In this interview, Professor James T. Kadonaga speaks to AZoLifeSciences about his latest research into gene activation, and how artificial intelligence can help to aid its discovery.
What provoked your research into gene activation?
The proper regulation of gene activity is critically important for many biological phenomena. In our cells, we have tens of thousands of genes, and each of these genes performs a specific function. It is thus essential that our genes are correctly turned on and off as needed. The improper regulation of gene activity can lead to disease or death.
In my lab, we have been interested in a key early step in gene activation – the initiation of transcription. Transcription is the synthesis of messenger RNA (mRNA) copies of our genes, which are segments of DNA that encode a biological function. We study a special DNA element called the "core promoter", which directs the initiation of gene transcription.
For each gene, the core promoter is the site at which the synthesis of mRNA begins. Although all core promoters can initiate transcription, different genes have different versions of the core promoter. We sometimes call the core promoter the "gateway to transcription", as it is the starting point of gene transcription.
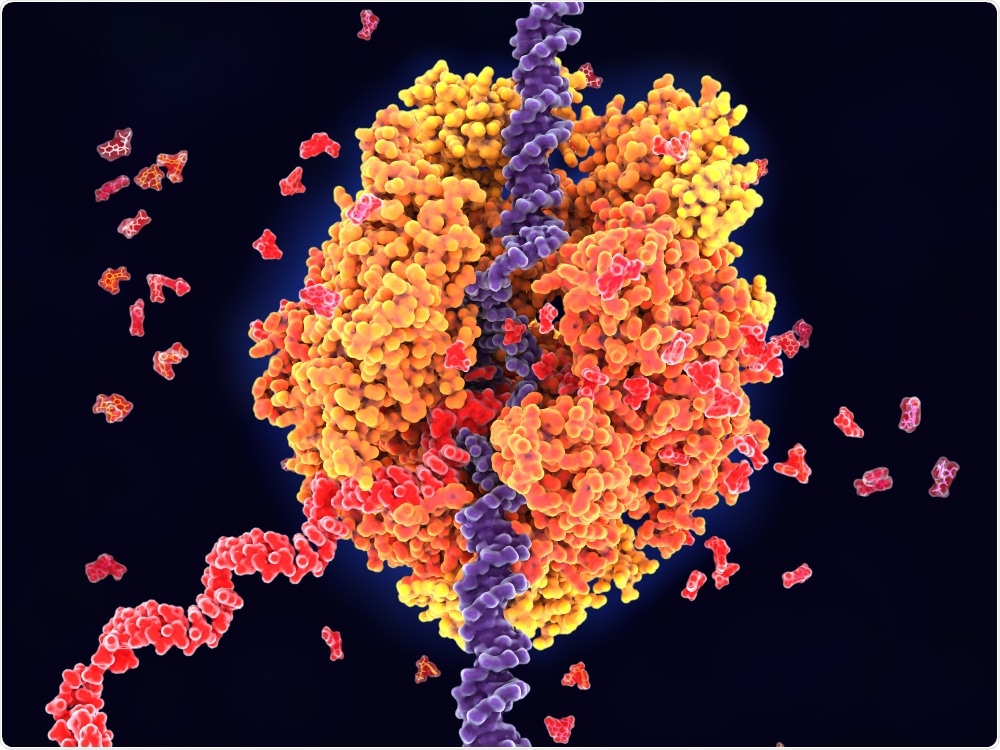
Image Credit: Juan Gaertner/Shutterstock.com
Can you describe what is meant by the “TATA box”?
A typical core promoter is composed of different DNA sequence modules, and the TATA box is a DNA sequence motif that is found in some core promoters. In this regard, it is useful to remember that DNA is a long molecular chain that has one of four different links (called nucleotides) termed A, C, G, and T.
There is important information that is encoded in the particular order in which the nucleotides are arranged in DNA. The DNA sequence modules in the core promoter, such as the TATA box, typically have specific DNA sequence signatures.
