What is a computational model?
Computational modeling is becoming an ever-increasing tool to simulate and study the behavior of complex systems through the use of mathematics, physics and computer science.
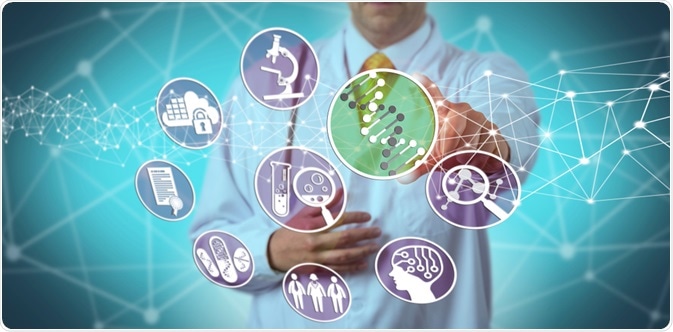
Image Credit: LeoWolfert/Shutterstock.com
The model itself contains numerous variables that are characteristic of the system being studied. To assess the impact of internal or external stimuli, each of these variables is adjusted either alone or in combination to observe how these changes affect outcomes.
The use of in silico techniques such as these can allow researchers to make predictions of what could happen in a real system in response to different stimuli and conditions.
These can be hugely beneficial to researchers by allowing them to conduct thousands of simulated experiments by computer to allow them to identify the ‘actual’ experiment that would be most beneficial to answer the research question at hand.
Within biomedical research today, a key feature of these models is that they can study a biological system at multiple levels from molecular processes through to organ level. This type of modeling is typically referred to as multi-scale modeling.
How can in silico research accelerate discovery?
New ‘omics’ technologies, when applied to molecular genetic analysis, have generated large amounts of raw data.
This raw data, through an advancing need of research laboratories to collaborate, has been shared alongside, molecular data, cellular data, population data, and large scale data. Through these techniques, it enables a fuller understanding of the biomedical complex system.
The generation of computational models aims to establish the greatest amount of understanding from a simplified system.
Drug discovery
In silico research is thought to be crucial in increasing the speed of the rate of drug discovery through the use of models rather than lab work and clinical trials which can otherwise be costly.
Over the past decade, the pharmaceutical industry has spent an estimated over $500 billion to produce only 227 new drugs- deemed new molecular entities.
Given the size of the pharmaceutical industry and the current low success rate, the use of more cost-effective methods can potentially have a positive impact on profits. A means of achieving this is through producing and screening drug candidates.
In 2010 using the protein docking algorithm EADock, researchers were able to identify a potential inhibitor that demonstrated significant anti-cancer activity in silico.
When translating this to an in vitro study 50% of these molecules were also active inhibitors.
The use of such algorithms harness the ability of algorithmic science and cloud computing to identify more compounds efficiently, whilst in a clinical setting, it could enable a prediction of patient response rates thus increasing the success rate at each stage of pharmaceutical trials.
Cell models
There have been extensive efforts made to establish a computational model of cellular behavior. There have been efforts to develop a model of tuberculosis to aid in the drug discovery process.
The key benefit of this is being faster than real-time simulated growth rates where pathological events of interest can be witnessed in minutes rather than months. Despite this, there are still significant problems in developing an exact, fully predictive model of a cells entire behavior.
The values required for the parameters require extensive experimental data.
Furthermore, should there be the presence of this data there are still constraints in the availability of computer processing power to force large simplifying assumptions that reduce the usefulness of present in silico cell models.
Challenges and future outlook
There are still multiple problems that need to be addressed when developing integrative models. Firstly, the reductionist-based approach from computation modeling allows scientists to gain an understanding of gene responses, protein responses, and cellular signaling pathways.
However, this approach ignores the data generated from in vitro and in vivo techniques- therefore a defined understanding of how these two approaches to research interact are needed.
Secondly, the use of a successful multi-scale model approach requires a database of variables. Whilst some databases address anatomical, genome and proteome data there is still limited physiological data from humans (both male and female) and animal studies under different physiological parameters.
The database would require physiological data from both normal and pathological values, thus giving a more comprehensive overview of the system in question. However, this data can only be generated from experimental animal data.
Sources
- Peter M.A. Sloot, Alfons G. Hoekstra, Multi-scale modeling in computational biomedicine, Briefings in Bioinformatics, Volume 11, Issue 1, January 2010, Pages 142–152
- Joseph Walpole, Jason A. Papin, and Shayn M. Peirce, Multiscale Computational Models of Complex Biological Systems, Annual Review of Biomedical Engineering 2013 15:1, 137-154
- Röhrig, Ute F.; Awad, Loay; Grosdidier, AuréLien; Larrieu, Pierre; Stroobant, Vincent; Colau, Didier; Cerundolo, Vincenzo; Simpson, Andrew J. G.; et al. (2010), "Rational Design of Indoleamine 2,3-Dioxygenase Inhibitors", Journal of Medicinal Chemistry, 53 (3): 1172–89, doi:10.1021/jm9014718,
Further Reading