In this interview, we speak to TetraScience Chief Scientific Officer (CSO) Mike Tarselli about how the Tetra Data Platform (TDP) enables scientific data integration and analysis, as well as what the future looks like for AI within the life sciences.
Please introduce yourself: What’s your role at TetraScience? What do you do?
I’m Mike Tarselli, the CSO for TetraScience. In my role as CSO, I look at our scientific strategy, address our customers’ needs, and help them understand best practices in the scientific data cloud space. Internally, I lead our scientific integrations – a fast-growing team of scientific technologists who work with customers to match product capabilities against important scientific use cases and challenging life sciences workflows.
We also work with the Tetra Partner Network (TPN) to ensure our products integrate seamlessly with the broadest range of instruments, informatics platforms, analytics tools, and other data sources and targets. Additionally, I manage the GxP compliance team to ensure we stay aligned with regulatory challenges in biopharma and tech. I also support our content and communications team: for example, with podcasts, white papers, blog posts, and articles such as this one you’re reading now.
Finally, I help to recruit the next generation of Tetra science + tech “cyborgs,” through interviewing, training, and mentorship.
TetraScience is the world's first and only open scientific data cloud. Can you tell us more about TetraScience and its mission and goals?
I wholeheartedly believe in and subscribe to our vision — solving the grand challenges of humanity – including improving and accelerating life-changing therapies. We work to improve human health through the lens of scientific data. We firmly believe biopharma companies could operate more effectively if they could use both the trove of data they already have, and the 2-3x explosion of new data they expect to acquire this year, alone – a rate that's only expected to increase in coming years.
The Massive Scientific Data Problem
Data scale increases exponentially. How can biopharma scientists, data scientists, and IT make sense of it all? Hiring can't keep pace with that 2-3x annual data growth. Nor does the industry yet have well-trained and refined artificial intelligence / machine learning (AI/ML) tackling the problems and key opportunities this data underlies. And this is because the data itself is buried in silos, sunk in proprietary file formats, full of gaps, and isn't FAIR (Findable, Accessible, Interoperable, Reusable).
How will organizations solve this massive challenge? How will they contextualize and standardize all the data received? How does the business ensure that everyone who needs access to data is given appropriate privileges? How will biopharma ensure that data is harmonized, so its meaning is preserved, and it remains understandable and usable 20 years into the future?
And the Massive Scientific Data Opportunity…
TetraScience helps organizations address these challenges by taking the data that all biopharma organizations have in their laboratories — data from diverse scientific instrumentation, sensors, and informatics software — and bringing it into a vendor-neutral scientific cloud platform. We archive data to ensure original artifacts can always be found and accessed, and so that users can understand its provenance and lineage. Raw data are then opened up, parsed, and extracted.
Then metadata are indexed and appended. Who ran a particular experiment when and where? What did they aim to do? Which compound or sample IDs were consumed and generated? (Along with many other potential decorations up- and downstream.) Customers find this “contextualized information” hugely valuable. It establishes data's meaning. It provides an audit trail enabling compliance. It makes the data that scientists need easy to find, using simple search strategies. And context helps preserve data's value over the long term – even, potentially, the value you might not suspect exists, today — because it's still awaiting discovery by coming generations of AI/ML.
Finally, we transform and harmonize the data into this beautiful hierarchical data model that allows users to open up their analytics tool of choice and draw up a curve, regardless of where the data came from. Harmonization makes the data FAIR – in-house, we say it's both 'liquid' (it flows) and 'actionable' (you can use it to drive decisions).
Tetra Data Overview Video
Despite the drug discovery field making advancements in recent years, challenges persist. What challenges does the drug discovery sector face today, and how does the Tetra Data Platform help to tackle them?
Let’s start with the largest challenge - 95% of therapeutic candidates that go into the pharma “funnel” don’t make it to patients. By making scientific data FAIR, we help biopharma apply many different kinds of scientific, instrumentation, software, and process improvements to confront aspects of this challenge and optimize drug development.
FAIR data helps labs automate: moving data liquidly across our platform from increasingly-roboticized, scaled-up instrument clusters to ELNs and LIMS to analytics to lab management and sample management systems. Science therefore accelerates: speeding up the iterative discover-measure-test-analyze (DMTA) loop of scientific work, and leveraging automation to do more work in parallel with high-throughput screening and other scalable technology. The result can be faster convergence on therapeutic candidates of interest, faster identification and elimination of marginal candidates before clinical trial, and ability to manage more projects in pipeline, all measurably increasing chances of success while avoiding unprofitable expenditure.
