Scientists have often demonstrated their interest in genetically modifying tree species for a wide range of applications, ranging from paper to biofuel production while staying clear of those modifications that lead to unexpected outcomes.
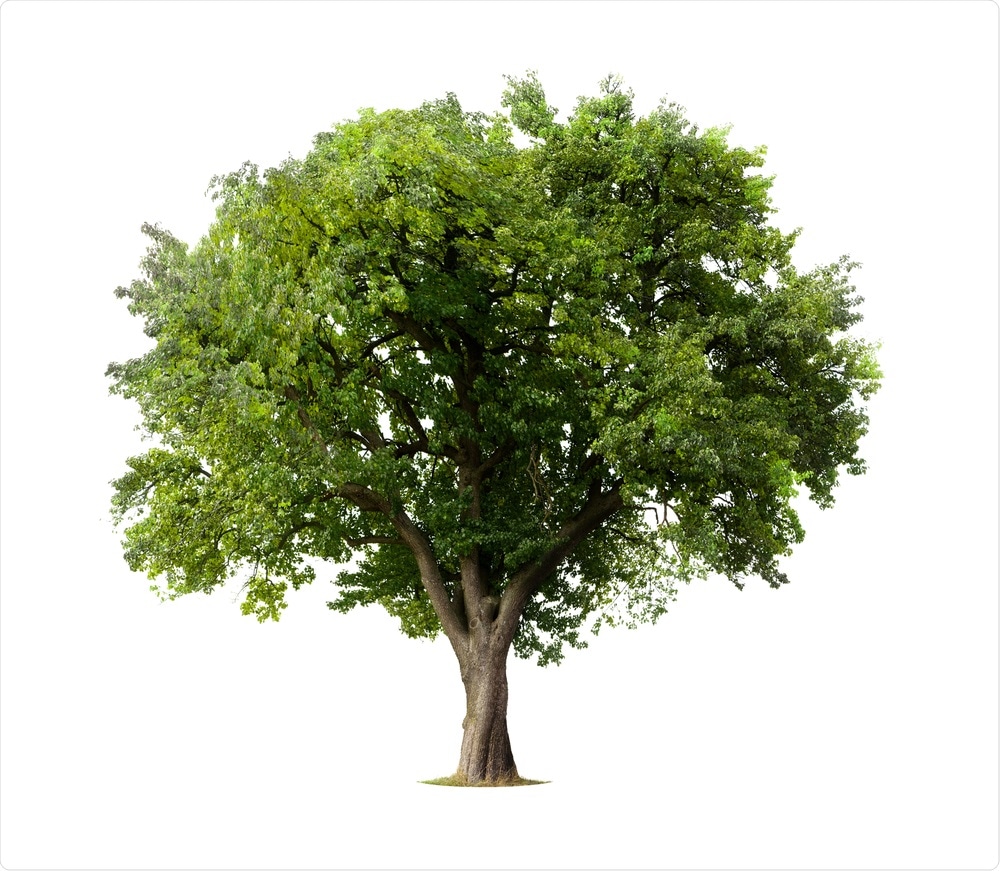
Image Credit: Jan Martin Will/Shutterstock.com
Such outcomes can emerge when planned modifications made to one gene lead to surprising modifications to other types of genes.
Now, an innovative model intends to predict these modifications, which would help prevent accidental consequences, and perhaps forge a path for more efficient studies in the areas of forestry and genetic modification.
The study in question focuses on a complex material called lignin, which is present in trees and helps to give a structure to trees. Lignin is what makes wood feel like wood.
Whether you want to use wood as a biofuel source or to create pulp and paper products, there is a desire to modify the chemical structure of lignin by manipulating lignin-specific genes, resulting in lignin that is easier to break down. However, you don’t want to make changes to a tree’s genome that compromise its ability to grow or thrive.”
Cranos Williams, Associate Professor, Department of Electrical and Computer Engineering, North Carolina State University
Williams is also the study’s corresponding author.
The scientists focused on a specific type of tree known as Populus trichocarpa, a model tree that is used extensively for research—this means, researchers who study tree biology and genetics devote plenty of time analyzing the Populus trichocarpa.
Previous research generated models that predict how independent changes to the expression of lignin genes impacted lignin characteristics.”
Megan Matthews, Study First Author and Postdoc, University of Illinois
Matthews was a former Ph.D. student at North Carolina State University.
Matthews continued, “These models, however, do not account for cross-regulatory influences between the genes. So, when we modify a targeted gene, the existing models do not accurately predict the changes we see in how non-targeted genes are being expressed. Not capturing these changes in expression of non-targeted genes hinders our ability to develop accurate gene-modification strategies, increasing the possibility of unintended outcomes in lignin and wood traits.
“To address this challenge, we developed a model that was able to predict the direct and indirect changes across all of the lignin genes, capturing the effects of multiple types of regulation. This allows us to predict how the expression of the non-targeted genes is impacted, as well as the expression of the targeted genes,” he further added.
Another of the key merits of this work, versus other models of gene regulation, is that previous models only looked at how the RNA is impacted when genes are modified. Those models assume the proteins will be impacted in the same way, but that’s not always the case. Our model is able to capture some of the changes to proteins that aren’t seen in the RNA, or vice versa.”
Megan Matthews, Study First Author and Postdoc, University of Illinois
Matthews added, “This model could be incorporated into larger, multi-scale models, providing a computational tool for exploring new approaches to genetically modifying tree species to improve lignin traits for use in a variety of industry sectors.”
That means, by modifying a single gene, scientists can inadvertently skew things up with other types of genes, producing trees that were not what they had originally planned for. But with the help of the latest model, scientists can work out a plan to prevent that problem.