The DNA sequence signature of the TATA box often resembles "TATAAA". Hence, the "TATA box" is a DNA sequence segment or "box" that contains a TATAAA (shortened to "TATA")-like sequence signature.
Understanding how 75% of our genes are promoted is still a mystery to researchers. Why is this?
Although the TATA box is an important core promoter element, it is present in only about 25% of human genes. We, therefore, sought to identify other core promoter motifs that mediate the initiation of transcription in the absence of the TATA box ("TATA-less transcription") in humans. The achievement of this goal has been elusive.
We have been working on this problem for the past 23+ years! The key problem has been that the DNA sequence signatures (like "TATAAA" for the TATA box) of other core promoter motifs have been difficult to identify in humans.
To gain a broader understanding of TATA-less transcription in animals, we also studied core promoters in the fruit fly Drosophila melanogaster. In fruit flies, we found a DNA sequence motif that we termed the DPE (downstream core promoter element). The fruit fly DPE can direct transcription in the absence of the TATA box. It thus seemed reasonable that there would be a related DPE-like element in humans.
However, after over 20 years of work, we had found only about two or three active DPE-like elements in human genes. This led many researchers to conclude that the DPE does not exist in humans. Alternatively, it was possible that the human DPE might be too complex to describe in a simple DNA sequence signature. We investigated this hypothesis by using artificial intelligence.
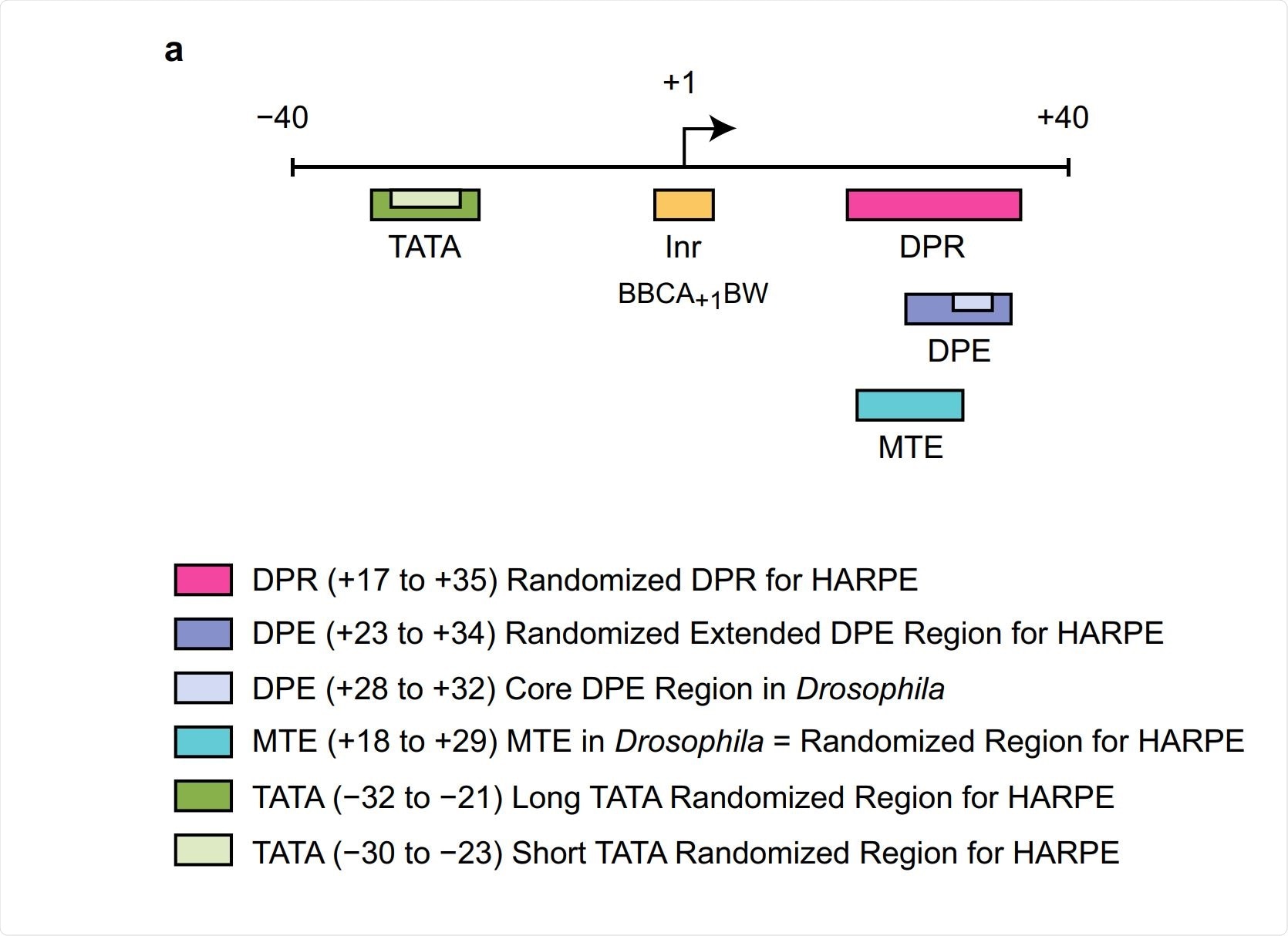
Image Credit: Identification of the human DPR core promoter element using machine learning
Your research involved using artificial intelligence to identify DNA activation codes. How did you carry out this research and what were your findings? How accurate is the machine learning model at predicting DPR activity in human DNA?
To venture beyond the use of DNA sequence signatures, we investigated the human core promoter by using machine learning, which is a form of artificial intelligence. In this analysis, we examined a slightly larger core promoter region than the DPE alone.
We called this larger region the DPR, for the downstream promoter region. (The DPR comprises the locations of the DPE and an adjacent motif that we termed the MTE, which we also found in fruit flies.) In this work, we employed a type of machine learning analysis termed support vector regression (SVR). [We tried different types of machine learning, and SVR analysis worked the best for us.]
