Discovering new medicines is a long and complicated process that normally requires several years and huge investments. A lot of research in drug discovery is currently focusing on new approaches to synthesize new molecules together with reducing efforts and costs.
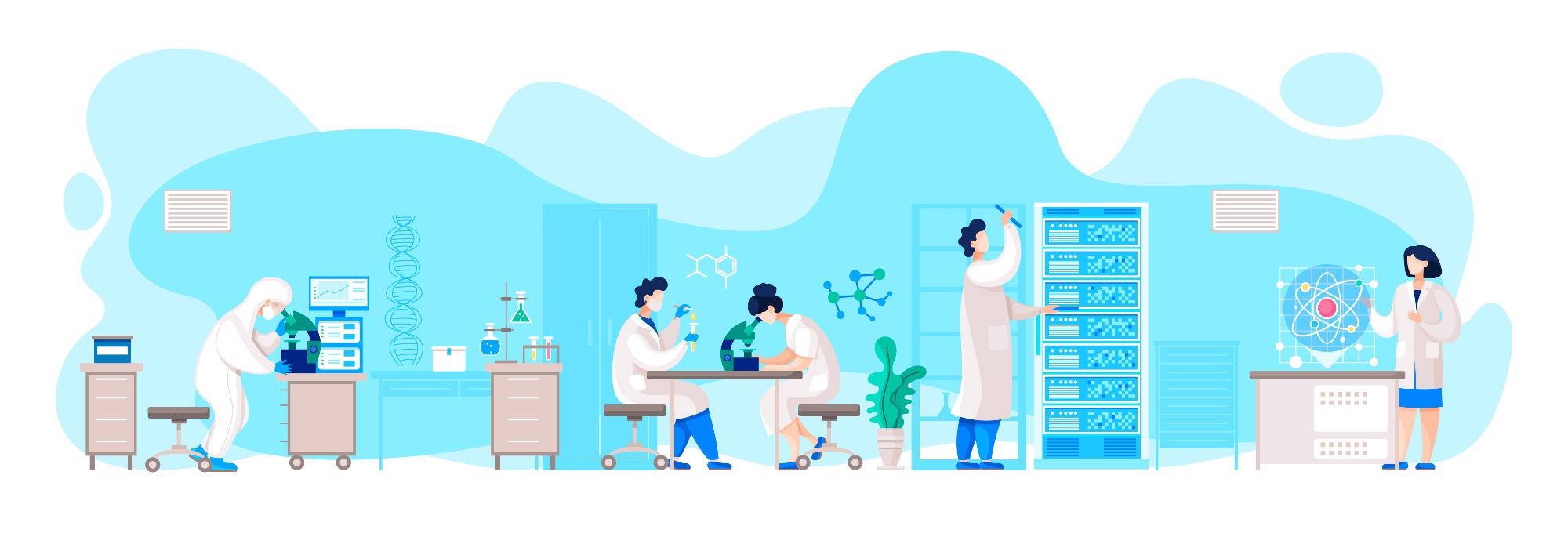
Image Credit: topvector/Shutterstock.com
From target identification to approvals, developing a new medicine can take around 12 years or more, as well as considerable investments, often in the excess of two million dollars. The strategy behind the drug development process is “fail fast, fail early”, with the aim to eliminate risks of failure before making large investments in the late stages.
At the basis of conventional drug discovery, there are two key separated steps: the generation of chemical libraries, where new molecules (potential leads) are synthesized, and the investigation of their biochemical properties through bioassays, via high throughput screening.
Both steps can be iterated and optimized to find the best candidates. There is however a strong need to find more efficient methods, which can save time, money, and chemical resources.
Fragment-Based Methods to Assemble New Molecules
Fragment-based drug discovery is experiencing increasing popularity. First introduced in the mid-1990, it is seen as an alternative to conventional methods for the development of new medicines, since it can provide potential drug leads with greater ligand efficiency.
This approach is based on the molecular recognition of small molecules (fragments) by a biological target, typically a protein. Such fragments are chemically reactive, and reactions on the protein surface accelerate the formation of those ligands with increased binding affinities and biological activities.
From a thermodynamic viewpoint, small contributions from the molecular fragments contribute to the free binding energy of the target molecule, yielding to high-affinity ligands.
A great advantage of the fragment-based approaches is the need for small libraries (<1000 molecules). The starting fragments can then be modified to generate a binder of higher affinity, which is subsequently further optimized to a lead structure.
There are several strategies for fragment-based drug discovery that have been proposed over the years, but all of them use a target protein as a template for the assembly of the new ligands that can then the optimized to develop new potential medicines.
Finding New Ligands via Dynamic Combinatorial Chemistry
Dynamic Combinatorial Chemistry (DCC) – first introduced towards the end of the 1990s – allows for the generation of libraries of molecules where the constituents assemble and interchange via reversible chemical reactions.
Despite a multitude of possible combinations, the presence of a template, such as a protein, drives the formation of the constituents with the best binding properties. In principle, this approach can considerably accelerate the identification of new molecules for drug discovery.
