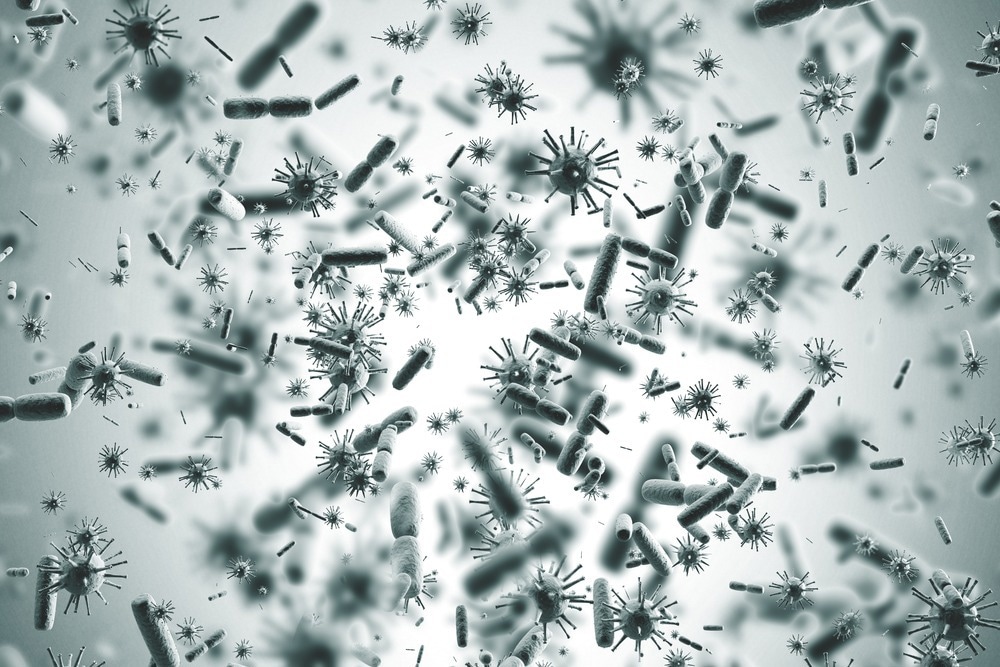
Image Credit: ImageFlow/Shutterstock.com
How is computation design used?
Computational methods are used at various stages of combating infectious diseases. Understanding the pathogen structure, the infection process, the rapid evolution, and adaptation to host features and pathogenicity remains an unsolved field. Genome assembly and sequencing can be used to tackle these blanks in the understanding of these processes and pathogens. Although genome sequencing is rapidly evolving, assembly software is struggling to keep pace.
Using genome sequencing, various pathogenic proteins can be modeled and used to understand intracellular processes, their replication, and within-host cell dynamics. Understanding the interactions with host genes can be used to design drugs that target long-term pathways and will remain effective over time.
Computational modeling can also occur at the populational level, which involves studying genomic data in an ecological context; this field of study is known as molecular ecosystems biology. It studies molecular interactions between organisms and their environment with an overarching aim of visualizing global patterns and predicting risks for infectious outbreaks that policymakers could use to evaluate potential intervention strategies.
Bacteria
Computation methods have been used to understand bacterial organisms' structure, treat different bacterial pathogens, and decrease antibiotic resistance.
A recent example is Shigella, a dysentery infection, for which there have been ongoing efforts to develop a vaccine; however, this requires a fundamental understanding of the host and bacterial interactions.
Machine learning algorithms and mathematical approaches have been used to study key immune interactions and identify that immunoglobulin-G (IgG) antibodies in the blood and immunoglobulin-A (IgA) antibody-secreting cells are associated with immune protection. It also determined that vaccines against certain proteins on the shigella surface are unlikely to provide protection.
Combating antibiotic resistance requires employing novel strategies such as using antibacterial peptides, components of the host defenses system, that have proven to kill resistant organisms. These peptides have been designed via computational modeling, studied peptide characteristics, and developed statistical predictors to optimize the molecular structure and design. These antibacterial peptides with modified and optimized structures have shown high potency against certain bacterial strains.
Viruses
Viral diseases have been studied using mathematical modeling focusing on human immunodeficiency virus (HIV), influenza infections, and, more recently, being adapted to study SARS-CoV-2. Modeling the immune system's response to different viruses can help optimize treatments.
