By Pooja Toshniwal PahariaReviewed by Lexie CornerMay 12 2025
A recent study published in Scientific Reports introduces an advanced method for detecting and classifying Shiitake mushrooms using the mamba-YOLO deep learning technique.
This approach supports mechanized harvesting, automated detection, and accurate quality grading, addressing long-standing challenges in mushroom farming such as labor-intensive manual picking and low efficiency.
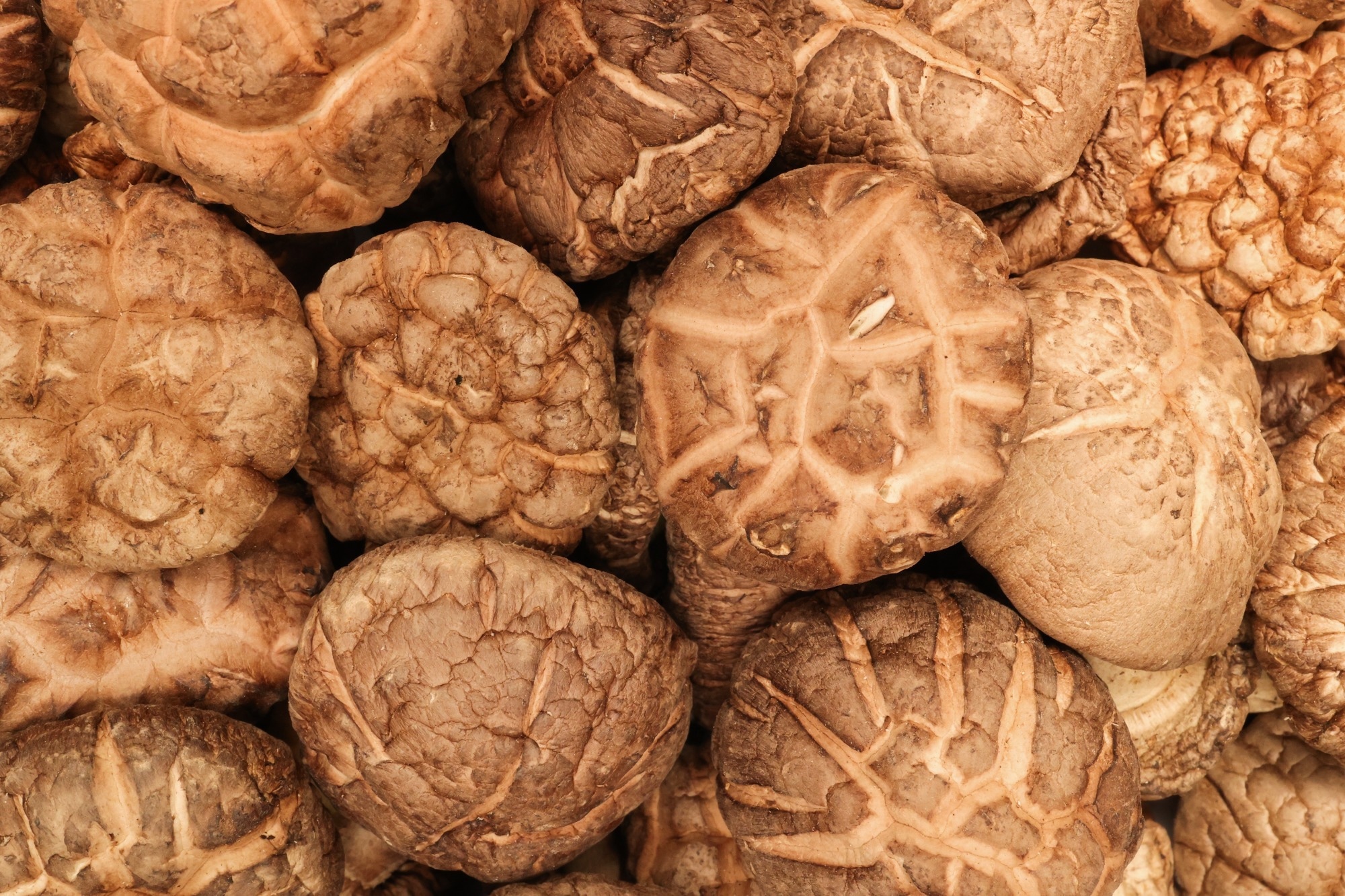
Image Credit: WHOEXPLORER/Shutterstock.com
Deep learning algorithms make it possible to identify Shiitake mushrooms in complex growing conditions, including environments with small, immature mushrooms, dense clusters, and overlapping growth.