We generated ~500,000 DNA sequence variants of the DPR and determined the transcription activity of each of the variants. We used 200,000 of those sequence variants to generate an SVR model that incorporates all of this information. Specifically, the SVR model was trained with the knowledge of 200,000 DPR sequence variants and the activities of each of the sequence variants.
With this information, the SVR model could predict the DPR activity of any new sequence that was not used in the training set of 200,000 sequences. We tested the ability of the SVR model to predict the DPR activities of thousands of independent DNA sequences (that is, sequences not used in the training of the SVR model), and the results were quite impressive.
For instance, the Spearman's rank correlation coefficient, rho, was typically around 0.90. These findings indicated that there is a distinct and identifiable DPR that activates transcription in humans.
Thus, the presence and strength (ability to activate transcription) of the human DPR can be accurately predicted by the SVR model. In contrast, the human DPR is too complex to be described by a DNA sequence signature. We found that the top 0.1% most active human DPR variants have an apparent DNA sequence signature that resembles the sequence signature of the fruit fly DPE, but the presence of this sequence signature alone is a poor predictor of DPR activity in humans.
Hence, the SVR model is much more effective than the DNA sequence signature for predicting the presence of an active DPR.

Image Credit: Kadonaga Lab, UC San Diego
What did you notice in your comparison between DPR and the TATA box?
There are similarities and differences between the DPR and the TATA box. Both the DPR and the TATA box can direct the initiation of gene transcription. In addition, the DPR and TATA box are of comparable estimated abundance (~25% to 33% of genes for the DPR; ~25% of genes for the TATA box). However, we also found a duality between the DPR and the TATA box. That is, genes with a DPR generally lack a TATA box and vice versa.
Therefore, many genes are primarily activated by the DPR, whereas other genes are primarily activated by the TATA box. This finding strongly suggests that DPR-dependent genes are regulated differently than TATA-dependent genes. This topic is an area of future investigation.
How will your research aid biotechnology and biomedical applications?
Many aspects of biotechnology and biomedical research involve turning genes on and off. Many human diseases are the consequence of the improper regulation of gene activity. It is therefore essential to know and to understand the DNA sequence elements that direct the activation of genes.