Meanwhile, automation enabled by FAIR data returns a great deal of productive time to scientists and data scientists (see our survey) and helps scientists focus on science, instead of manual data collection, transcription, validation, and other error-prone toil. Overall, we believe the time saved by our technology will get effective therapeutics to market faster, improving our customers' competitive outlook, and increasing patent lifetime and market exclusivity.
How does the Tetra Data Platform Work?
The Tetra Data Platform (TDP) performs two main functions. Firstly, it ingests data onto the cloud through a Tetra Integration. You cannot make the data “dance” until it lands on a unified platform.
Next up, TDP contextualizes and harmonizes the data. It puts the data into a consistent hierarchical schema - Intermediate Data Schema, or IDS - which allows the user to figure out what data they have actually got. Users can readily inspect metadata and compare data sets across multiple vendors across instrumentation like HPLC, cell counters, flow cytometers, mass specs, or plate readers.
Often, we can use RESTful APIs to communicate with instruments, software, and sensors directly over the internet. However, many older control software systems require a software agent — a small Windows application that “talks'' to that software to unpack the data in a comprehensible format and send it to TDP. If you need to support non-networked instruments, like balances or bioanalyzers, we support "internet of things” (IoT) devices and agents that work together to stream this data to the cloud, at intervals. No matter what kind of data you are going to give us, we have a way to understand and harmonize it. Our platform is built on top of Amazon Web Services. Raw data is stored there. Parsers then begin to unpack the data, and software pipelines then act upon the data to harmonize it.
The technicalities depend on your use cases, short-term, and longer-term goals. As the CSO, I am always wanting to solve the grand challenges. But it’s equally important to know the “nuts and bolts” of how technical integrations work, and what use-cases customers need to serve, today. The nice thing is that, once a customer has access to our platform and successfully realizes one or more use-cases — often with a relatively small effort – other applications suggest themselves, and scaling out to support new instruments, software, and workflows becomes progressively easier and more predictable.
What should life sciences organizations think about if they seek to replatform to the Cloud?
For cloud-based software, if you want interoperability across multiple platforms and you want to reuse this data in five or ten years, don’t choose physical storage media. We should not constrain data to hardware that will be obsolete in a few years.
I have walked through laboratories where a Windows 98-powered beige PC was the most recent version qualified to store data from a specific laboratory instrument. In 2018! This outmoded tech relies upon a person who must be the SME (subject matter expert), facilitating the data interaction between that system and the rest of the data corpus in the company.
By contrast, in other sectors – finance, retail, even government – data now mostly flows liquidly from sources to databases to target applications and back. These sectors rely on automation, security protocols, and testing to prevent issues, while still letting data flow easily and enabling rapid innovation.
Back in biopharma, as noted earlier, scientists today are relegated to serving as human data buses — spending 50 to 60% of their energy moving data into text files, opening it up in Excel, or graphing things by hand. Those highly-qualified scientists could be putting their time and energy into much more important tasks — like actual scientific research and analysis!
Of course, we have empathy for those who started their careers before the Cloud was omnipresent. It can be daunting to place your life’s work on remote resources you can’t see or touch. Thus, raising awareness about the massive advantages Cloud can bring, how much easier it can make research; and streamlining the process of replatforming itself, are critical.
As more and more tech-savvy and cloud-savvy people come into science, we are seeing this shift happen. People who grew up with and/or understand this technology know it can help them move faster and work better.
There is a cultural stereotype of the scientist drilling away at her laboratory bench, thinking giant thoughts, scribing things on reams of paper, shouting "Eureka!" as flames and lightning bolts appear. But this just isn't the way science is done, anymore. Science is now team-based and very much a global effort.
For example, a team in Hyderabad will start a project, a team in Boston will pick it up, and then a team in Singapore will run assays before the first group wakes up the next day. This cannot be achieved if the data lives on-prem or on a single computer, or is exchanged using paper, .pdfs, or PowerPoint slides with embedded charts. High-efficiency global collaboration can only be achieved if data is universally comprehensible, easily shared across the Internet, and travels with context.
