Deep learning is one of the leading machine learning methods that train computers to do what comes naturally to humans. The deep learning technique has many applications; for instance, this technology has been used for creating driverless cars, where deep learning enables the detection of a stop sign or distinguishing a pedestrian from a lamp post. Deep learning concepts have been combined with neuroscience theory to predict nervous system function and uncover general principles.
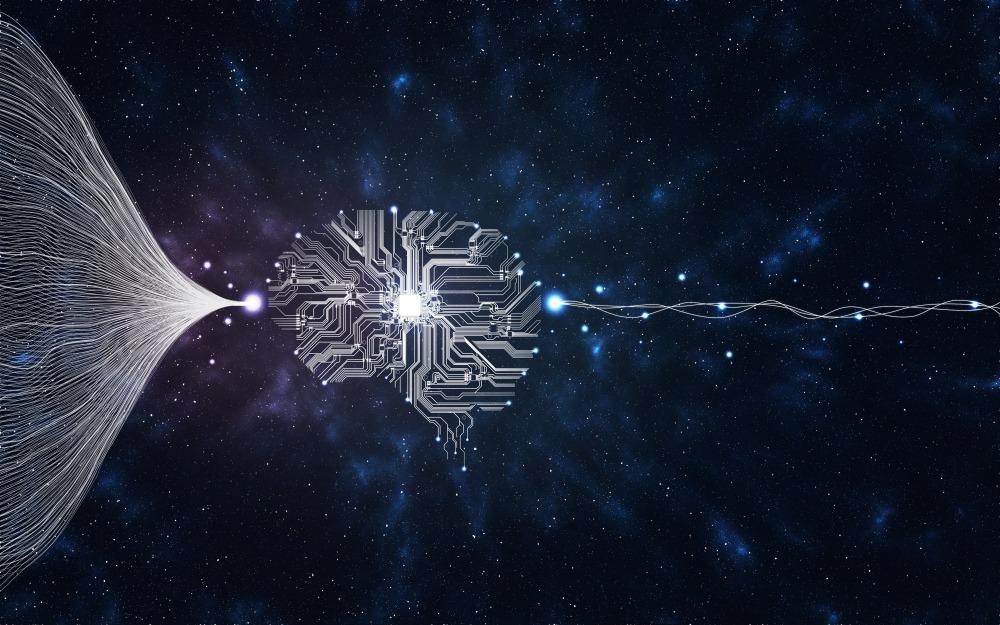
Image Credit: cono0430/Shutterstock.com
Scientists have stated that a revolution in machine learning will benefit neuroscience immensely. Technological advances have enabled the manipulation of the brain at a large scale and quantifying complex behaviors. Data from deep learning techniques are used to develop models of the brain.
Classical Frameworks of Neuroscience
Initially, when the classical frameworks for systems neuroscience were developed, scientists could only record small sets of neurons. This enabled the study of neural activity, the development of the theory of the function of a single neuron, and a circuit-level theory of how the neurons combine their operations. This method performs well for simple computations, for example, to study how central pattern generators regulate rhythmic movements and how the retina computes motion. However, the classical computation system faces challenges while scaling up recordings of thousands of neurons and analyzing all the functions of neurons.
Classical systems did not aid in elucidating neocortex or hippocampus functions. The shortcomings in the classical system urged scientists to develop a new strategy that could take advantage of the experimental advances. This has led to the development of a framework based on the interactions between neuroscience and Artificial Intelligence (AI).
The emergence and rapid progress of deep learning enabled scientists to utilize Artificial Neural Networks (ANNs). Researchers have explained that ANNs model neural computation is based on basic units that mimic real neurons' integration and activation properties. Notably, the specific computations conducted via ANNs are not created but learned.
