Single-cell analysis is an effective biomedical technique used to identify unusual cell populations, monitor cell development and differentiation, comprehend disease causes, and design individualized therapies, but it generates massive amounts of data that can be challenging to handle.
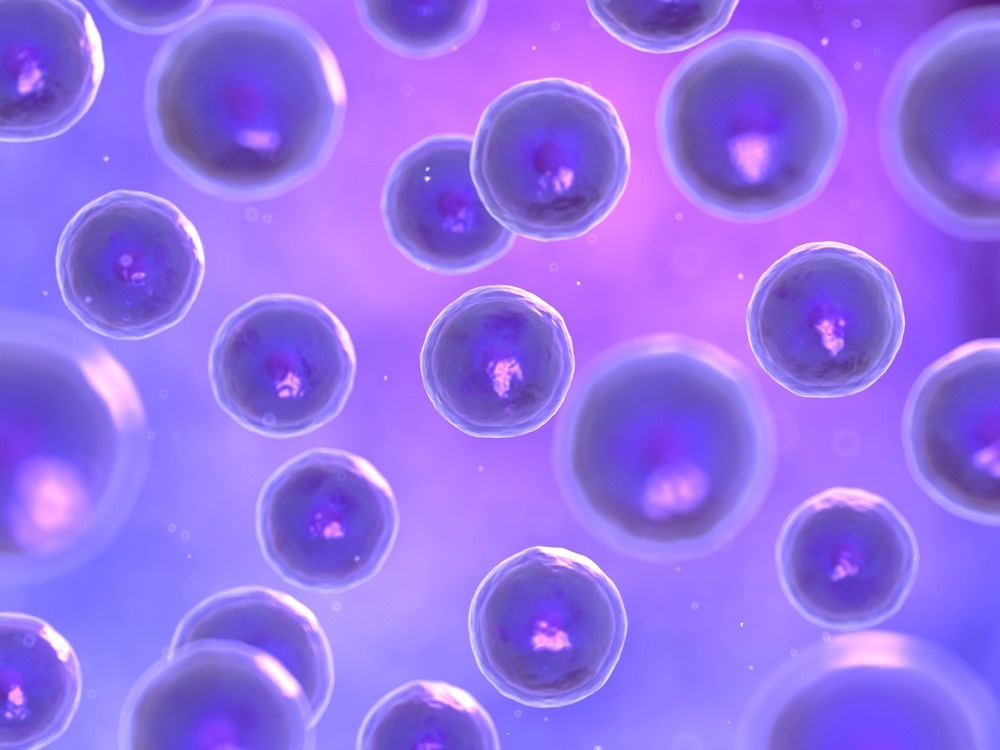
Image Credit: SciePro/Shutterstock.com
Demetri Psaltis of the Swiss Federal Institute of Technology Lausanne and Pietro Ferraro of the Institute of Applied Sciences and Intelligent Systems directed an international team of researchers who illustrated an effective encoding strategy for single-cell tomograms that greatly simplifies the handling and storage of single-cell data while preserving fidelity.
Their findings were published in Intelligent Computing, a Science Partner Journal, on January 11th, 2023.
The authors’ novel method effectively manages and processes the massive datasets produced by tomographic phase microscopy, a popular tool for single-cell research. This approach can provide 3D images of living cells and tissues quickly and without causing damage to the sample, making it a useful tool for investigating cellular dynamics such as division, migration, and differentiation.
A fundamental issue in tomographic phase microscopy nowadays is successfully processing huge amounts of 3D data to accomplish a quick and precise cellular diagnosis. The new technique has a data compression ratio of 22.9. This suggests that big-data single-cell tomograms can be compressed while saving over 95% of the space while losing less than 1% of the information.
The strategy functions effectively on a variety of experimental data, including spherical and non-spherical cells. In fact, it can process any volumetric image quickly.
A lab-on-a-chip tomographic imaging flow cytometry system—a technology that uses lasers to assess numerous features of individual cells as they travel through a fluid-filled channel—will be available in the future. To do this, a microfluidic module capable of regulating the locations and rotation of flowing cells using advanced cell manipulation solutions like multicore fiber will be required.
From a computational standpoint, the results presented here pave the way for an efficient methodology for storing, manipulating, and processing volumetric pictures, particularly for lab-on-chip systems that require a minimal memory footprint. The findings presented here will pave the way for future advances in computational microscopy for single-cell phase-contrast tomography.
The novel method is based on the Zernike polynomial’s 3D extension. The 3D Zernike representation is a method of describing an object’s shape in three dimensions. It is based on a mathematical notion known as spherical harmonics and excels at characterizing objects with roughly spherical shapes. Because cells in nature are frequently shaped like spheres, the 3D Zernike representation can be a powerful tool for analyzing them.
The investigations showed that using 3D Zernike polynomials, the 3D refractive index distribution of a cell may be easily represented in one dimension. They rebuilt a tomographic cell phantom and compared its performance to the most commonly used volumetric image compression strategies. After that, the approach was validated using real-world single-cell tomography data.
Source:
Journal reference:
Memmolo, P., et al. (2023). Loss Minimized Data Reduction in Single-Cell Tomographic Phase Microscopy Using 3D Zernike Descriptors. Intelligent Computing. doi.org/10.34133/icomputing.0010.