It is vital to optimize the drug delivery process because it potentiates the therapeutic effect of medicinal drugs. An optimized drug delivery method augments the physicochemical features of the drug by overcoming the inherent instability of bioactive compounds, which damages its pharmacokinetic properties. Recently, scientists have utilized artificial intelligence (AI) to design, characterize and optimize drug delivery.
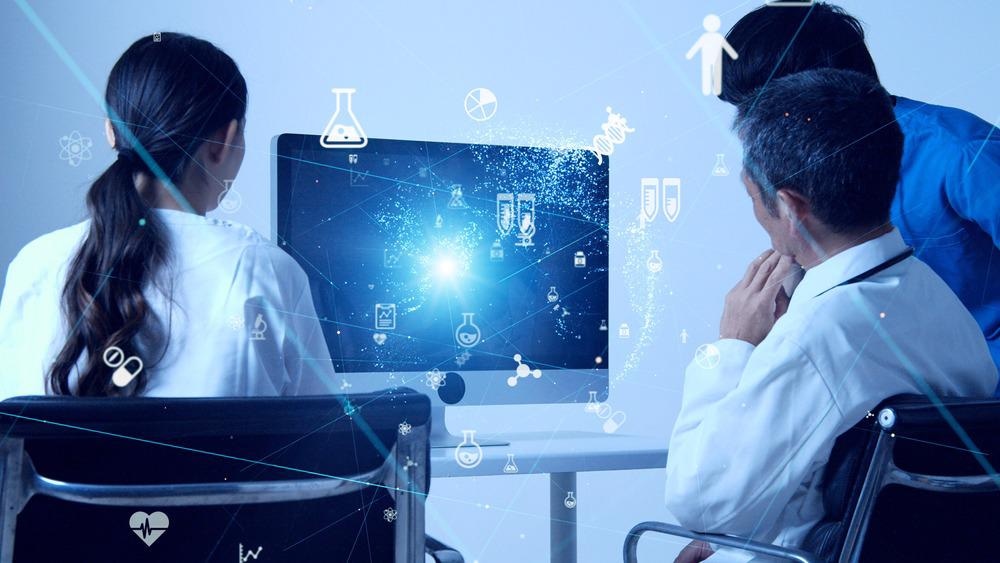
Image Credit: metamorworks/Shutterstock.com
Drug delivery involves various approaches that include formulations, manufacturing techniques, storage of bioactive compounds, and transportation to the target sites to achieve a maximum therapeutic effect. Recently, drug delivery has been strongly associated with nanotechnology-based innovations. Although scientists applied various fields of sciences, such as pharmaceutical sciences, biosciences, and engineering, to optimize drug delivery techniques, the evolution of computational methods has accelerated the entire process. Researchers use AI to design, characterize and manufacture drug delivery nanosystems.
In the last decade, AI has been applied for analyzing and interpreting biological and genetic information. It has not only fast-tracked the process of drug discovery but also enabled the rapid identification of functional small molecules and the prediction of their behavior.
How does Artificial Intelligence Work?
Over the last few years, rapid advancements in data digitalization in the pharmaceutical sector have occurred. This has resulted in the documentation of an enormous amount of data, which requires proper processing and scrutinizing to be applied in experimental research. AI can tackle enormous amounts of data with enhanced automation. It has become a promising tool for achieving reverse engineering and continuous optimization of drug delivery systems. Applications of AI have significantly improved treatment outcomes.
AI is a technology-based system that comprises various advanced tools and networks that recapitulate human intelligence. AI can make independent decisions for a specific objective by utilizing software and systems that can learn, reason, and interpret the input data. AI uses many method domains, such as a fundamental machine learning paradigm (ML). ML uses algorithms to identify patterns within a set of input data. Deep learning is a subcategory of ML, which engages artificial neural networks (ANNs) and mimics the transmission of electrical impulses in the human brain.
Scientists have developed various tools based on the network that forms the core architecture of AI technology. One of the tools based on the AI system is International Business Machine (IBM) Watson supercomputer, which has access to patients’ medical records and can correlate it to a vast database to detect abnormalities or diseases. For instance, IBM can detect breast cancer in only 60 seconds.
Artificial Intelligence and Drug Delivery
A vast chemical space comprises more than 1060 molecules that could be used as a potential drug. Researchers use AI to identify lead compounds with pharmaceutical potential against a disease, facilitate fast drug target validation, and optimize the drug delivery process. This article mainly focuses on the optimization of drug delivery through AI.
Nanorobots consist of integrated circuits, power supply, sensors, and secure backup of data maintained via AI-based technologies. These nanorobots are used in drug delivery. They are programmed to avoid a collision, identify the target, get attached to the target, deliver the drug compounds, and finally, come out of the body.
Advanced microrobots and nanorobots can navigate the targeted sites based on physiological conditions (e.g., pH). This method improves the efficacy of drugs and reduces systemic adverse effects. Researchers have developed implantable nanorobots that can deliver drugs in a controlled and dose-dependent manner.
Scientists used ANNs, logistic regression, and network-based modeling to screen drug combinations and improve the overall dose regimen. Often, combination drug therapy, where multiple drugs need to be delivered at a precise time, is a more effective treatment than a single drug. For instance, cancer treatment requires six or seven drugs as a combination therapy.
Researchers have also developed a quadratic phenotype optimization platform to identify optimal combination therapy to treat bortezomib-resistant multiple myeloma. An efficient combination of drug delivery could be achieved by analyzing data on the synergism or antagonism of drugs when administered together. Scientists used the Master Regulator Inference Algorithm, which utilizes ‘Mater regulator genes,’ to predict 56% synergism efficiently.