Today, scientists are repeatedly faced with what start to feel like very dumb problems involving data. For example, it's very difficult in pharma to find all the data that a colleague has ever generated or worked with for a given project or instrument – thus making it very difficult to conduct research that builds upon that colleague's work. Scientists seeking to do so are obliged to search through multiple systems, ancient files, and, in certain cases, peruse paper documents, just to find everything that a colleague did over a span of time. This could take weeks, compared with the fast approach of just clicking a button and asking for data that's tagged on a cloud to be sent back.
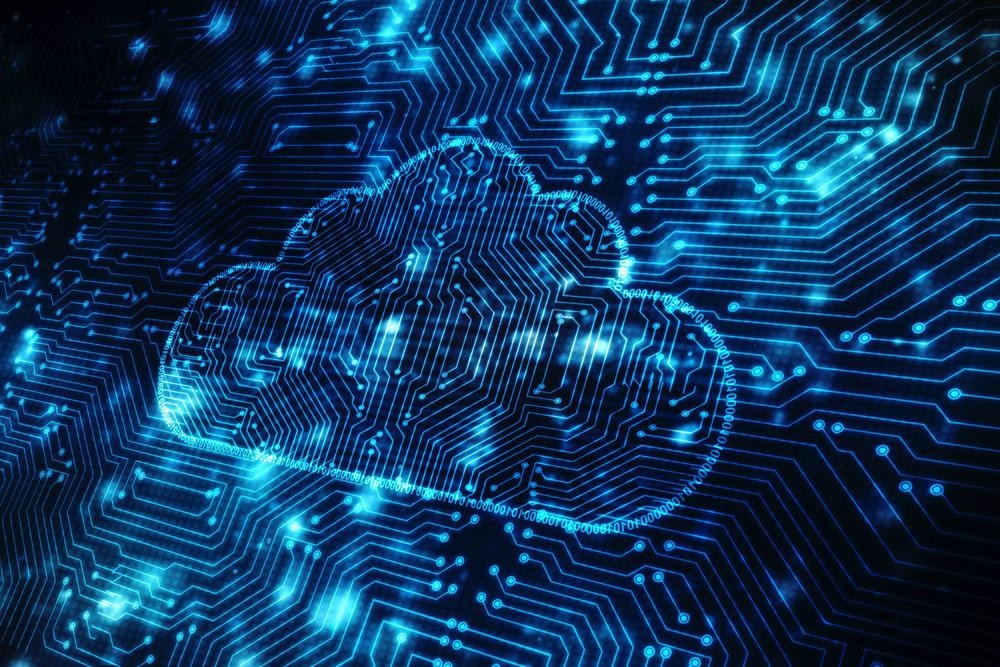
Image Credit: Blackboard/Shutterstock.com
TDP speeds up labor-intensive tasks, allowing researchers to refocus on new discoveries and innovations. How important is innovation to life sciences?
I don't know if it’s in my remit, specifically as a representative of a technology company, to talk about innovation in the life sciences, writ large. I will speak to the fact that on the data side, if you do not have accessible, harmonized data, you cannot benefit from machine learning or AI – clearly powerful tools for innovation. If you do not have cleaned, schematized, FAIR data, then you certainly should not set up an automation core.
Instead, lacking arranged data, you need to maintain manual, slow, error-prone human-driven processes, because automation can't work without orderly inputs. By contrast, orderly, arranged data creates opportunities for automation. The state of data reflects the nature of how laboratories work, and what a laboratory is. In the pharma of the 1980s and 1990s, processes were still largely test tube- and flask-based, and rodent-based assays would generate readouts into Excel charts used for data recording and analysis. The modern lab has evolved.
Radical shifts have occurred in lab architecture and scientific team structure over the past decade. Laboratories today, both because of the COVID-19 pandemic and due to globalization, miniaturization, and technology, are much more roboticized and automated. Data-intensive, data-driven processes on single cells and complex libraries increase throughput. No longer do dozens of busy scientists mill about; it’s more like 7-10 scientists using instrumentation, conducting experiments at the bench, and facilitating large automation runs. A supporting cast of “dry-lab” professionals – data scientists, automation engineers, IT project managers, molecular modelers, and many others – operate in parallel to support the research and manufacturing efforts, assisting across the pharma value lifecycle. We need to help people realize this shift in working norms and how cloud replatforming facilitates it.
