Drug discovery and AI
The process of discovering new drugs is often long and complex and can be divided into four major steps: target selection and validation; compound screening and optimization; preclinical studied and clinical trials.
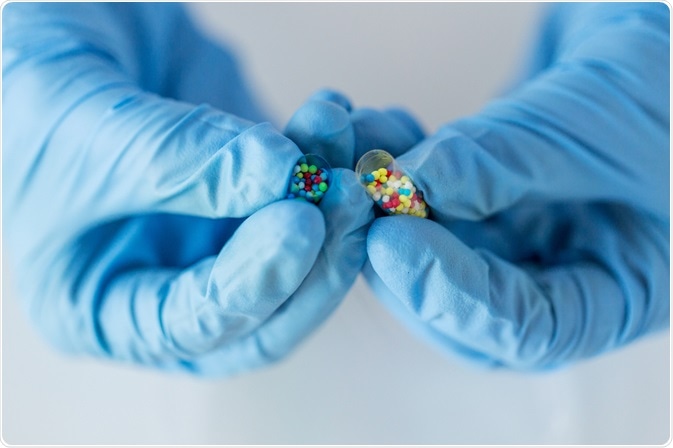
Image Credit: Syda Productions/Shutterstock.com
Initially, the target relating to a specific disease needs to be identified; this is through cellular and genetic target validation; proteomic analysis, and bioinformatic predictions.
Following this is hit identification where compounds are identified from a series of molecular libraries through combinatorial chemistry, high- throughput screening, and virtual screening. For newly synthesized drug candidates, they are interrogated for their structure-activity and also through in silico analysis to improve their functional properties.
Afterward, in vivo studies are carried out to determine pharmacokinetic properties and assess for toxicity. Once the drug candidate has passed these stages it is administered to patients in a series of clinical trials to interrogate the safety and efficacy of the drug. This process is estimated to take on average 12 years and cost approximately $2.6 billion.
This, therefore, presents a need to decrease the costs and speed up the testing of drug candidates. Therefore AI-based methods are increasingly being used in stages of the process to improve both the time and cost-efficiency.
These include cell classification, computer-aided organic synthesis, designing new molecules, and developing assays to predict 3D structures of target proteins. Whilst these processes can be long and often tedious, with the use of AI these processes can be automated and optimized to speed up the drug discovery process.
Finding the hit or lead
The implementation of AI in the discovery of a small drug-like molecule concerns the utilization of chemical space. The chemical space provides the stage for identifying novel and high-quality molecules as it is possible to enumerate the organic compounds.
Further to this, using predictive model software can enable the identification of target-specific virtual molecules and their subsequent association with their respective target while also optimizing the safety and efficacy attributes.
The use of AI systems can also have the additional benefit of reducing the attrition rates and expenditure associated with research and development by decreasing the number of compounds tested in vitro and in vivo.
Through using in silico techniques, it allows for the profiling via utilization of virtual ligands or a structure-based design used in combination with available data on small molecule modulator probes or their structural biology.
Thus, from this in addition to phenotypic data or disease, biology or molecules network-based algorithms can be applied to increase the success rates in development. Whilst the AI techniques that are in development must be validated before applying them to the drug development process.
AI in drug repurposing
With AI, the drug repurposing process has become increasingly more attractive. The idea being applying an existing therapeutic to a new disease is advantageous as the drug in question is ‘pre-approved’ to go directly to phase II trials for a different indication without having to pass through phase I clinical trials and toxicology testing. In silico methods can predict pharmacological properties of a drug and drug repurposing using transcriptomic data.
These methods used often rely on deep neural networks (DNNs) which is essentially a highly adaptive multi-layer system comprising of artificial connected neurons that can perform data transformation. Previous work by Alliper et al. demonstrated DNNs could classify complex drug action mechanisms on the pathway level.
Therefore, drugs could be classified into therapeutic categories according to their functional class, efficiency, therapeutic use, and toxicity profiles. Furthermore, the advances in precision medicine have resulted in next-generation AI that offers the ability to design drug molecules from generative adversarial networks (GANs).
GANs are a remarkable piece of technology through their ability to produce a photo-realistic image from text descriptions. Thus, this offers an astounding ability beyond just purely analyzing data, such as imagining or creating new data modeled on real data.
Another common technique used in silico data generation is reinforcement learning. The advantage of this is that it is less dependent on learning from datasets, therefore the networks can recognize certain strategies in drug molecule design.
Zhavoronknov et al. were able to generate algorithms that can reconstruct missing features from half-full datasets and therefore interpret the differences between normal and disease profiles within complex data.
An interesting element to consider from this is the algorithm is trained to recognize cardiotoxic and non-cardiotoxic drugs based on gene expression profiles from cells incubated with different drugs.
Conclusions and future perspectives
At present, pharmaceutical companies face challenges in their drug development because of an increased cost and reduced efficiency. Multiple AI tools have been developed to make these processes more cost-efficient.
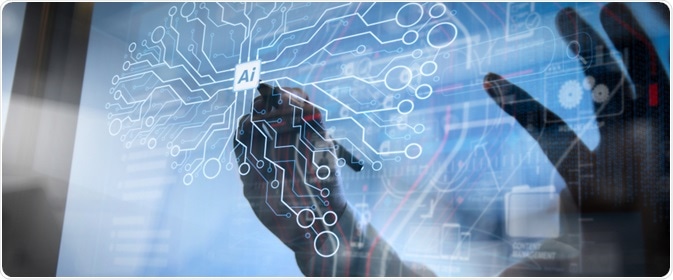
Image Credit: everything possible/Shutterstock.com
However, yet, there have not been any drugs developed utilizing AI approaches. Whilst it is still a somewhat novel and exciting area, it may still take time to see these advances as an individual should know how to develop and interrogate these algorithms.
In spite of the benefit of speeding up the drug discovery process, real lab experiments still need to be conducted to generate initial datasets to work from as a base point.
Sources
- Reymond, J.-L. et al. (2010) Chemical space as a source for new drugs. Med. Chem. Commun. 1, 30–38
- Okafo, G. et al. (2018) Adapting drug discovery to artificial intelligence. Drug Target Rev. 2018, 50–52
- Corsello, S.M. et al. (2017) The Drug Repurposing Hub: a next-generation drug library and information resource. Nat. Med. 23, 405–408
- Aliper, A. et al. (2016) Deep learning applications for predicting pharmacological properties of drugs and drug repurposing using transcriptomic data. Mol. Pharm. 13, 2524–2530
- Ozerov, I.V. et al. (2016) In silico pathway activation network decomposition analysis (iPANDA) as a method for biomarker development. Nat. Commun. 7, 13427
Further Reading