Discovering new drugs is essential for treating diseases caused by antibiotic-resistant pathogens as well as improving treatments for currently untreatable diseases. The use of artificial intelligence (AI) and machine learning methods has increased in various sectors, particularly within the pharmaceutical sector. There have been efforts to adopt AI to boost drug discovery, aiming for a greater quantity of output at a lower cost.
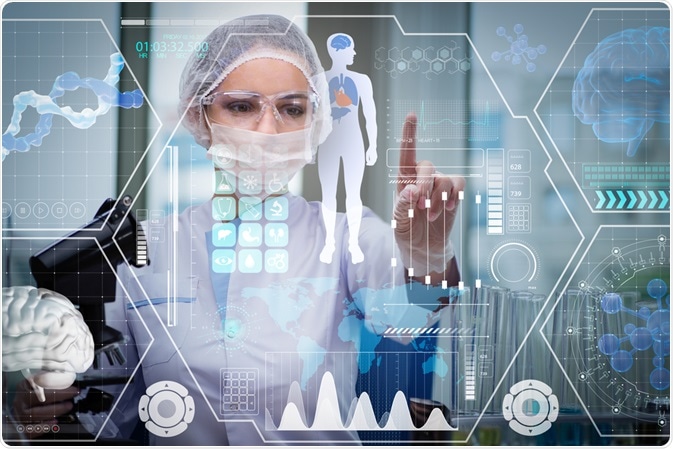
Artificial Intelligence in Drug Discovery Concept. Image Credit: Elnur/Shutterstock.com
What is artificial intelligence (AI)
Artificial intelligence is intelligence demonstrated by machines. AI employs systems that can handle a large volume of data, interpret and learn from the input data and thus make independent decisions to generate specific outputs.
Machine learning can depend on different algorithms, collectively known as artificial neuronal networks (ANN), which comprises of a set of networks analog to the human neuronal network. Multilayer perceptron (MLP) networks are mainly used for pattern recognition; recurrent neural networks (RNNs) for storing information; and convolutional neural networks (CNNs) for image processing, biological system processing as well as sophisticated signal processing.
Current methods in drug discovery
Drug discovery requires the processing of vast information and different algorithms. It is essential to address the following area to develop an effective drug:
- Identification of the drug target
- Physiochemical properties of the drug (e.g. solubility, distribution in the body, and metabolism)
- Cellular toxicity
- Behavior in heterogeneous cell culture/ 3D models
- Animal model efficacy
Traditionally, drugs are discovered by analyzing isolated mechanisms and receptors, by either hypothesis-driven or non-driven phenotypic screening. Computer-aided drug design (CADD), and structure-activity relationship (SAR) analysis (which correlates changes in chemical structure to changes in activity) are used for modeling drug interactions.
However, it is not trivial to translate the effect of one drug candidate compound in higher dimensional readouts, for example, its effect on the whole biological system. In addition, the drug screening process can be time-consuming and expensive.
AI in drug discovery
The chemical space is a dataset of potential pharmacologically active molecules in an order of 1060 molecules. AI can recognize hit and lead compounds in large protein datasets, such as PubChem, ChemBank, DrugBank, and ChemDB, and provide a quicker way for drug validation and drug structure optimization.
This allows AI to contribute to the de novo design of drugs, that is, to design novel structures computationally with the desired properties. Algorithms, such as Nearest-Neighbour classifiers, RF, extreme learning machines, SVMs, and deep neural networks (DNNs) are often used to predict synthesis feasibility as well as substance toxicity and activity in vivo. Once identified as being worthy of validation, the compound can be synthesized for experimental tests. For predicting drug-protein interactions, AIs can be trained on pharmacological and chemical data.
AI can also replace the older “trial-and-error” method of optimizing dosage and preferred way of drug delivery. A computational modeling method - Quantitative structure-activity relationship (QSAR) – can solve issues on stability, dissolution, and porosity.
Future outlook
Not only can AI speed up the drug discovery process, but it can also optimize the production methods of the drug candidate, further reducing the time required to produce a drug for clinical use. Nevertheless, one of the downsides of AI in drug discovery is that each step in drug discovery requires a different modeling algorithm.
There is no integrated, stream-lined program for target identification to ligand-protein activity and properties predictions. Moreover, AI-based methods of drug discovery depend on the chemical space in which the AI is trained to pick out possible compounds. There is a need to access novel chemical spaces.
Nevertheless, the emergence of AI in drug discovery changes the lifecycle of drug design in pharmaceutical companies. And although there are currently no drugs on the market developed using AI-based approaches, it is highly likely that AI will contribute to a large extent in discovering new drugs in the near future.
Sources:
- Bender, A., & Cortés-Ciriano, I. (2020). Artificial intelligence in drug discovery: what is realistic, what are illusions? Part 1: Ways to make an impact, and why we are not there yet. Drug Discovery Today. https://doi.org/10.1016/j.drudis.2020.12.009
- Fleming, N. (2018). How artificial intelligence is changing drug discovery. Nature (London), 557(7707), S55–S57. https://doi.org/10.1038/d41586-018-05267-x
- Paul, D., Sanap, G., Shenoy, S., Kalyane, D., Kalia, K., & Tekade, R. K. (2021). Artificial intelligence in drug discovery and development. Drug discovery today, 26(1), 80–93. https://doi.org/10.1016/j.drudis.2020.10.010
Further Reading