Artificially synthesizing genomes has numerous applications, including medical research and industrial strains. Scientists are continuously progressing in the depth and breadth of genome design and synthesis, from Craig Venter’s team’s synthesis of the artificial life JCVI-syn1.0 in 2010 to the rewriting and synthesis of the prokaryotic E. coli genome, and the Sc2.0 project’s artificial synthesis of the yeast genome.
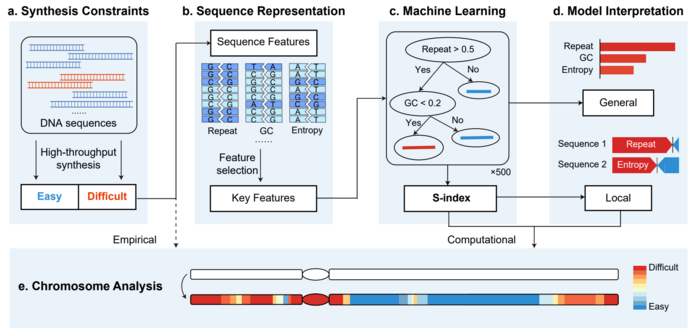
A, Collection of the DNA sequences obtained from high-throughput synthesis. The sequences were classified into easy-to-synthesize (blue) or difficult-to-synthesize (red). B, Graphical representations of DNA sequences: repeat, GC content, information entropy, and other types of features. Key features were identified from these sequence features by machine learning methods. C, The XGBoost algorithm was utilized to build the classification model and calculate the S-index. D, Methods used to interpret the model. The feature contributions were quantified according to the global importance scores and local SHAP explanations. e, Application of the S-index on a specific chromosome. The heatmap indicates the synthesis difficulties for the different fragments, which range from difficult (red) to easy (blue). The white sequences indicate the unanalyzed chromosome sequence. Image Credit: ©Science China Press
But some gene segments continue to be difficult to synthesize, resulting in the inability to complete artificial chromosomes, limiting the application and promotion of artificial genome synthesis technology. To solve this issue, Tianjin University’s Professor Yingjin Yuan’s team created an interpretable machine learning framework that can forecast and quantify the complexity of chromosomal synthesis, offering assistance for optimizing chromosome design and synthesis processes.
By evaluating data from a vast number of known chromosome fragments, the study team developed an effective feature selection method and found six important sequence features that cover energy and structural information during DNA chemical synthesis and assembly.
Based on these findings, the researchers created the eXtreme Gradient Boosting (XGBoost) model, which can accurately forecast the synthesis challenges of chromosomal fragments. The model achieved an AUC (area under the receiver operating characteristic curves) of 0.895 in cross-validation and an AUC of 0.885 on an independent test set in partnership with a DNA synthesis company, exhibiting good precision and predictive ability.
To analyze and explain the synthesis difficulties of chromosomes, the study team presented a Synthesis difficulty Index (S-index) based on the SHAP method.
The study discovered that different chromosomes had significantly different synthesis difficulties, and the S-index could quantitatively explain the causes of synthesis difficulties for some gene fragments, providing a foundation for chromosome sequence design and synthesis and enhancing the effectiveness and success rate of designer chromosome synthesis.
This accomplishment is a useful tool for chromosomal engineering and genome rewriting researchers, and it is intended to provide more complete guidance and support for chromosome design and synthesis.
Source:
Journal reference:
Zheng, Y., et al. (2023). Machine learning-aided scoring of synthesis difficulties for designer chromosomes. Science China-Life Sciences. doi.org/10.1007/s11427-023-2306-x.