What's next for TetraScience? Are there any exciting projects that you were involved in?
I first joined TetraScience back in August of 2020, and it’s amazing to think about how fast the company has grown; we’re on a rocket ship! We have a long funding runway and plenty of juicy scientific challenges ahead. We certainly face the growing pains of an evolving company, as any start-up would. When you scale a company 8x in human capital, and revenue increases 30x in two years, you have boundless opportunity but a fair amount of cultural scale and process-building ongoing.
So which opportunities should we take on, and which opportunities should we reject? Right now, we’re deliberately and narrowly focused on biopharma use cases. Our early solutions improved analytical chemistry, late-stage development, assay creation, and bioprocessing, but more scientific domains have reached out. Cell and gene therapy, new modalities, RNA therapeutics, chemical Design of Experiments (DoE), QC/QA, and manufacturing - we’ll be quite busy for the foreseeable future.
How much of the biopharma life cycle could we improve? We could get into high-throughput and high-content screening, and the very early stages of automation. Later on, we could also go into clinical, manufacturing, and maybe even into data formation (AI/ML). Other industries possess a “pharma-like” model of research, development, and production and we are of course looking at those as potential ways to use our solution.
The COVID-19 pandemic was a terrible, staunch challenge for the entire world. That said, as a silver lining, the pandemic accelerated in the adoption of scalable tools like cloud platforms and data automation, - which are essential to get ahead of the next pandemic if and when the time comes.
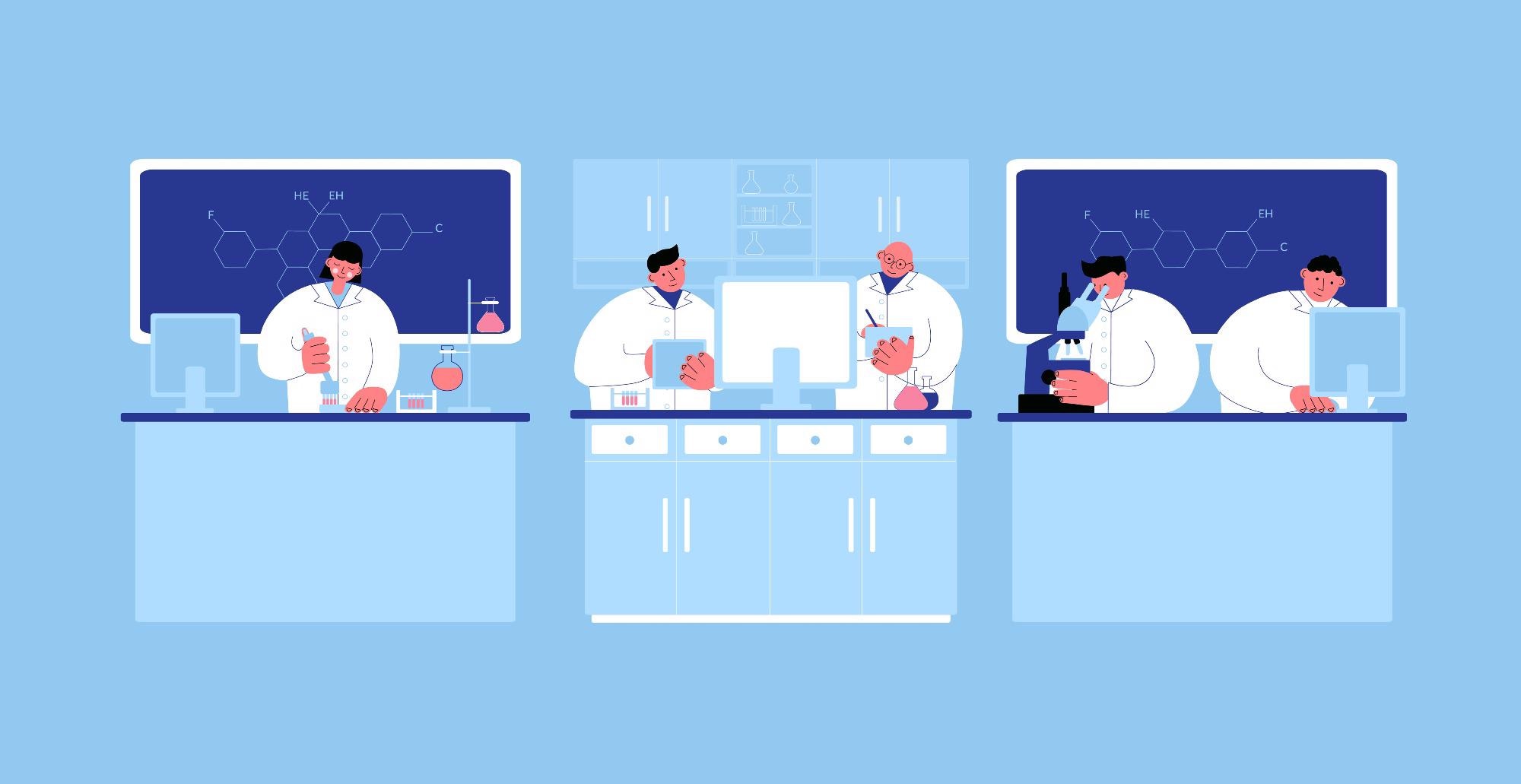
Image Credit: Sabina Palm/Shutterstock.com
What do you believe the future of drug discovery looks like within the life science industries? Are there any trends you particularly foresee?
