Drug discovery predominantly focuses on targeting and modulating the activity of proteins with known functions or harnessing the ability of a small molecule to impart desired physiological effects. Utilizing the structure of native ligands or molecular targets, small molecules can be developed to selectively bind proteins and regulate biological processes.
A new type of medicine, custom-made with tiny proteins | Christopher Bahl
With typically well-defined binding sites, ligand-protein interactions are often targeted for drug intervention. However, protein-protein interactions (PPIs) are fundamental to modulating many biological processes, with dysregulation often seen in disease states. Though a seemingly attractive drug target, PPIs have been generally regarded as “undruggable.”
Targeting featureless protein interfaces
Understanding the molecular recognition mechanism at PPI interfaces has presented a hurdle to drug development for many years. With large, flexible binding sites containing few grooves or pockets and high affinities for their native protein interaction, these interfaces have proven challenging targets in drug design.
In recent years the therapeutic potential of targeting PPI interfaces has seen a resurgence with the elucidation of “hot spots.” Structure-activity relationship analysis revealed that hot-spot regions in PPI interfaces are responsible for contributing the majority of the binding free energy. Targeting these regions with small molecules could allow for the intervention of PPIs.
By utilizing information about hot-spot regions and the PPI interface, structure-based drug design can aid the development of small molecules with complementary shapes and chemical composition to their target. Combined with computational tools and machine learning algorithms, candidates can be rapidly and efficiently screened for potency in modulating target activity.
Exhibiting high selectivity and efficacy, as well as being relatively safe and well-tolerated, peptide-based therapeutics could help convert these traditionally “undruggable” targets into attractive opportunities for clinical intervention. However, peptides present their own set of challenges.
An alternative to traditional small molecule inhibitors
While small molecules have been successfully developed to inhibit oncoproteins in targeted chemotherapies, only 3000 out of ~25,000 proteins encoded by the human genome possess binding sites suitable for small molecules to target. Small molecules are not viable inhibitors for PPI networks critical to tumorogenesis and metastatic pathways due to the high cellular compartmentalization and the featureless nature of protein interfaces.
Utilizing peptide-based therapeutics to inhibit these PPI interfaces, it has been possible to inhibit key oncogenes such as MYC, HOX, KRAS, BCL-2, and HDM2/HDMX both in vitro and in vivo. Successfully translating into clinical practice, peptide-based therapeutics against BCL-2 and HDM2/HDMX are currently available for the treatment of hematologic and solid malignancies.
Though seemingly offering enormous potential as drug candidates, a significant challenge in developing peptide-based PPI modulators has been the lack of natural ligands available to determine structural requirements. The ability to characterize PPI drug targets and the design of complementary molecules has advanced mainly due to computational methods and deep learning algorithms. Designing virtual 3D structures of molecular targets allows computational tools to support the identification of drug leads from libraries of synthetic compounds and peptides.
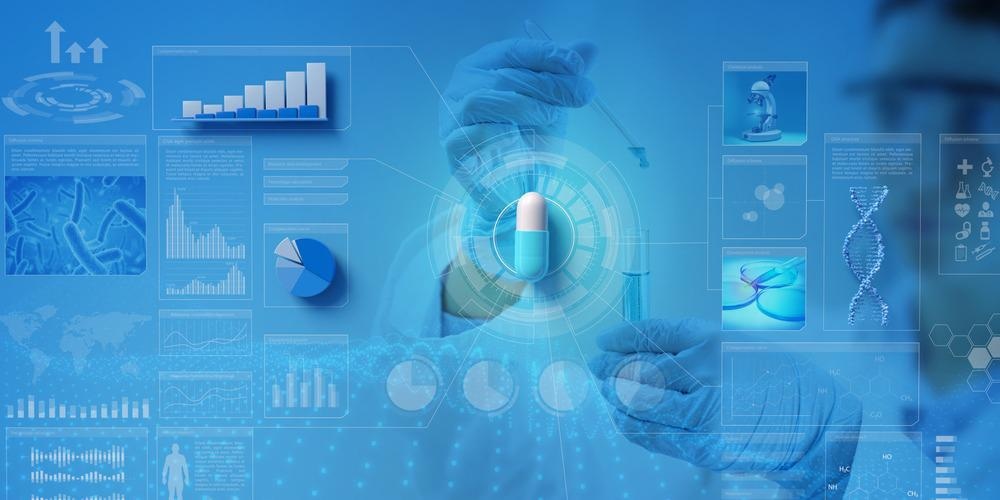
Image Credit: paulista/Shutterstock.com
The intelligent design of peptide-based therapeutics
Deep learning techniques allow for accurately predicting the interactions between drug candidates and molecular targets in silico. To reduce the time and cost of experimental prediction of PPI structure and chemistry, machine learning tools can determine the molecular components required for a drug candidate to bind and modulate its target effectively.
Utilizing fragment-based drug design, virtual screens can also be used to inform the modification and optimization of drug candidates. To improve the oral bioavailability and generally short half-life of peptide-based therapeutics, peptidomimetic design is often employed. Peptidomimetics are divided into 4 classes based on the degree of alteration and difference to the parent peptide, mirroring the pharmacophore of parent peptides.
Peptides with minimal chemical alterations fall into Class A, while Class B mimetics are peptides with non-amino acid additions. Class C mimetics categorize small molecules with key (“hot spot”) residues in the bioactive conformation of the parent peptide. The final group, Class D, encompasses non-peptide small molecules that mimic the function of bioactive peptides.
Improving pharmacokinetic (PK) and pharmacodynamic properties of peptides through modifications guided by computational tools broadens the field of drug development to new horizons. The last century has seen ~60 peptide drugs approved for clinical use in the United States, Europe, and Japan. However, targeting less than 10% of the PPIs in the human interactome, the potential of peptide therapeutics has yet to be fully elucidated. With computer-aided drug development and optimization of peptide-based therapeutics expanding, the potential applications of peptides continues to grow.
Sources:
- Lau, J.L., and Dunn M.K. (2018) Therapeutic peptides: Historical perspectives, current development trends, and future directions. Bioorg Med Chem. doi: 10.1016/j.bmc.2017.06.052.
- Mabonga, L., and Kappo, A.P. (2020) Peptidomimetics: A Synthetic Tool for Inhibiting Protein–Protein Interactions in Cancer. Int J Pept Res Ther. https://doi.org/10.1007/s10989-019-09831-5.
- Wu, K. J., et al. (2019). Mimicking Strategy for Protein-Protein Interaction Inhibitor Discovery by Virtual Screening. Molecules. https://doi.org/10.3390/molecules24244428
- Farhadi, T., and Hashemian, S.M. (2018). Computer-aided design of amino acid-based therapeutics: a review. Drug design, development and therapy. https://doi.org/10.2147/DDDT.S159767
- Shin, W.H., Christoffer, C.W., and Kihara, D. (2017). In silico structure-based approaches to discover protein-protein interaction-targeting drugs. Methods. https://doi.org/10.1016/j.ymeth.2017.08.006
- Lu, H., et al. Recent advances in the development of protein–protein interactions modulators: mechanisms and clinical trials. Sig Transduct Target Ther 5, 213 (2020). https://doi.org/10.1038/s41392-020-00315-3
- Fosgerau K, Hoffmann T. (2015) Peptide therapeutics: current status and future directions. Drug Discov Today. doi: 10.1016/j.drudis.2014.10.003.
- Sorolla, A., et al. (2020). Precision medicine by designer interference peptides: applications in oncology and molecular therapeutics. Oncogene https://doi.org/10.1038/s41388-019-1056-3
Further Reading