Additionally, Network-based Laplacian is another platform used to control synergistic drug combinations used for efficient drug delivery. These AI-based models could predict twenty-eight synergistic anticancer combinations. Also, Combination Synergy Estimation has been designed to predict potential synergistic antimalarial combinations.
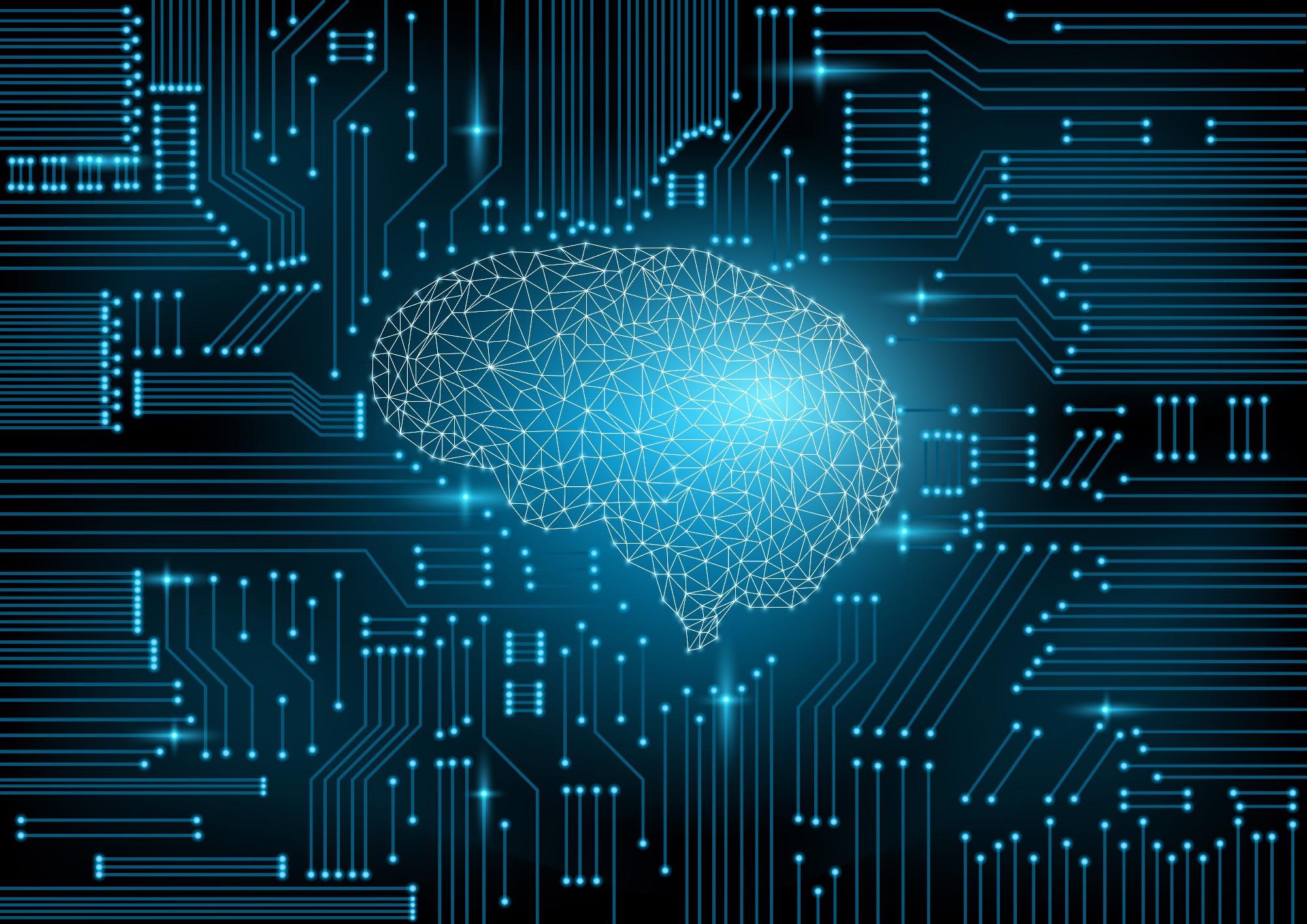
Image Credit: CNStock/Shutterstock.com
Challenges Faced by AI-based Technology
One of the challenges AI-based systems face is the diversity and uncertainty of data sets. Data sets available for drug development in pharma companies include millions of compounds, and traditional ML tools cannot process such types of data.
Although the quantitative structure-activity relationship (QSAR)-based computational model overcomes some limitations and can predict many compounds, it also faces some difficulties. Some limitations include small training sets, faulty experimental data in training sets, and lack of experimental validation.
Sources:
- Paul, D. et al. (2021) Artificial intelligence in drug discovery and development. Drug discovery today. 26(1). pp. 80–93. https://doi.org/10.1016/j.drudis.2020.10.010
- Using AI to accelerate drug discovery. (2020) [Online] Available at: https://www.nature.com/articles/d42473-020-00354-y
- Colombo, S. (2020) Applications of artificial intelligence in drug delivery and pharmaceutical development. Artificial Intelligence in Healthcare. pp. 85-116. https://doi.org/10.1016/B978-0-12-818438-7.00004-6
- Hassanzadeh, P. (2019) The significance of artificial intelligence in drug delivery system design. Advanced Drug Delivery Reviews. 151-152. pp. 169-190. https://doi.org/10.1016/j.addr.2019.05.001
- Fleming, N. (2018) How artificial intelligence is changing drug discovery. Nature. 557. S55-S57. doi: https://doi.org/10.1038/d41586-018-05267-x
Further Reading