Fluorescence microscopy is a technique that uses fluorescent light to examine complex tissues at a level of detail that could not be achieved with conventional microscopes.
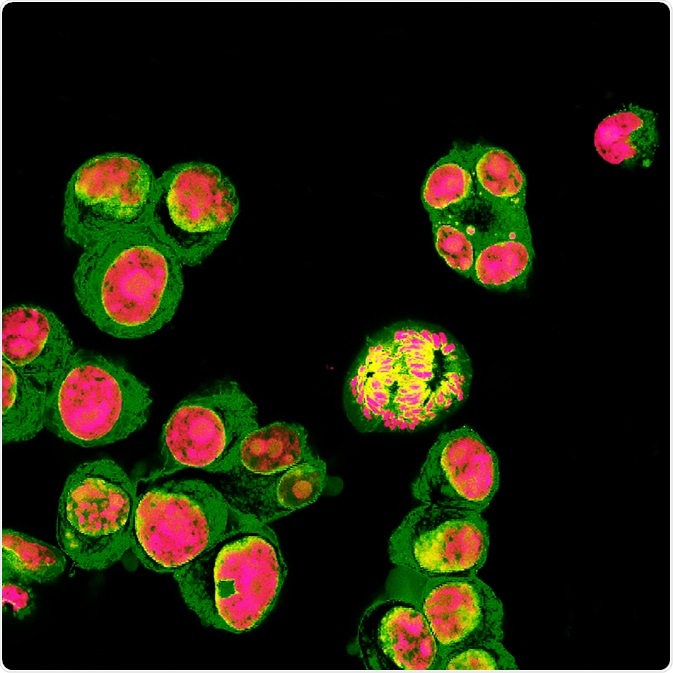
Image Credit: Vshivkova/Shutterstock.com
Using fluorescence microscopy, researchers can acquire high-sensitivity, high-resolution images of cellular structures and molecular species, for example, in samples that have been labeled with fluorophores.
On absorbing high-intensity light, the samples then emit a fluorescent light and scientists can magnify images of the resulting fluorescing material.
Deep learning is a branch of artificial intelligence that uses algorithms to model large data sets in a way that enables automated learning that resembles human brain function.
Deep learning is increasingly capturing the interest of researchers in the field of fluorescence microscopy for its current and potential applications in super-resolution and 3D image construction.
Turning low-resolution images into super-resolved ones
A recent study by Ozcan et. al. (2019) described how a deep learning-based approach provided super-resolution across a range of fluorescent microscopy modalities.
The method, which does not require numerical models of the imaging, is based entirely on a machine learning system called the generative adversarial network (GAN). The system is made up of algorithms that use two neural networks (one degenerative, one discriminative) that statistically learn and compete to provide higher-level predictions.
The team used GAN to change low-resolution input images from optical systems that had been diffraction-limited into super-resolution images.
The team demonstrated that the wide-field images acquired when objectives with low-numerical aperture are used could be super-resolved to match the resolution achieved when objectives with a high numerical aperture are used.
The team also showed that this super-resolution could be achieved across other modalities. They also showed that GAN can be used to change confocal microscopy images into the super-resolved fluorescent images that can be obtained using a stimulated emission depletion (STED) microscope.
Furthermore, the team demonstrated that images of cellular structures that can be obtained with total internal reflection fluorescence (TIRF) microscopy could also be super-resolved by GAN to match the level obtained with a TIRF-based structured illumination microscope.
In addition, the researchers say the GAN rapidly provides these super-resolved images, without requiring any search for iterations of parameters and could even work when it was applied to sample types that it had not been trained to super-resolve.
Refocusing 2D images into 3D ones
Another study by Ozcan et. al. (2019) showed that another deep learning neural network called Deep-Z learned to refocus 2D fluorescence microscopy images into stacks of 3D image slices of sample surfaces that enabled activity to be observed within the sample.
The team reports that the 3D images obtained with Deep-Z enabled them to observe neuronal activity within the Caenorhabditis elegans worm.
The 3D images were obtained using a sequence of fluorescent microscope images that were captured at a single focal plane, enhancing the field depth by 20 times, without requiring axial scanning, any extra hardware or having to compromise resolution or acquisition speed.
The method could also be used to connect other imaging modalities. The method 3D-focused a wide-field fluorescence microscopy image to achieve the images that can be obtained at various sample planes with confocal microscopy.
Another research group involving Tahir et. al (2019) applied an end-to-end Protein Localization Convolutional Neural Network (PLCNN) to accurately and reliably image the distribution of proteins in cells.
The researchers say that although many advances have been made in the field of fluorescent microscopy over recent decades, the resulting large number of images has presented challenges in terms of processing and analyzing.
Furthermore, the subjective examination of the images may not accurately distinguish the proteins, which may look different despite being from the same class or look the same despite belonging to different classes.
Although such challenges can be overcome using conventional machine learning, the preprocessing and extraction approaches involved before classification can be unreliable. This has meant researchers have increasingly relied on automated computational techniques over the last 20 years, which has improved the accuracy of protein subcellular localization based on fluorescent microscopy.
Tahir et. al. (2019) have applied the PLCNN deep learning approach to five publicly available subcellular localization datasets, namely the HeLa dataset, the CHO dataset, two LOCATE datasets and the Yeast dataset.
The researchers say that PLCNN consistently outperformed current state-of-the-art machine learning and deep learning algorithms across a wide range of protein localization datasets.
Analysis of the network showed that it captures protein features of interest, without capturing irrelevant details. The team adds that the generalizability of the approach is demonstrated by the consistent performance across all datasets used.
Sources:
- Belthangady C and Royer L. Applications, promises, and pitfalls of deep learning for fluorescence image reconstruction. Nature Methods 2019;16, p215–1225 https://doi.org/10.1038/s41592-019-0458-z
- Ozcan A, et al. Deep learning enables cross-modality super-resolution in fluorescence microscopy. Nature Methods 2019;16, 103–110 (2019). https://doi.org/10.1038/s41592-018-0239-0
- Ozcan A, et al. Three-dimensional virtual refocusing of fluorescence microscopy images using deep learning. Nature Methods 2019;16, p 323–1331 https://doi.org/10.1038/s41592-019-0622-5
- Tahir M et al. Deep localization of protein structures in fluorescence microscopy images. Deep AI 2019. Available at: deepai.org/.../deep-localization-of-protein-structures-in-fluorescence-microscopy-images
Further Reading