Identification of genes has rapidly evolved with the advancements in molecular biology techniques and increased accessible data on genomics and functional genomics information. Bioinformatics helps identify genes within a long DNA sequence. This technique locates a gene simply by analyzing sequence data using a computer (in silico).
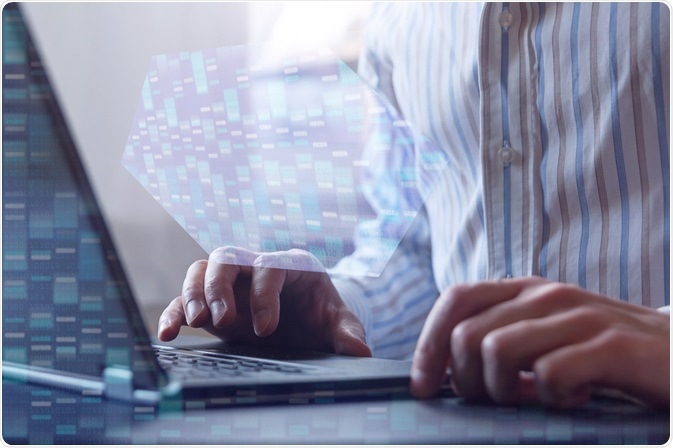
Image Credit: bluesroad/Shutterstock.com
One of the most essential aspects of bioinformatics is gene prediction. Gene prediction involves locating regions of genomic DNA that encode genes (protein-coding genes). Gene prediction or gene identification is extremely important because it helps scientists to distinguish between coding and non-coding regions of a genome, explain genes in terms of their function, conduct research related to detection, treatment, and prevention of genetic disorder diseases, etc.
Genes are identified broadly via two methods, i.e., a) similarity-based searches and b) Ab-initio prediction. These methods are briefly discussed below.
Similarity-based Searches
As the name suggests, this method of gene identification is based on sequence similarity searches. Similar genetic sequences are found between ESTs (expressed sequence tags), proteins, or other genomes and unknown genomes. This method assumes that exons (functional regions) are conserved evolutionarily than introns (nonfunctional regions).
The commonly used bioinformatics tool that is based on the similarity search method is BLAST. Other commonly used software are PROCRUSTES and GeneWise. This software predicts genes by using the global alignment of a homologous protein to translate open reading frames (ORFs) in a genomic sequence. However, CSTfinder is a software that uses pairwise genome comparison to identify genes.
Ab- initio Prediction
This method of gene identification is based on gene structure and signal-based searches. Ab initio gene predictions use known gene structure as a template to determine unknown genes. This method is based on two types of sequence information, namely, signal sensors and content sensors. Signal sensors include short sequence motifs, for example, start codons, stop codons, splice sites, and branch points.
On the other hand, content sensors rely on patterns of a codon that are unique to a species or in other words major distinct features present in the gene. This allows coding sequences to stand out from the surrounding non-coding sequences by statistical detection algorithms. Researchers use this method for the detection of an exon.
Many algorithms are being used for modeling gene structure, e.g., linear discriminant analysis, dynamic programming, hidden Markov model, linguist methods, and neural networks. These models have helped develop many ab initio gene prediction programs such as FGENESH, GeneID, GeneParser, GENSCAN, GlimmerM, etc.
Bioinformatic Tools Used for Gene Identification
CRAIL: It is one of the most commonly known computational tools used for ORF identification. This tool provides important information such as splice junctions, translation start points, and non-coding scores of 60 base regions on both sides of the putative exon.
GLIMMER: Glimmer is a software used for finding genes in microbial DNA, especially the genomes of bacteria and archaea. Gene Locator and Interpolated Markov Modeler (Glimmer) uses interpolated Markov models (IMMs) to recognize the coding regions and differentiate them from noncoding DNA.
GenScan: This tool is used for the identification of complete gene structures in genomic DNA for various organisms. It can predict exon-intron structures of genes as well as locations in genomic sequences.
Genie: This gene finder is based on generalized hidden Markov models. Genie was developed as a collaborative project by the University of California’s Computational Biology Group, Lawrence Berkeley National Laboratory’s the Human Genome Informatics Group, and the Berkeley Drosophila Genome Project.
Gene Finder: This tool is used to predict splice sites. It can also identify protein-coding exons, construct gene models, and recognize the promotor and poly-A region.
ORF Finder: This is a graphical analysis tool that can detect open reading frames along with their protein translation from sequences already in the database. This program is used to search new DNA sequences for potential protein-encoding segments.
Easy Gene: This tool is used to identify genes in prokaryotes, the current version of which includes 138 different organisms. Each gene identified by Easy Gene is attributed with a significant score (R-value), which reveals the probability of a sequence to be a non-coding open reading frame rather than a real gene.
Gene Publisher: This program performs automated data analysis from gene expression experiments on several different platforms. This tool also accepts Affymetrix CEL files or gene tables as inputs and conducts detailed numerical and statistical analysis. It connects its result with the available data across various databases and finally produces a cumulative report of the result.
ORPHEUS: This software is used to predict genes from large genomic fragments or complete bacterial genomes.
HMMgene: This program is based on the hidden Markov model and is used to predict genes in anonymous DNA. It can predict whole or partial genes as a result of which it can identify exons and can splice precisely. It can also predict start/stop codon and splice genes. It is used to identify the genes of vertebrates.
Promoter: This software is based on neural networks and genetic algorithms. It can predict transcription start sites of vertebrate PolII promoters in DNA sequences.
Sources:
- Chavali, A.K. and Rhee, S.Y. (2018). Bioinformatics tools for the identification of gene clusters that biosynthesize specialized metabolites, Briefings in Bioinformatics, 19(5), pp. 1022–1034, https://doi.org/10.1093/bib/bbx020
- Ghorbani, M. and Karimi, H. (2015). Bioinformatics Approaches for Gene Finding. International Journal of Scientific Research in Science and Technology, 4(1), pp. 12-15.
- Wang, Z. et al. (2004). A brief review of computational gene prediction methods. Genomics, proteomics & bioinformatics, 2(4), pp. 216–221. https://doi.org/10.1016/s1672-0229(04)02028-5
- Koonin, E.V., and Galperin, M.Y. (2003). Sequence - Evolution - Function: Computational Approaches in Comparative Genomics. Boston: Kluwer Academic. Chapter 4, Principles and Methods of Sequence Analysis. [Online] Available at: https://www.ncbi.nlm.nih.gov/books/NBK20261/
Further Reading