Several models have been developed to better understand influenza infections to quantify the role of different immune responses in regulating influenza infection and other viral or bacterial co-infections. Modeling of the virus and host immune systems have been used to understand the spatiotemporal dynamics and the movement of the virus throughout the respiratory tract causing the infection. These models have provided valuable insight, such as extended shedding at the nasopharynx.
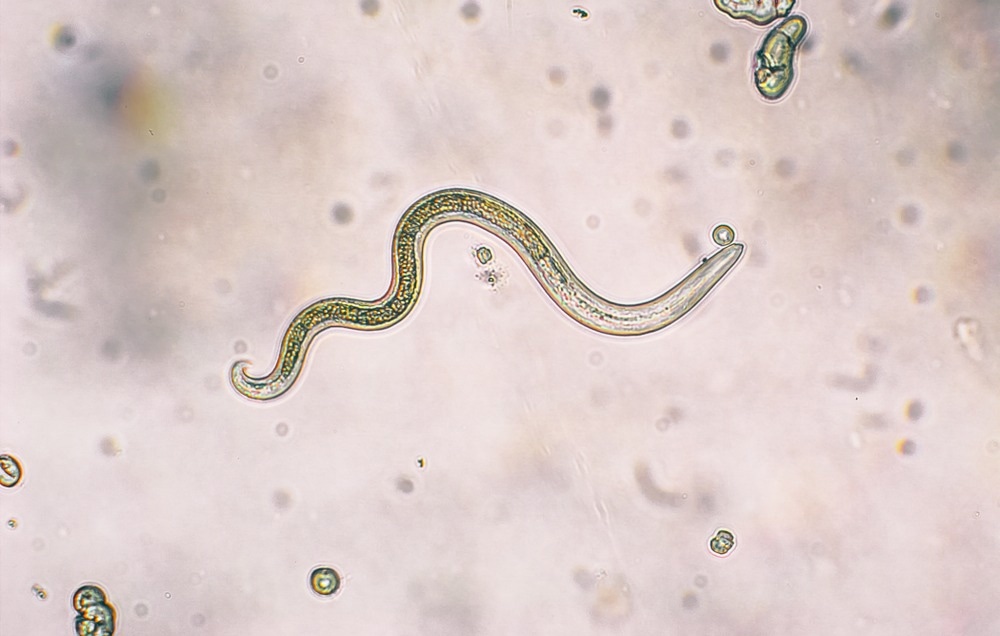
Image Credit: olgaru79/Shutterstock.com
Parasites
Malaria treatment has also vastly improved with the utilization of computational modeling. There have been several models that studied the within-host dynamics of malaria. These models have been used to predict infection outcomes by testing different preventative methods or anti-malarial treatments. These models have then been combined with statistical learning to optimize the data. This was also used to identify that the growth of Plasmodium falciparum, a severe type of malaria, was inhibited by cathepsin nine and matrix metalloproteinase 9. Modeling of these proteins suggested therapeutic use for malaria treatment.
Within-host models have also been coupled with pharmacokinetic and pharmacodynamic (PK/PD) models to predict mechanisms of action and the efficacy of many anti-malarial treatments. For example, modeling the anti-malarial drug SJ733 predicted the mechanism of action as inducing parasite clearance and with a half-life comparable with other rapid-acting treatments.
Computational design combined with other models has proven effective in combating various infectious diseases by preventing the spread of the disease or improving the treatment. Historically, modeling has provided great insight into the structure of pathogenic organisms and their interactions with the host and environment. As the field continues to grow and advance, it will play an even greater role in the fight against infectious diseases of various natures and origins.
Sources:
- Berglund, E. C., Nystedt, B., & Andersson, S. G. (2009). Computational resources in infectious disease: limitations and challenges. PLoS computational biology, 5(10), e1000481. https://doi.org/10.1371/journal.pcbi.1000481
- Garira W. (2018). A primer on multiscale modelling of infectious disease systems. Infectious Disease Modelling, 3, 176–191. https://doi.org/10.1016/j.idm.2018.09.005
- Jenner, A. L., Aogo, R. A., Davis, C. L., Smith, A. M., & Craig, M. (2020). Leveraging Computational Modeling to Understand Infectious Diseases. Current pathobiology reports, 8(4), 149–161. https://doi.org/10.1007/s40139-020-00213-x
Further Reading