The study highlights the broader potential of deep learning models and high-performance detection algorithms in biological classification, using Shiitake mushrooms as a practical case.
Challenges in Current Cultivation and Harvesting Methods
Shiitake mushrooms are widely valued for their nutritional content, savory flavor, and health benefits, including immune support, cardiovascular health, and longevity. Despite their popularity, commercial cultivation remains difficult due to weather risks like rain damage. The industry is shifting toward more efficient and sustainable practices through factory-based production and smart farming technologies.
Substitute cultivation is the primary method used to grow Shiitake mushrooms. It’s favored for its simplicity, short production cycle, and high yield. However, manual harvesting still dominates the industry and demands significant human and material resources. Automating this process would help address the inefficiencies and physical demands of manual labor.
About the study
In this study, the researchers present a method for identifying and grading Shiitake mushrooms based on maturity and cap surface characteristics, using the mamba-YOLO model. They built a custom dataset featuring a variety of growth conditions, including isolated mushrooms, as well as those partially hidden or overlapping.
The dataset was collected from the Qihe 9 variety, grown using substitute cultivation at the Biological Intelligent Factory in Shandong Province between July and August 2024.
Images were captured using a smartphone positioned 40 cm from the mushrooms. These were then resized to 480 × 640 pixels using Photoshop and divided into training (1,624 images), validation (465 images), and test (231 images) sets.
How the Model Works
The mamba-YOLO model integrates several key modules. The RGBlock module captures diverse features with minimal computational cost. LSBlock enhances feature extraction to handle complex visual inputs. The Vision Clue Merge module consolidates features from different levels to boost detection performance.
The model also incorporates a Pyramid Attention Feature Pyramid Network (PAFPN), which processes both low- and high-resolution features to improve accuracy in identifying and classifying mushrooms. Its "head" component uses convolutional layers for object detection, allowing the system to adapt to mushrooms of various sizes and real-world conditions. Model training is optimized with the Stochastic Gradient Descent (SGD) algorithm.
Mushrooms are classified based on maturity and cap surface texture into six categories: plane-surface immature (p-immature) and mature (p-mature); cracked-surface immature (c-immature) and mature (c-mature); and deformed-surface immature (d-immature) and mature (d-mature). Each mushroom stick is also labeled for easier identification during harvesting. The system’s decision-making is supported by state space models (SSMs) integrated into the mamba-YOLO framework.
Performance and Evaluation
The model’s performance was evaluated using standard metrics including precision, recall, detection speed, and mean average precision (mAP) at 50 % and 50–90 % thresholds. Compared to Faster R-CNN and several YOLO versions (5s, 6s, 7, and 8), mamba-YOLO demonstrated superior results:
- Precision: 98.89 %
- Recall: 98.79 %
- mAP @ 50 %: 97.86 %
- mAP @ 50–90 %: 89.97 %
Despite a slightly slower detection speed (8.30 ms per image) than some YOLO variants, the system remains suitable for real-time applications. Its compact size (6.10 MB) makes it well-suited for low-power, resource-constrained devices like mobile picking robots. These advantages—low energy use, portability, and long battery life—position the model as a practical solution for smart farming.
The model also performed well under natural lighting, achieving a mAP of 97.43 %. However, its accuracy decreased under poor lighting conditions, such as low light (with shading materials) and excessive light (with LED sources), where shadows and background highlights were sometimes misclassified as immature mushrooms.
Download your PDF copy now!
Looking Ahead
This research demonstrates that mamba-YOLO is an accurate, efficient, and lightweight tool for classifying Shiitake mushrooms, paving the way for its integration into automated harvesting systems. Future work could explore more robust validation methods, larger and more diverse datasets, and adaptive lighting systems to further improve detection reliability.
Alternative image capture techniques, such as hyperspectral or multi-angle imaging, may also enhance performance in visually complex environments. Testing the model on actual picking robots will be key for practical deployment.
References
Qi, K., et al. Detection and classification of Shiitake mushroom fruiting bodies based on Mamba YOLO. Sci Rep 15, 15214 (2025), DOI: 10.1038/s41598-025-00133-z, https://www.nature.com/articles/s41598-025-00133-z