Artificial Intelligence (AI) is set to revolutionize the field of microscopy. Recent years have seen numerous research teams successfully integrate AI into vision applications.
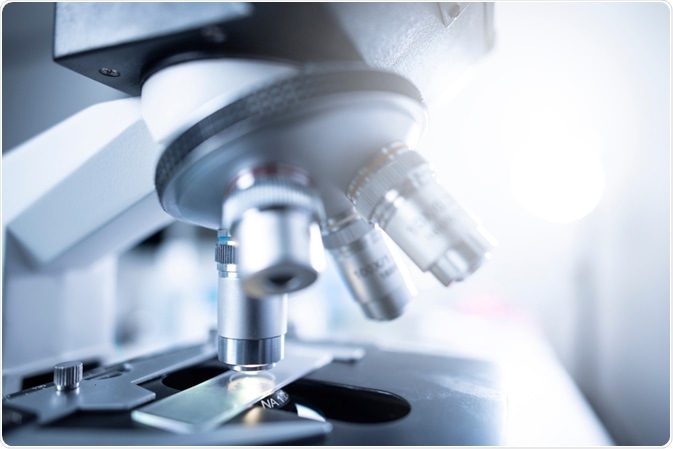
Image Credit: Chokniti Khongchum/Shutterstock.com
These innovations, driven by Deep Learning (DL) and Deep Neural Networks (DNNs) are opening up a new realm of possibility in the biomedical sector.
Several major players in the global tech industry are developing methods using DL that offer biologists a means of extracting more information than ever before about the images they create with microscopy techniques.
Google uses deep learning to identify cell types
Several years ago, researchers at the Gladstone Institutes and the University of California created a robotic microscopy technique that was able to record data from single cells over an extended period.
Google had been looking for such a vast set of data to apply its deep learning approach to, so they teamed up with a plan of focusing on blue-sky ideas (those problems that humans cannot solve, or can but computers can do much faster).
The team set out to develop a neural network that could investigate images created by microscopy techniques to identify information that human researchers were unable to. In particular, the team considered that images taken of cells using light microscopy often reveal little regarding the more complex nature of the cell, to reveal this information, scientists use staining methods.
The team wanted to explore whether a neural network could be trained to identify features from unstained cells, making the process faster and more efficient.
After some years of development, the team published the findings of their work in the journal Cell in 2018. They demonstrated that they had trained a neural network to distinguish cell types as well as subcellular structures (e.g. nuclei and neuronal dendrites) from images for unstained cells. The team followed up on this by successfully using their method to identify stem cell-derived endothelial cells in unstained samples.
The process of incorporating AI with microscopy results in giving scientists access to a deeper level of information about their cell samples without the need for more complex imaging techniques involving staining that are costly and time-consuming.
Until this collaboration between the Gladstone Institutes, the University of California, and Google, there was almost no work using deep learning in microscopy. But now, it is changing the landscape of image analysis, opening up new capabilities, and streamlining analytical processes.
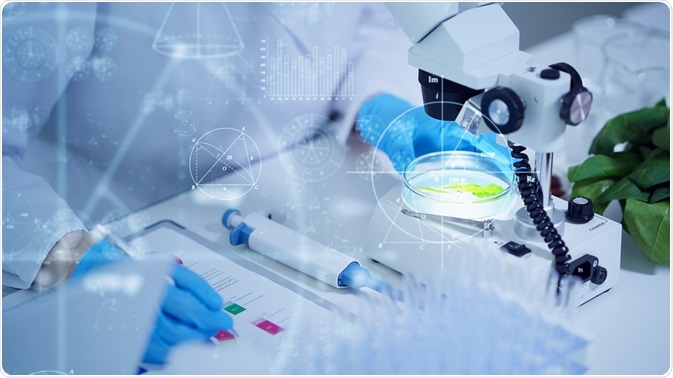
Image Credit: metamorworks/Shutterstock.com
The growth of AI use alongside microscopy
There are now several systems that successfully use deep learning to identify cells. Each work in slightly different ways. For example, a team at the University of California developed an approach that can identify pollen and fungal spores in the air via a mobile phone microscope and a deep learning network. The technique is 94% accurate.
