Researchers in the United States have developed a point scanning approach that increases the spatiotemporal resolution of imaging systems to unprecedented levels.
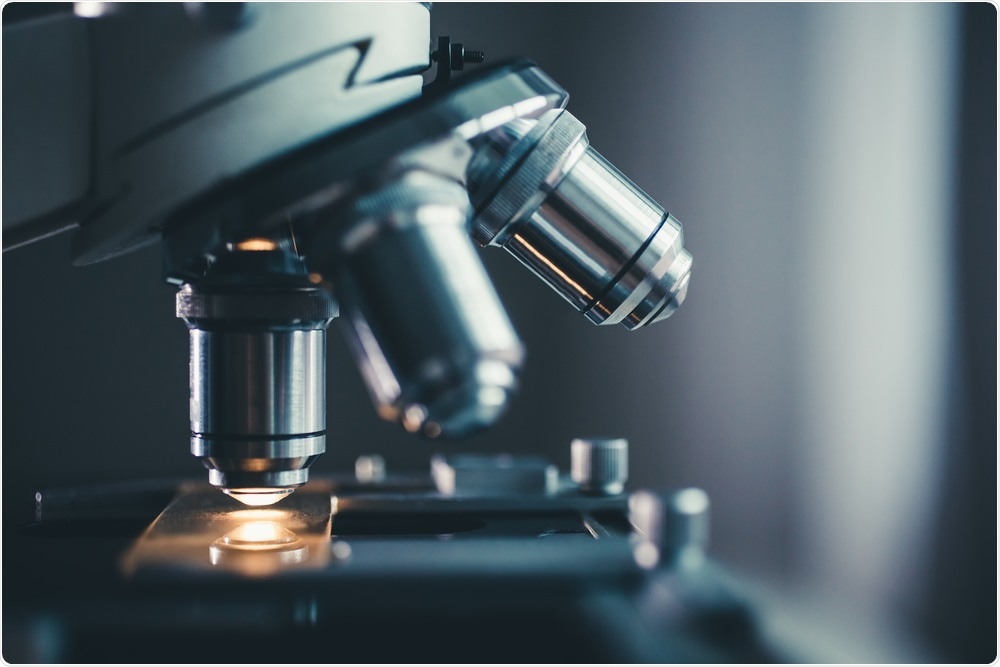
Image Credit: Konstantin Kolosov/Shutterstock.com
The team trained a deep-learning-based image restoration technique to super-resolved low-resolution images to high-resolution ones.
Microcopy is an essential tool for analyzing the organization of biological systems, but for all imaging modalities, optimizing the resolution, speed, signal-to-noise ratio and sample preservation poses challenges because it is difficult to optimize one parameter, without compromising another one.
This is a particular problem with point scanning systems such as scanning electron microscopy (SEM) or laser scanning confocal microscopy, which are probably the most used tools in biomedical imaging laboratories owing to their versatility and ease-of-operation.
Optimizing point scanning systems is difficult because they are restricted by an inverse relationship between imaging speed and pixel resolution.
3D electron microscopy techniques
3D electron microscopy (EM) is a powerful tool used to establish the volumetric ultrastructure of tissues. As well as serial section EM (ssEM) and focused ion beam SEM (FIB-SEM), another widely used tool for 3D electron microscopy imaging is serial block-face SEM (SBFSEM), in which an ultramicrotome cuts thin surface slices following SEM.
SBFSEM is a relatively high throughput method, but it is limited by higher electron doses that compromise image reliability, long imaging times and large file sizes. The datasets are often acquired using sub-Nyquist sampling, which prevents the reliable detection of smaller cell structures.
Although undersampled 3DEM datasets can be used, the ability to mine certain regions for higher resolution data on ultrastructure is desirable.
Introducing the deep learning model
Now, Linjing Fang and colleagues have provided a solution by using a technique they have called point-scanning super-resolution (PSSR) imaging, which uses a deep learning model to super-resolved undersampled images.
Frustrated by our inability to perform SBFSEM imaging with the desired 2 nm resolution and SNR necessary to reliably detect presynaptic vesicles, we decided to test whether a deep convolutional neural net model (PSSR) trained on 2 nm high resolution (HR) images could “super-resolve” 8 nm low resolution (LR) images to 2 nm resolution,”
To train the model, the team needed multiple high- and low-resolution pairs of images. Rather than manually acquiring these, they decided to produce semi-synthetic training information by computationally “decrappifying” oversampled high signal-to-noise ratio ground truth images to generate low-resolution versions that could be used to train the PSSR model to restore undersampled images.
How did PSSR perform?
The team reports that PSSR was very effective at restoring low-resolution images that had been acquired using a range of optics, samples, and detectors in various different labs.
“Our PSSR model is not restricted to data acquired in the exact same fashion or modality as our training set,” writes the team.
Next, the researchers tested whether PSSR could restore 8nm SBFSEM datasets to high-quality 2nm datasets.
Remarkably, the team found that not only did PSSR achieve this, but it also did so for a range of samples, including fly, rat and mouse samples and using images acquired from four microscopes from other laboratories.
In addition to the researchers’ SBFSEM and SEM imaging systems, PSSR restored images acquired using data from a field emission SEM system and a FIB-SEM system.
“PSSR can be used for 25x super-sampling with useful results, increasing the lateral resolution and speed of FIB-SEM imaging by a factor of at least 25x,” writes the team.
For fluorescence microscopy, the researchers found that combining undersampled confocal images with a PSSR model enhanced spatial resolution and signal-to-noise ratio at a laser dose that was around 100 times lower and a frame rate that was 16 times greater, compared with using high-resolution images.
Fang and colleagues say the study demonstrates that deep learning-based restoration of undersampled images enables more rapid and lower dose imaging for both SEM and scanning confocal microscopy systems. This results in faster imaging, less sample damage and smaller image file sizes as a result of fewer pixels being acquired
The Point Scanning Super-Resolution (PSSR) approach provides a strategy for increasing the spatiotemporal resolution of point scanning imaging systems to previously unattainable levels due to limitations imposed by sample damage or imaging speed when imaging at full pixel resolution,”
Future directions
The researchers also point out that deep learning-enabled deconvolution approaches could, in the future, be able to produce a more generalized model for a specific imaging system rather than a certain type of sample.
They say the capabilities of deep convolutional neural networks in the processing of imaging data could provide new opportunities for redesigning imaging systems, which could reduce costs, compared with the use of conventional methods for extracting high-resolution imaging data. “Similarly, automated, real-time corrections to the images and real-time feedback to the imaging hardware are now easily within reach.” The team says: “This is an area of active investigation in our laboratory and others.”
Journal reference:
Fang L, et al. Deep Learning-Based Point-Scanning Super-Resolution Imaging. Biorxiv 2019. DOI: https://doi.org/10.1101/740548