Deep Learning and Computational Neuroscience
Scientists have been extremely intrigued by the potential of deep learning for neuroscience. Several studies have reflected upon the exciting applications of deep learning in image, video, and speech processing. Researchers anticipate that there will be rapid growth in the number of computational neuroscientists in the future. To date, most of the applications of deep learning in computational neuroscience have been associated with understanding the visual system.
In this context, the study of hierarchical convolutional neural networks has successfully predicted neural responses in several layers of the primate visual cortex, including V1, V2, V4, and inferior temporal cortex (IT). Some scientists stated that deep learning is associated with reusing the ideas of ANN.
A deep ANN possesses multiple layers that feed-forward or are recurrent over time. These layers resemble brain regions instead of being analogous to an individual lamina of a real brain. Deep learning involves training hierarchical ANNs in an end-to-end manner. Recently, advancements in deep learning have been associated with applying bigger ANNs and training with bigger datasets using Graphics Processing Units (GPUs). These developments have solved many problems, such as language processing and translation, image and speech classification and generation, haptics and grasping, sensory prediction, navigation, reasoning, and playing games.
Scientists have observed that this success is closely linked to the fact that convolutional neural networks closely mimic the overall architecture of the cortex. In this system, scientists approached the method of teaching a multilayer network, one at a time. Typically, deep learning networks have many more layers than the corresponding real brain system.
Current "very deep" models contain tens of layers. In deep learning methods, residual networks are used as shortcuts to directly connect units in the lower layers with those in the higher layers.
Deep Learning and the Brain
Several studies have indicated that deep learning can help understand the theories of the brain. Scientists revealed that ANN models of the brain help analyze the neurobiological data. Several studies have reported that the three core components of a deep learning framework for the brain are objective functions, learning rules, and architectures.
The objective functions describe the goal of the learning system. The learning rules describe how the parameters of a model are updated. These rules are typically used to improve the objective functions. They are associated with supervised learning as well as unsupervised learning. Architectures describe how the units of ANN are organized and their functions.
The majority of AI researchers focus on these three core components instead of designing specific computations. This is because it appears to be the most tractable way to solve real-world problems. Although many neuroscientists have emphasized the importance of learning rules and architecture, identifying the objective function has been less common. It has been suggested that normative explanations based on the three components could bring us one step closer to the form of "understanding" that many scientists seek.
Machine learning + neuroscience = biologically feasible computing | Benjamin Migliori | TEDxSanDiego
Common Concerns
Computer science and cognitive science tackle some big questions, one of which is precisely how learning occurs. Typically, neural networks work on the principle of supervised learning, which could be different from how learning takes place in real life. For example, a baby sees around a billion images in the first two years of life, and only a handful of these images are labeled. It could be difficult for a neural network to replicate this by seeing images from, say, ImageNet, where most images are categorized and annotated.
A second question is whether some aspects of intelligence are 'pre-installed' by evolution. As an example, we seem to be able to recognize a face as a face, and babies can do this from birth. Could our genes be encoding a mechanism to learn certain tasks quickly? The answer to that question could help researchers work out a way to assist machines in learning.
Sources
- What Is Deep Learning? 3 things you need to know. (2022) [Online] Available at: https://uk.mathworks.com/discovery/deep-learning.html
- Introducing Deep Learning with MATLAB. (2022) [Online] Available at: https://uk.mathworks.com/campaigns/offers/deep-learning-with-matlab.html
- Savage, N. (2019) How AI and neuroscience drive each other forwards. Nature. 571. pp. S15-S17. doi: https://doi.org/10.1038/d41586-019-02212-4
- Richards, B. A.et al. (2019) A deep learning framework for neuroscience. Nature neuroscience. 22(11). pp. 1761–1770. https://doi.org/10.1038/s41593-019-0520-2
- Vu, M. T. et al. (2018) A Shared Vision for Machine Learning in Neuroscience. The Journal of neuroscience : the official journal of the Society for Neuroscience. 38(7). pp. 1601–1607. https://doi.org/10.1523/JNEUROSCI.0508-17.2018
Further Reading