Though Raman Spectroscopy has been in use for almost a century now, the advancements and refining of this methodology have morphed the entire practice, save for the underlining principle.
Presently, the frontier of Raman Spectroscopy is seeing a rise in machine learning to improve the resolution and quality of resulting spectra. This field is still in its infancy; however, we have already received a glimpse of how it can be used in conjunction with monitoring active transport, machine learning, and production and manufacturing.
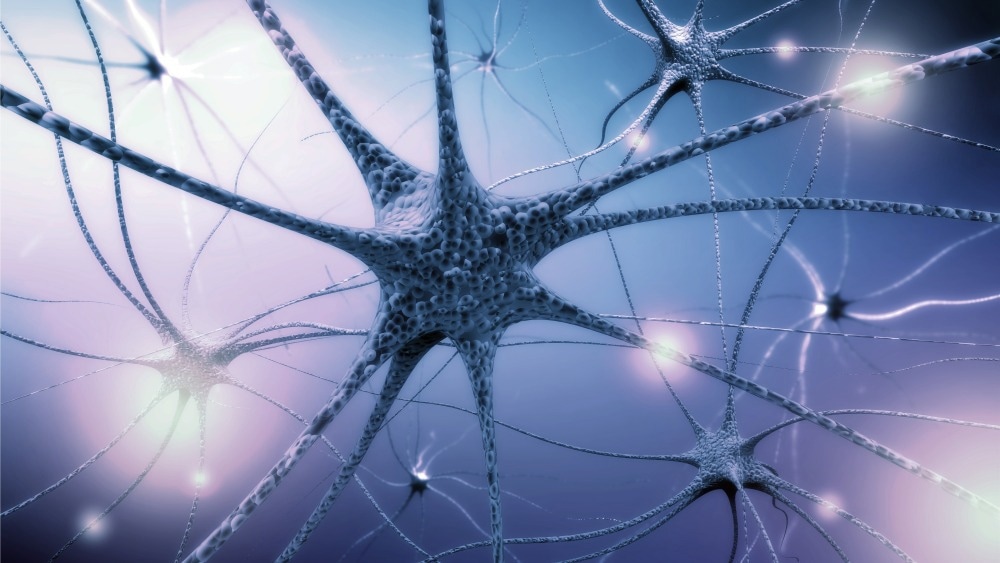
Image Credit: Billion Photos/Shutterstock.com
How Surface Enhanced Raman Scattering (SERS) can be Used to Monitor Neurotransmitter Activity
A recent advancement that holds a lot of potential in the future of cell signaling and neurology is the detection of cell secretion events using Raman Spectroscopy. In the past, measurements pertaining to active transport, transfection, and cell-secreting interactions have been difficult to attain because of the rate and scale at which these exchanges occur.
Some success in “active transport recording” has been achieved using the patch clamp method, where a glass pipette with a small opening can contact an ion channel atop a neuronal membrane and proceed to measure the small electrical current using amplifiers.
However, this does not grant us a macroscopic view of cellular exchange and can only record the flow through a single ion channel. In addition, this technique fails to expound upon the identity of the different molecules passing through said channel. The patch clamp method is mainly used to compare the voltage of different channels.
To achieve a better analysis of the atomic identities between cell exchanges, the use of surface-enhanced Raman scattering (SERS) can be employed. Like electrophysiology techniques, this method will provide signals corresponding to the differences in ion concentration within the cell, measured in volts.
The superior nature of this technique is found in the ability to detect the unique vibrational spectra corresponding to one molecule or atom. This means that the identity of one molecule (at a time) can be detected by viewing how the light interacts with the bonds of said molecule. Machine learning can assign a spectral signature to the molecule from here.
Placing the glass nanofiber near the surface of the cell (<10 microns) after subjecting it to the following conditions:
- 0.3% (3-Aminopropyl)triethoxysilane (APTES) in EtOH
- Rinse in anhydrous EtOH
- Expose to specified nanoparticles
- Treatment using O2 plasma
After stimulating the cell in such a way to release certain molecules, they can bind to the tip of the Raman scattering nanosensor, which results in the real-time data acquisition of a known analyte.
A practical example of this technique can be used in monitoring sodium currents between axons, relating to electrochemical activity between the presynaptic terminal and the postsynaptic neuron. This activity can be monitored by the millisecond and allows us to see how certain conditions will affect the release of neurotransmitters.
How Machine Learning is Implemented in Raman Spectroscopy
The categorized data sets from the Raman Spectroscopy make it a prime candidate for supervised algorithms, which are a subcategory of machine learning. These supervised algorithms provide labels to data without user input, which maximizes the recognition of elements and features associated with those labels. A practical example can be seen in the spectral analysis of pure compounds.
The AI software will begin labeling a compound of choice. The more analogs the machine labels, the more the algorithm is “trained” to recognize that specific structure. When assayed with Raman spectroscopy, it will begin maximizing recognition of the spectroscopic bands that result from that structure. Members of the University of Montreal have applied this technique to glucose surveillance to ATP and ADP in different biological mediums.
Juxtaposing these supervised algorithms are the unsupervised algorithms. These differ in the sense that no information is granted regarding the analyte's identity. In this way, the algorithms generate groups based on spectral differences alone. This methodology is employed when the analyte in question, or the origins of the spectral graph, are unknown.