A team in Poland recently developed deep learning algorithms that can identify the genus and species of bacteria from microscopy images, an essential part of agriculture, food safety, and medical research.
Finkbeiner, from the team at the Gladstone Institutes, has recently joined forces with the Michael J. Fox Foundation for Parkinson’s Research to explore how AI can be used with microscopy to determine features of human cell types that could improve diagnosis of disease.
The team intends to innovate a way to differentiate stem cell-derived neurons taken from with Parkinson’s disease, from those taken from healthy cells using a neural network. They were successful in developing AI that can determine healthy cells from diseased cells using several markers, transforming how disease screening can be conducted.
The field of oncology is also set to benefit from advancements in the use of AI alongside microscopy. For many years, machine learning has been applied to help analyze the micrographs of biopsy samples.
Recently, deep learning has been increasingly used to improve both diagnosis and treatment. AI can be trained on images that have already been analyzed by a pathologist, which allows the deep learning models to learn how to classify tumors, as well as to assess the level of cancer progression.
Methodologies using AI have proven to be more accurate than clinicians in most cases.
How does it work?
Deep learning models adopt an agnostic approach. This means that rather than forcing the markers into distinct categories it decides what might be the best candidates for certain categories. However, in essence, the system learns by itself.
Scientists cannot instruct Artificial Intelligence on how to learn, and in turn, the AI cannot tell the scientist how it learned something.
The future for microscopy and artificial intelligence
AI’s involvement with microscopy techniques is just in its infancy, and further developments can be expected in the coming years. Currently, it is helping with the identification of cell type and the deeper analysis of cell samples for research and diagnostic purposes.
In addition, it is helping expand possibilities for biomedical applications. Finally, it is changing the status quo in terms of how researchers are collecting and analyzing microscopy data.
Sources:
- Artificial Intelligence Sees More in Microscopy than Humans Do. Available at: www.the-scientist.com/.../artificial-intelligence-sees-more-in-microscopy-than-humans-do-65746
- Christiansen, E., Yang, S., Ando, D., Javaherian, A., Skibinski, G., Lipnick, S., Mount, E., O’Neil, A., Shah, K., Lee, A., Goyal, P., Fedus, W., Poplin, R., Esteva, A., Berndl, M., Rubin, L., Nelson, P. and Finkbeiner, S., 2018. In Silico Labeling: Predicting Fluorescent Labels in Unlabeled Images. Cell, 173(3), pp.792-803.e19. https://www.cell.com/cell/fulltext/S0092-8674(18)30364-7
- Kim, D., Min, Y., Oh, J. and Cho, Y., 2019. AI-powered transmitted light microscopy for functional analysis of live cells. Scientific Reports, 9(1). https://www.nature.com/articles/s41598-019-54961-x#citeas
- von Chamier, L., Laine, R., and Henriques, R., 2019. Artificial intelligence for microscopy: what you should know. Biochemical Society Transactions, 47(4), pp.1029-1040. portlandpress.com/.../Artificial-intelligence-for-microscopy-what-you
- Yu, K., Zhang, C., Berry, G., Altman, R., Ré, C., Rubin, D., and Snyder, M., 2016. Predicting non-small cell lung cancer prognosis by fully automated microscopic pathology image features. Nature Communications, 7(1). https://www.nature.com/articles/ncomms12474#citeas
- Zieliński, B., Plichta, A., Misztal, K., Spurek, P., Brzychczy-Włoch, M., and Ochońska, D., 2017. Deep learning approach to bacterial colony classification. PLOS ONE, 12(9), p.e0184554. journals.plos.org/plosone/article?id=10.1371/journal.pone.0184554