With this knowledge, we will have much greater success in achieving our goals in biotechnology and biomedical research.
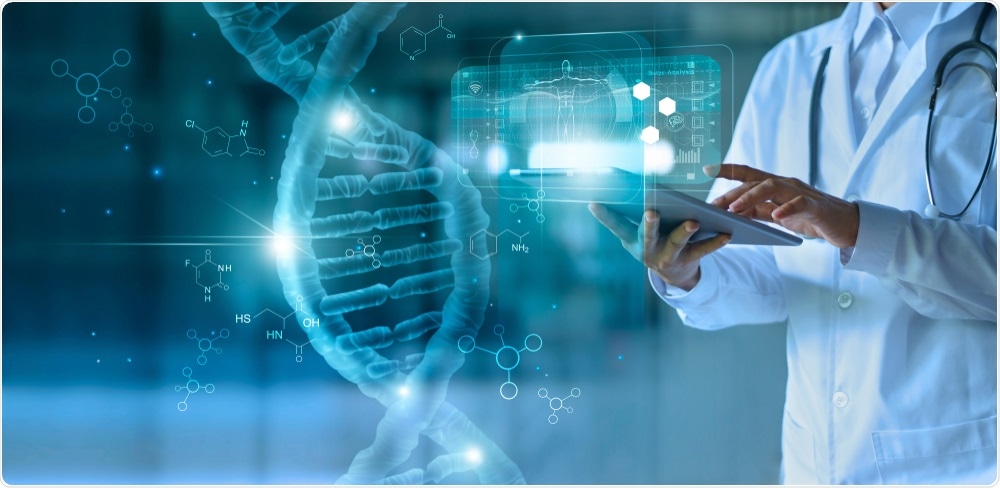
Image Credit: PopTika/Shutterstock.com
Do you believe that now you have identified the DPR, more DNA activation codes will be discovered using artificial intelligence?
Yes. Artificial intelligence can certainly be used to decipher the DNA activation codes of new functionally important DNA elements that do not have easily identifiable DNA sequence signatures. We are currently carrying out such experiments. It is also useful to note that artificial intelligence can be used to provide more accurate predictive models of known DNA sequence motifs.
For example, in our recent paper, we made an SVR model for the TATA box, and this SVR model was better at predicting the activities of TATA box sequence variants than standard sequence signature methods.
In your research, you used machine learning. How does this technology help aid in scientific discoveries? What are the benefits of using machine learning in research?
I am not an expert in machine learning; so, I will leave the complete answer to the experts. In the context of our research, the machine learning model for the DPR was clearly superior to the DNA sequence signature approach. It was the difference between success and failure.
In this regard, it is useful to note that DNA sequence signatures are usually based on the sequence characteristics of only the most active DNA sequence variants (for example, the top 1% or top 0.1% most active sequences).
In contrast, the machine learning models are based on the characteristics of all sequences – that is, strong, medium, and weak sequences. Thus, machine learning models incorporate much more information than DNA sequence signatures.
It is also important to mention that our machine models provide a quantitative prediction of DPR or TATA box activity. In other words, if you submit a DNA sequence query to the machine learning model, it will give you a number for its predicted activity.
In the old days, we would look at a potential TATA box sequence and say, "That looks like a good TATA box." Now, we would use the machine learning model for the TATA box and say, "That sequence has a TATA box score of 5."
In the analysis of DNA sequence elements, I believe that there will be a general trend from the use of DNA sequence signatures to machine learning models. More generally, we will only be limited by our own imaginations for other applications of machine learning in the biological and biomedical sciences.