To generate a dynamic combinatorial library (DCL), the fragments used must have functional groups that undergo reversible exchange. Different reversible reactions can be employed, such as acetal, boronic ester, disulfide, and imine formation.
Fragments must also have functional complementarity with the target to interact with the binding site and must have suitable geometrical features to achieve optimal binding.
To highlight the potential of DCC in drug discovery, a dynamic library using nine hydrazides and one bisaldehyde was generated to identify inhibitors for endothiapepsin – an aspartic acid protease. Out of 90 different combinations (78 bisacylhydrazones and 13 monoacylhydrazones) six ligands were obtained in higher yields. Two of these ligands showed inhibitory activity, with one being 240 times more potent than the starting fragments.
Tethering is another specific form of DCC, which involves the reversible disulfide exchange on the surface of a protein. The reaction takes place between a small molecule containing a disulfide and a protein with a native cysteine residue, or in the alternative, with engineered residues near the site of interest.
This approach can be used to improve the binding affinities of existing molecules, for example in the development of inhibitors of cytokine interleukin-2 (IL-2) – a critical component in the immune response – or to assemble new high-affinity molecules towards new targets, such as Caspase-3, an enzyme thought to be a target of intervention in Alzheimer’s and Parkinson’s disease.
One of the advantages of tethering is the ability to focus on a particular region of the template. However, it is often necessary to produce a disulfide library with products that generally are not commercially available.
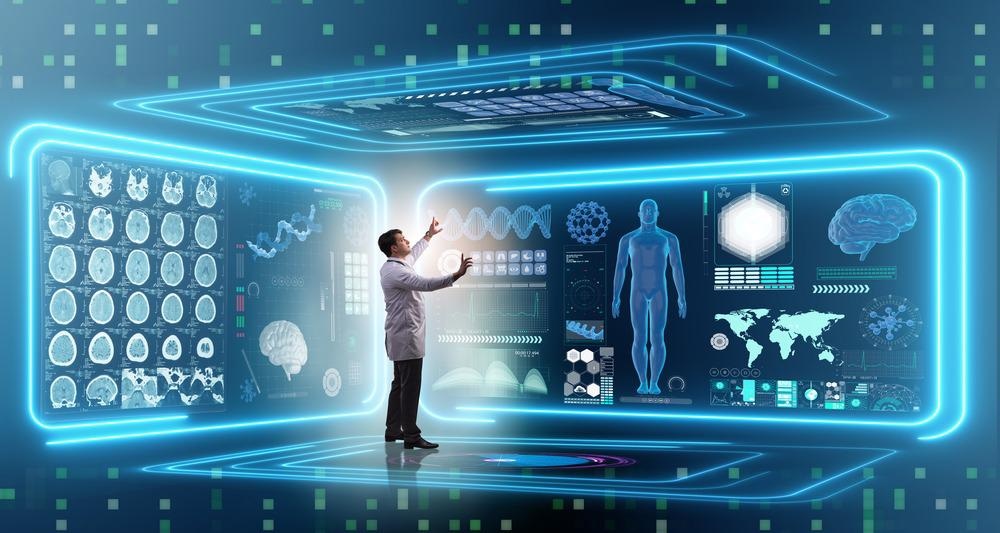
Image Credit: Elnur/Shutterstock.com
Searching for New Drugs with Artificial Intelligence
Due to the great challenges associated with developing new medicines, many efforts are also directed towards using artificial intelligence, particularly machine learning methods, to assist the drug discovery process in identifying new promising molecules.
Since AI and machine learning rely on algorithms to be trained, the main limitations of these methods resides in the scientists’ knowledge of how molecules interact with the target site. Most of the studies are focusing on designing molecules that can safely and effectively work on a well-known protein associated with a disease.
For instance, recently, a machine-learning program was trained to find patterns in data that correlate with cytochrome P450 (CYP450) inhibition, which is one of the main causes of failure of potential drug leads. The results can help researchers rule out quickly those molecules that are potentially toxic and focus instead on candidates that have better chances at progressing through human clinical trials.
The need to find better ways to improve the drug discovery process has prompted the development of new methods for assembling novel molecules. Despite showing great potential, there is still a lot of research needed in order to achieve concrete results and translate initial studies into clinical applications.
Sources:
- Schmidt, M. F. & Rademann, J. (2009). Dynamic template-assisted strategies in fragment-based drug discovery. Trends Biotechnol, 27, 512-21.10.1016/j.tibtech.2009.06.001
- Jaegle, M., Wong, E. L., Tauber, C., Nawrotzky, E., Arkona, C. & Rademann, J. (2017). Protein-Templated Fragment Ligations-From Molecular Recognition to Drug Discovery. Angew Chem Int Ed Engl, 56, 7358-7378.10.1002/anie.201610372
- Erlanson, D. A., Wells, J. A. & Braisted, A. C. (2004). Tethering: fragment-based drug discovery. Annu Rev Biophys Biomol Struct, 33, 199-223.10.1146/annurev.biophys.33.110502.140409
- Freedman, D. H. (2019). Hunting for New Drugs with AI. Nature, 576, S49-s53.10.1038/d41586-019-03846-0
Further Reading