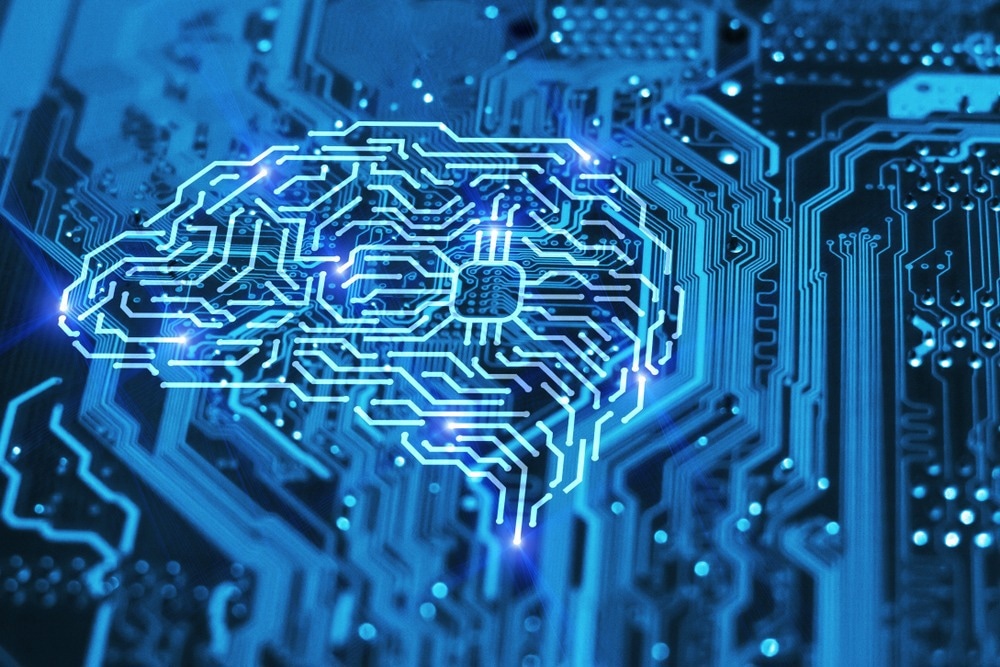
Image Credit: Michael Traitov/Shutterstock.com
How Machine Learning is integrated into Nanoparticle Production
An interesting intermingling can be found between Raman spectroscopy, machine learning, and control synthesis. Control synthesis is creating automated control strategies tailored to specific goals. In a paper produced by Mekki-Berrada et al. (2021), the reader is introduced to how silver nanoparticles can be synthesized with the desired absorbance spectrum, and dimensions, using Raman spectroscopy. Additionally, this is accomplished through the implementation of feedback loops.
In this paper, the desired silver nanoparticles are synthesized by continuously altering the flow rate between the water (solvent), AgNO3, silver seeds, ascorbic acid, tribasic dihydrate, polyvinyl alcohol, and oil through Hamilton glass syringes. The production of nanoparticles can present many challenges. This is because it is difficult to control the non-linear growth of silver seeds with that of pre-existing models when it is at the nanometer scale (as each is designed to be 10nm high and 50nm long).
Manufacturing conditions are first implemented using baseline calculations that relate to the five input valves corresponding to the five reagents. Once flow synthesis is performed through the droplet-based microfluidic platform, supervised algorithms can be implemented to ensure that each silver particle is made uniformly. By scrupulously monitoring the optical fingerprint of each nanoparticle, the AI software can discern just how concentrated each of the five reagents should be and how much pressure should be applied to each glass syringe.
Sources:
- Mekki-Berrada, F., Ren, Z., Huang, T. et al. Two-step machine learning enables optimized nanoparticle synthesis. npj Comput Mater 7, 55 (2021). https://doi.org/10.1038/s41524-021-00520-w
- Jean-Francois Masson, Julien Breault-Turcot, Rita Faid, Hugo-Pierre Poirier-Richard, Hélène Yockell-Lelièvre, Félix Lussier, and Joachim P. Spatz (2014) Plasmonic Nanopipette Biosensor. Analytical Chemistry 2014 86 (18), 8998-9005 DOI: 10.1021/ac501473c
- Hu Zhu, Félix Lussier, Charles Ducrot, Marie-Josée Bourque, Joachim P. Spatz, Wenli Cui, Li Yu, Wei Peng, Louis-Éric Trudeau, C. Geraldine Bazuin, and Jean-Francois Masson. (2019) Block Copolymer Brush Layer-Templated Gold Nanoparticles on Nanofibers for Surface-Enhanced Raman Scattering Optophysiology. ACS Applied Materials & Interfaces 11 (4), 4373-4384 DOI: 10.1021/acsami.8b19161
- Gomez-Diaz, C., Bargeton, B., Abuin, L. et al. A CD36 ectodomain mediates insect pheromone detection via a putative tunnelling mechanism. Nat Commun 7, 11866 (2016). https://doi.org/10.1038/ncomms11866
- Neugebauer U, Heinemann SH, Schmitt M, Popp J. Combination of patch clamp and Raman spectroscopy for single-cell analysis. Anal Chem. 2011 Jan 1;83(1):344-50. doi: 10.1021/ac1024667. Epub 2010 Dec 8. PMID: 21141833.
Further Reading