People often compare drug discovery to “art” instead of science. It’s a long process: you have to understand the nuances of a given disease state, patient variability, surprising or seemingly paradoxical results. You really have to walk the journey.
It is quite common, nowadays, that if you go into pharmaceuticals right out of school, you’ll realize — if you are very lucky — at most two marketed, commercialized drugs over your career. Drug discovery is a huge investment of time, money, and dealing with failures.
I believe that drug research will change to resemble parallel industries. We’ll see more streamlining like in the food production or petroleum industry — large conglomerates will centralize manufacturing and standardize on specific quality processes. More difficult scientific research will be reserved for rare diseases, for example.
The second point: we're in the absolute infancy of data science, AI, and machine learning. Machine learning can currently be used to detect your face on an iPhone, to have your vacuum walk across the floor, to have a car look for road signs and people. Now compare this to human biology: you’ll have to train models using every molecule / protein interaction that might occur. It’s orders of magnitude different.
We are not anywhere near where we need to be on the AI and machine learning front. I’ll probably be near retirement by the time we finally have intelligent agents recommending targeted medication regimens to specific patients.
But that’s the dream. People want intelligent machines that can look at all this data while we’re asleep, tell us what the best drug is to make for a particular condition, and explain the reasons why, or make predictions about the assays. We aren't there yet, but that's what's coming. I can’t wait to see how TetraScience - and biopharma worldwide - grows and changes in the next 5 years.
About Mike Tarselli
Mike Tarselli, Ph.D., MBA is the Chief Scientific Officer for TetraScience, a Boston-based start-up building the life sciences R&D Data Cloud. In this capacity, he handles data integrations, use case research, GxP compliance, and internal training. Previous roles include SLAS, Novartis, Millennium, ARIAD, and Biomedisyn. Mike has been recognized by IUPAC, Wikipedia, ACS, and the Burroughs-Wellcome Trust. His volunteer roles promote scientific education and diversity at the National Science Foundation, the Pistoia Alliance, and the UMass College of Natural Sciences Dean's advisory board. 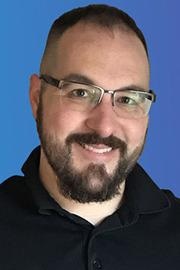
ORCID: https://orcid.org/0000-0003-1285-3134
LinkedIn: https://www.linkedin.com/in/tarselli/
Mike's research interests include FAIR data, scientific futurism, natural products, and synthetic biology.
About TetraScience
TetraScience is the Scientific Data Cloud company with a mission to transform life sciences, accelerate discovery, and improve and extend human life. The Tetra Scientific Data Cloud provides life sciences companies with the flexibility, scalability, and data-centric capabilities to enable easy access to centralized, harmonized, and actionable scientific data and is actively deployed across enterprise pharma and biotech organizations.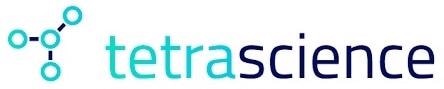
As an open platform, TetraScience has built the largest integration network of lab instruments, informatics applications, CRO/CDMOs, analytics, and data science partners, creating seamless interoperability and an innovation feedback loop that will drive the future of life sciences