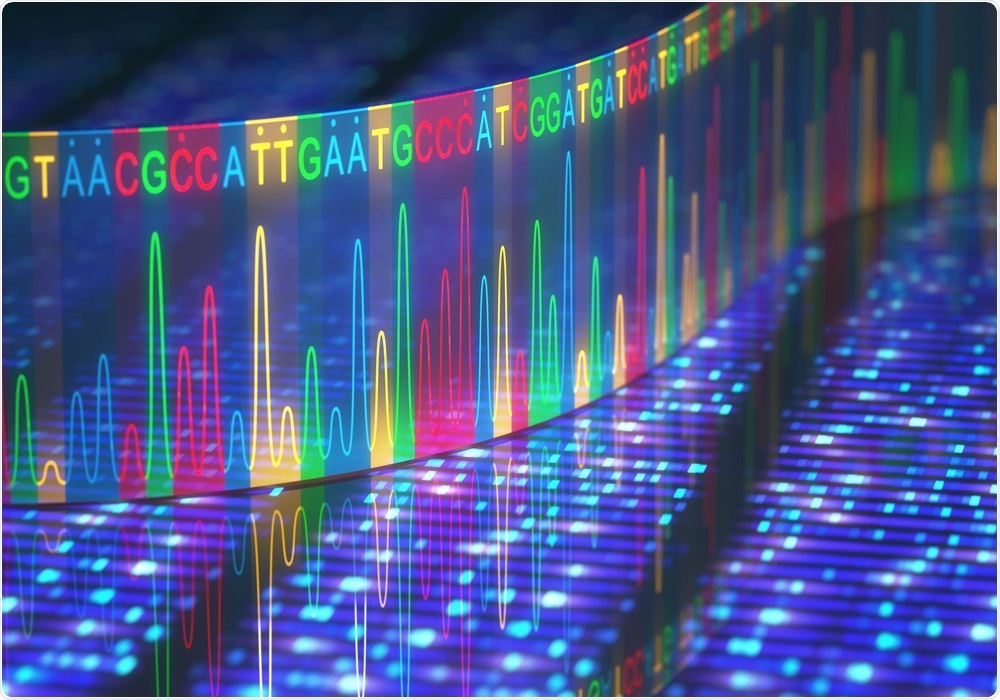
Image Credit: ktsdesign/Shutterstock.com
What are the next steps in your research into gene activation?
Some of our future goals include the following. First, we hope to gain a better understanding of the complete core promoter region. This work includes the use of machine learning to identify other core promoter elements that have yet to be discovered. We are also very interested in the protein factors that recognize the core promoter elements and activate transcription.
More broadly, we will seek to identify the interactions between specific core promoter elements, such as the DPR, and other regulatory elements, such as those called transcriptional enhancers. As the "gateway to transcription", the core promoter is strategically positioned at a key point in gene activation.
Where can readers find more information?
About Professor James Kadonaga
Jim Kadonaga was an undergraduate in chemistry at MIT, where he received both the Alpha Chi Sigma Prize and the American Institute of Chemists Certificate in 1980. He carried out his graduate studies (1980-84) with Jeremy R. Knowles in the Department of Chemistry at Harvard University, where he was a DuPont Fellow.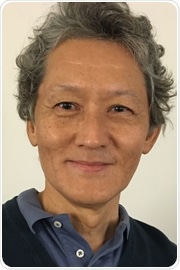
Jim was a postdoctoral associate (1984-88) with Robert Tjian at the University of California Berkeley as a Fellow of the Miller Institute for Basic Research in Science, American Cancer Society California Division, and Lucille P. Markey Charitable Trust. He joined the faculty of the University of California San Diego (UCSD) in 1988 and was one of 15 scientists to be named as a Presidential Faculty Fellow by President George H.W. Bush in 1992.
In 1994, he was elected to Fellow of the American Association for the Advancement of Science, and in 1995, he was elected to Fellow of the American Academy of Microbiology. He served as the Chair of the Section of Molecular Biology from 2003 to 2007.
In 2012, he received the UCSD Chancellor's Associates Award for Excellence in Research in Science and Engineering. In 2017, he was elected to the American Academy of Arts & Sciences. In 2020, he was elected to Revelle College Faculty Fellow at UCSD. Jim is presently the Amylin Endowed Chair and a Distinguished Professor in the Section of Molecular Biology at UCSD.