Developing new compounds for therapeutic use presents major challenges no matter what the approach. Artificial intelligence (AI) has shown great potential for solving some of those challenges. Whether it is determining what new compounds will work or how to further optimize them, AI can help guide researchers and developers.
In this episode, Ashwini Ghogare, the intrapreneur CEO of Artificial Intelligence and Automation in Drug Discovery at Millipore Sigma, offers key insights into how AI can be used to help work out the synthesis of new compounds. The technology can also be used to predict ADME or physical-chemical properties.
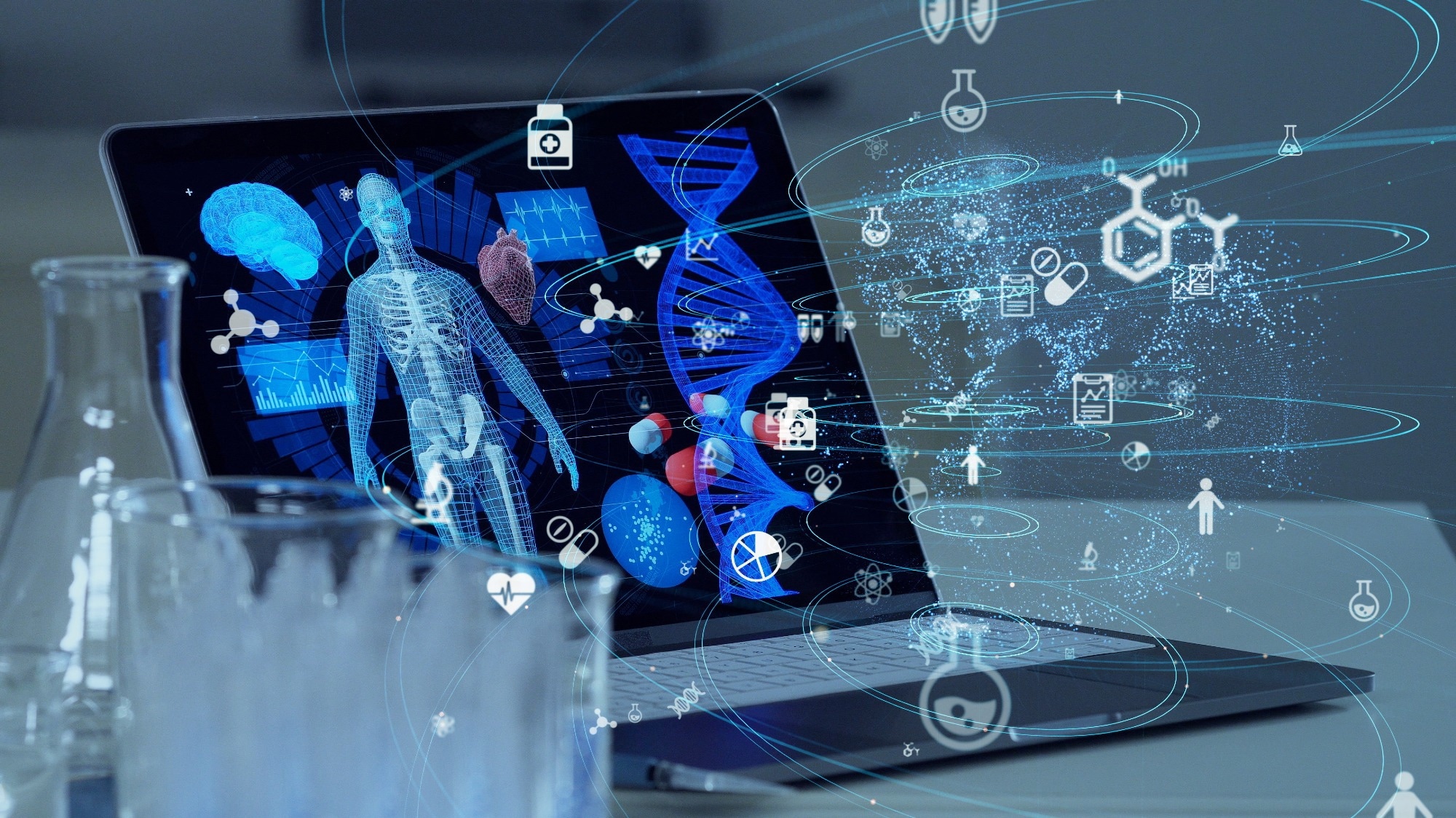
Image Credit: metamorworks/Shutterstock.com
When evaluating complex molecules, there is a lot to consider during synthesis due to the fact that some groups may be cross-reactive, etc. Thus, questions arise about creating what you intend to make and the level of success that comes with it. Ghogare explained how this is possible, for example, through the synthesis of component groups rather than following a linear process from start to finish.
Therefore, during the early phases, the aim is to determine whether it is possible to make a molecule and have it do what you want. Later on, comes the job of figuring out how to scale up the manufacturing process efficiently.
When it comes to predicting ADME properties, this is important as making drugs is expensive, and most will fail. However, if it was possible to have advanced knowledge regarding the ADME properties, it could lead to a reduction in the cost of development.
The main challenge here is that there is limited to no data available for training models. The data publicly available comes from published studies. However, to properly train a model, it is valuable to have negative data in the training set.
Merch KGAa is paving the way across the industry, taking advantage of sharing datasets for training without having to share or compromise any underlying data.
There can be a number of different reasons why the data can be negative, or drugs fail. Ashwini highlights that one way of addressing this situation is through automation, as it could remove potential sources of error.
Speaking about the future of pharmaceuticals, Ashwini envisions a world where not only are compounds synthesized through automation, but the machines themselves are autonomous. This would enable these machines to analyze data from the synthesized compounds and operate independently from that point forward.
About Life Science Marketing Radio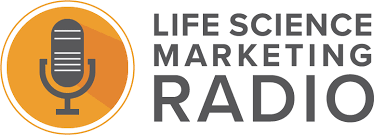
Life Science Marketing Radio is connecting life science professionals with the brightest minds and best thinking in the industry to grow their network and advance their careers.
Stay on top of new technologies around life science and embark on a journey to learn as much as possible on artificial intelligence, machine learning, and more.
Sponsored Content Policy: News-Medical.net publishes articles and related content that may be derived from sources where we have existing commercial relationships, provided such content adds value to the core editorial ethos of News-Medical.Net which is to educate and inform site visitors interested in medical research, science, medical devices and treatments.